River Water Temperature Prediction Using a Hybrid Model Based on Variational Mode Decomposition (VMD) and Outlier Robust Extreme Learning Machine
Environmental Processes(2024)
摘要
We propose a new method for predicting daily river water temperature (Tw) using two input variables, namely: (i) air temperature (Ta); and (ii) river discharge (Q). The study was conducted using data collected at two stations operated by the United States Geological Survey (USGS), located at the Missouri River, USA, i.e., Hermann and St. Joseph stations. We propose the application and comparison between four Extreme Learning Machines (ELM) models, namely the standalone ELM, the Outlier Robust Extreme Learning Machine (ORELM), the Regularized Extreme Learning Machine (RELM), and the Weighted Regularized Extreme Learning Machine (WRELM). In addition, we use the Variational Mode Decomposition (VMD) as a signal decomposition algorithm for decomposing the Ta and Q into several sub-signals, i.e., the Intrinsic Mode Functions (IMFs), which were used as new input variables. The ELM, ORELM, RELM, and WRELM models were developed for modeling Tw and we compared their performances for three scenarios: (1) Ta as the input variable; (2) Q as the input variable; and (3) Q and Ta combined as the input variables. Models were developed and compared for three scenarios with VMD and without VMD (standard) for predicting Tw. The model performances were evaluated using the Nash–Sutcliffe Efficiency (NSE) and the Mean Absolute Error (MAE). The results show that the predictive accuracy of the hybrid ORELM-VMD, WRELM-VMD, RELM-VMD, and ELM-VMD was higher relative to other standalone models, i.e., ORELM, WRELM, RELM, and ELM. Furthermore, the ORELM1 combined with the VMD algorithm was found to be the best-performing model with the best NSE and MAE values, which ranged from 0.844 to 0.856, and 2.719 to 2.204 for the Hermann station and from 0.916 to 0.924, and 2.177 to 1.970, for the Joseph station, respectively. We can draw the following conclusions based on the results obtained in the present study. First, all models with and without VMD decomposition failed to accurately predict Tw using only Q as the input, showing performances significantly decreased compared to the models based on Ta. Second, combining Q with Ta does not help improve the predictive accuracy; on the contrary, all models have shown that their performances decreased. Finally, we argue that our research demonstrates a solid prediction approach to improve the prediction of river water temperature. • Water temperature (Tw) was predicted using only air temperature (Ta) and river discharge (Q). • A comparison was conducted between the extreme learning machine (ELM) and its three variants. • An analysis of the input variables was performed using Variational Mode Decomposition (VMD). • Based on the obtained results, it can be concluded that Hybrid ELM with VMD offers superior performance.
更多查看译文
关键词
Machine learning,Air temperature,Water temperature,River discharge,Signal decomposition,Extreme learning machine (ELM),ORELM,RELM,WRELM
AI 理解论文
溯源树
样例
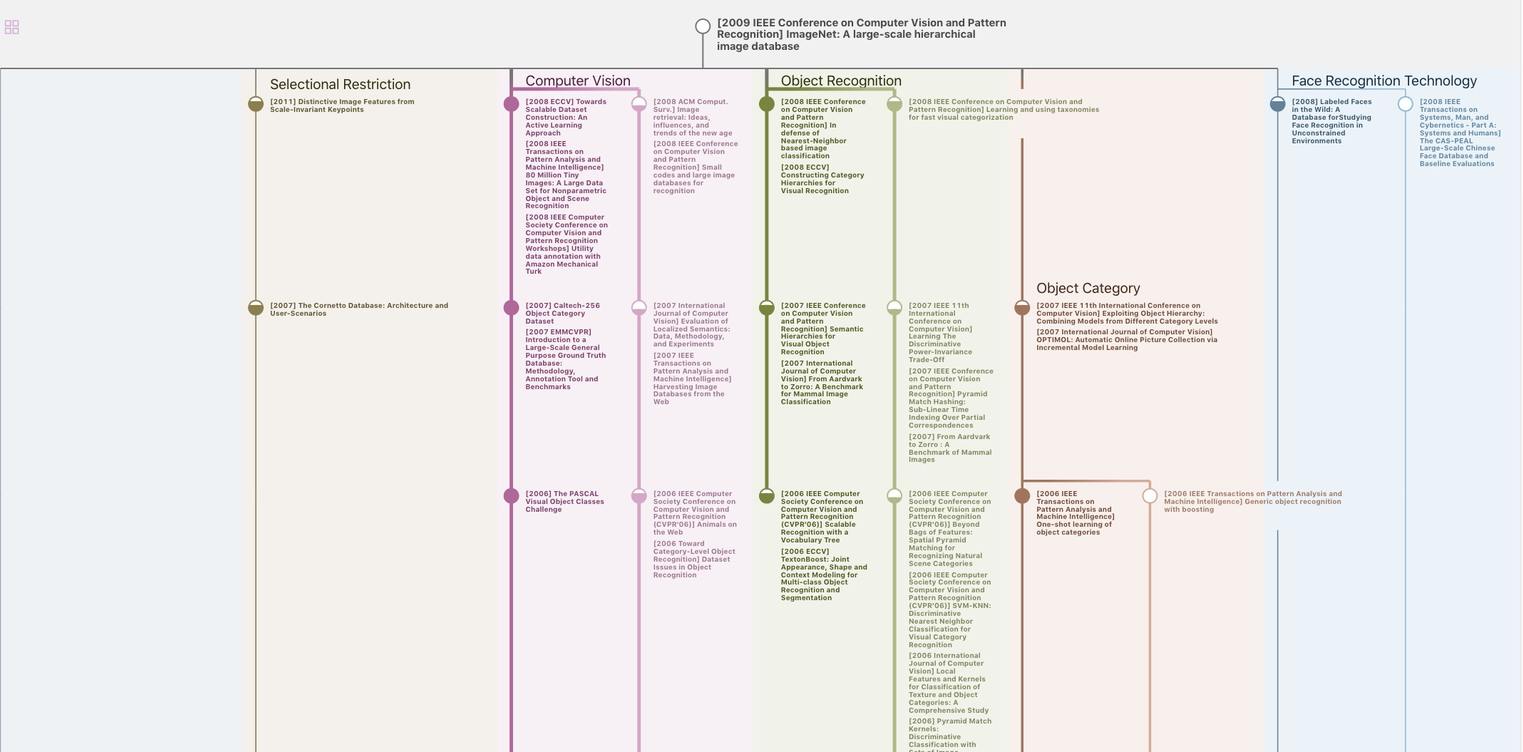
生成溯源树,研究论文发展脉络
Chat Paper
正在生成论文摘要