DM-GAN: CNN Hybrid Vits for Training GANs under Limited Data
Pattern Recognition(2024)
摘要
Generative adversarial network (GAN) training demands substantial data and computational resources. This paper aims to explore an economical approach for generating novel images with limited image data, addressing the challenge of data scarcity. Our contributions involve resolving the few-shot image generation challenge through the development of an unsupervised hybrid generative adversarial network named DM-GAN. We introduce a lightweight hybrid module (DC-Vit) comprising convolution and visual transformation, merging local and global features to enhance image perception, expressiveness, and ensure stable image generation. Additionally, a multi-scale adaptive skip connection module is incorporated to effectively mitigate the feature loss problem arising from inter-layer jumps, thereby producing more complete and regular images. To enhance the texture learning process and improve the quality and realism of synthesized images, we integrate the gray conjugate matrix into the loss function. Empirical evaluations are conducted on small sample datasets at various resolutions, including publicly accessible collections of art paintings, real-life photographs, and proprietary artifact image datasets. The experimental results unequivocally demonstrate the qualitative and quantitative superiority of our model over existing methods, underscoring its efficacy and robustness.
更多查看译文
关键词
GAN,Few-shot,Vision transformer,Proprietary artifact image
AI 理解论文
溯源树
样例
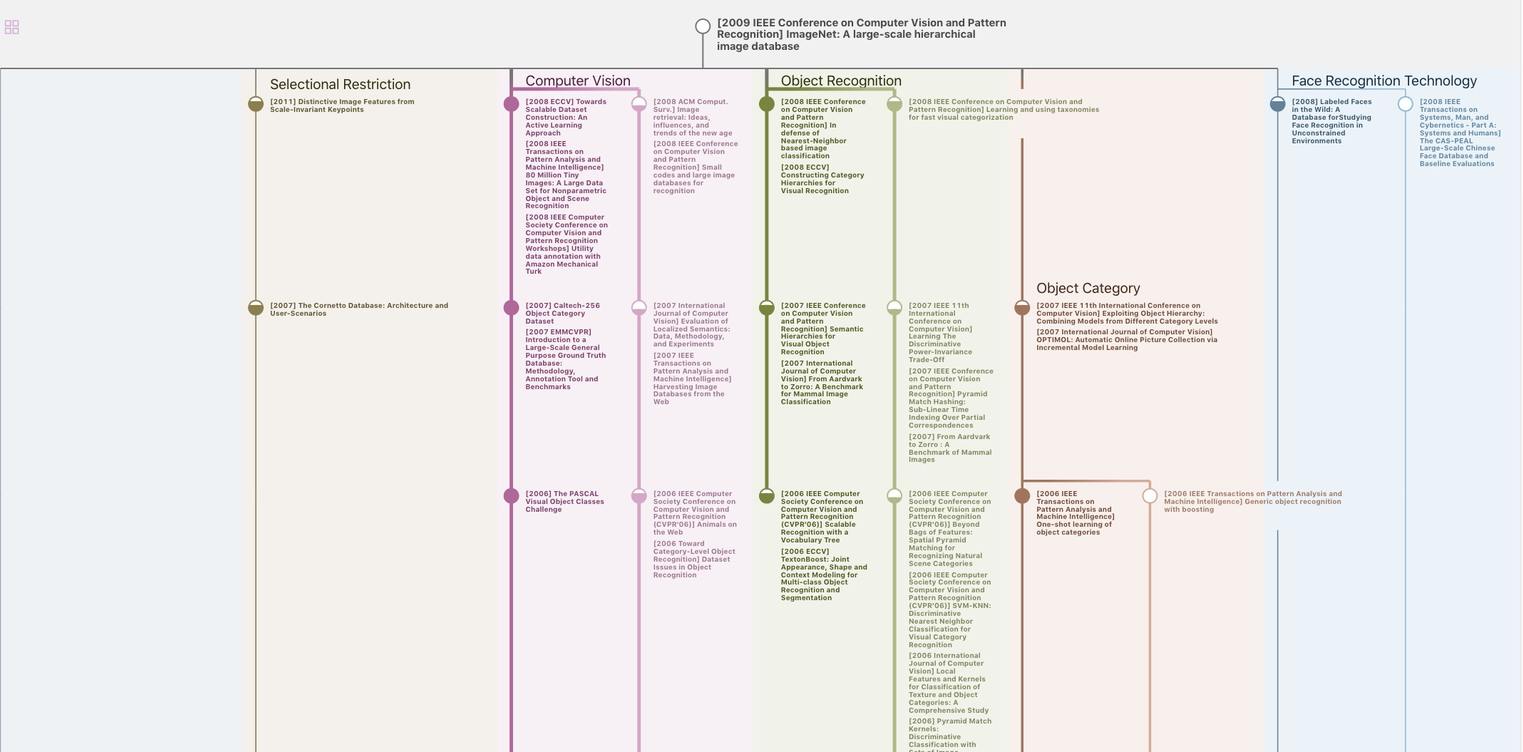
生成溯源树,研究论文发展脉络
Chat Paper
正在生成论文摘要