DisenSemi: Semi-Supervised Graph Classification Via Disentangled Representation Learning
IEEE TRANSACTIONS ON NEURAL NETWORKS AND LEARNING SYSTEMS(2024)
摘要
Graph classification is a critical task in numerous multimedia applications, where graphs are employed to represent diverse types of multimedia data, including images, videos, and social networks. Nevertheless, in the real world, labeled graph data are always limited or scarce. To address this issue, we focus on the semi-supervised graph classification task, which involves both supervised and unsupervised models learning from labeled and unlabeled data. In contrast to recent approaches that transfer the entire knowledge from the unsupervised model to the supervised one, we argue that an effective transfer should only retain the relevant semantics that align well with the supervised task. We introduce a novel framework termed in this article, which learns disentangled representation for semi-supervised graph classification. Specifically, a disentangled graph encoder is proposed to generate factorwise graph representations for both supervised and unsupervised models. Then, we train two models via supervised objective and mutual information (MI)-based constraints, respectively. To ensure the meaningful transfer of knowledge from the unsupervised encoder to the supervised one, we further define an MI-based disentangled consistency regularization between two models and identify the corresponding rationale that aligns well with the current graph classification task. Experiments conducted on various publicly available datasets demonstrate the effectiveness of our .
更多查看译文
关键词
Disentangled representation learning,graph neural networks (GNNs),semi-supervised graph classification,graph neural networks (GNNs),semi-supervised graph classification
AI 理解论文
溯源树
样例
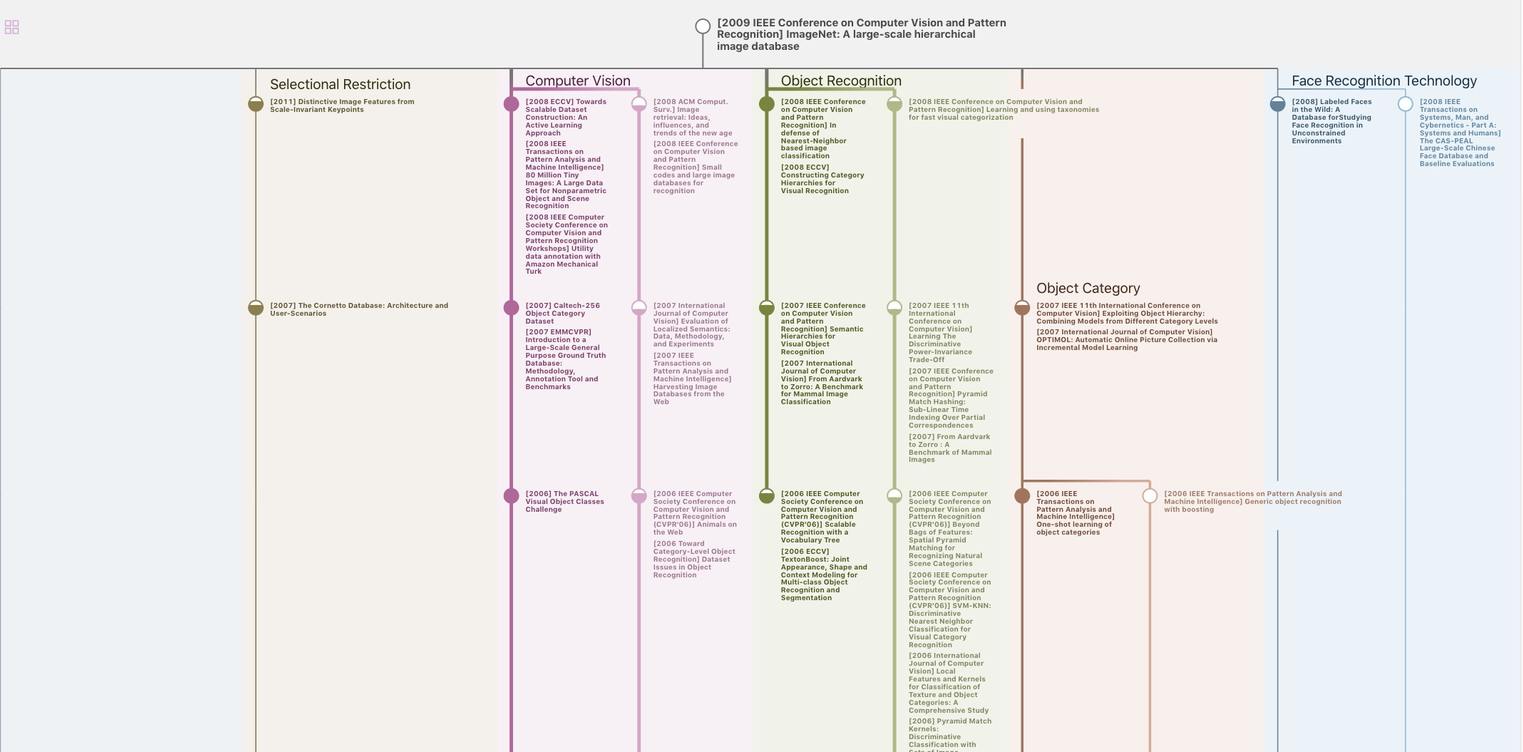
生成溯源树,研究论文发展脉络
Chat Paper
正在生成论文摘要