Momentary Depression-Severity Prediction in Acutely Depressed Patients Undergoing Sleep Deprivation Therapy: Speech-based Machine Learning (Preprint)
JMIR Mental Health(2024)
Abstract
Mobile devices for remote monitoring are inevitable tools to support treatment and patient care, especially in recurrent diseases such as Major Depressive Disorder. The aim of this study was to learn if machine learning (ML) models based on longitudinal speech data are helpful in predicting momentary depression severity. Data analyses were based on a dataset including 30 inpatients during an acute depressive episode receiving Sleep Deprivation Therapy in stationary care, an intervention inducing a rapid change in depressive symptomatology in a relatively short period of time. Using an ambulatory assessment approach, we captured speech samples and assessed concomitant depression severity via self-report questionnaire over the course of three weeks (before, during, and after therapy). We extracted 89 speech features from the speech samples using the eGeMAPS parameter set from openSMILE and the additional parameter speech rate. We aimed to understand if a multi-parameter ML approach would significantly improve the prediction compared to previous statistical analyses, and, in addition, which mechanism for splitting training and test data was most successful, especially focusing on the idea of personalized prediction. To do so, we trained and evaluated a set of >500 ML pipelines including random forest, linear regression, support vector regression, and eXtreme gradient boosting regression models and tested them on five different train-test split scenarios: a group 5-fold nested cross-validation on subject level, a leave-one-subject-out approach, a chronological split, an odd-even split, and a random split. In the 5-fold cross-validation, the leave-one-subject-out, and the chronological split approaches, none of the models were statistically different from random chance. The other two approaches produced significant results for at least one of the models tested, with similar performance. In total, the superior model was an XGBoost regression in the odd-even split approach (R² = 0.339, MAE = 0.38; both P<.001), indicating that 33.9% of the variance in depression severity could be predicted by the speech features. Overall, our analyses highlight that ML fails to predict depression scores of unseen patients, but prediction performance increased strongly compared to our previous analyses with multilevel models. We conclude that future personalized ML models might improve prediction performance even more, leading to better patient management and care.
MoreTranslated text
AI Read Science
Must-Reading Tree
Example
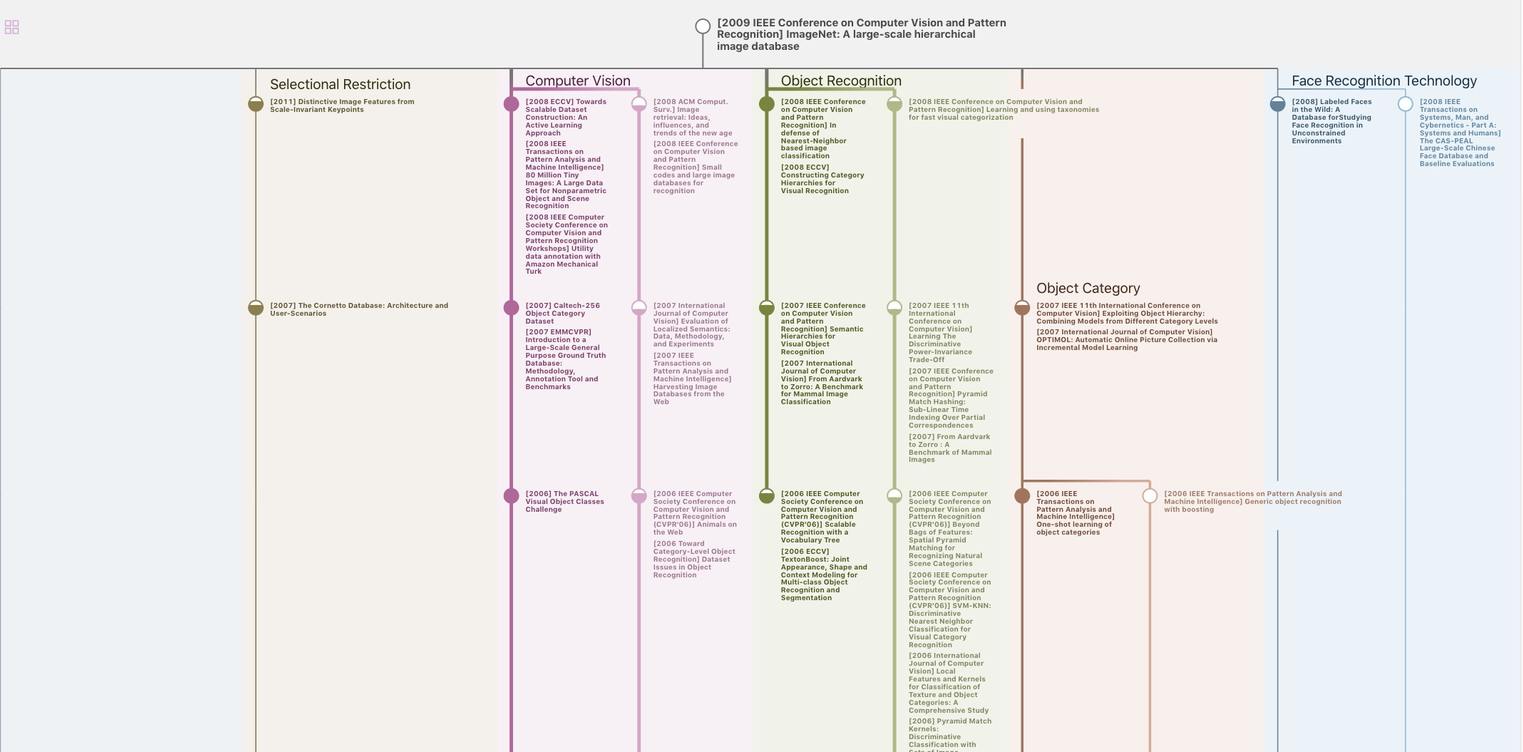
Generate MRT to find the research sequence of this paper
Chat Paper
Summary is being generated by the instructions you defined