Residual Life Prediction of Rolling Bearing Based on LSTM Neural Networks
2024 IEEE 4th International Conference on Electronic Technology, Communication and Information (ICETCI)(2024)
Abstract
The prediction of remaining useful life can provide a basis for preventive maintenance decisions of mechanical equipment, which is of great significance for extending the life cycle of mechanical equipment, improving production efficiency, and reducing accidents. A new method for predicting the remaining useful life and health monitoring of bearings is proposed based on the characteristics of Long Short Term Memory (LSTM) neural networks. Firstly, this paper extracts the 34 dimensional characteristic parameters of rolling bearing full life vibration signal in different domains. We use time correlation, monotonicity, and separability to filter out the 17 dimensional characteristic parameters strongly associated with degradation information, forming a degradation feature dataset; Then, we construct an LSTM neural network model and train the LSTM neural network with a degradation feature dataset that includes normalized life labels. Finally, we conduct experiments to predict the remaining useful life (RUL) of rolling bearings under three working conditions. The LSTM neural network is trained by using a rolling bearing dataset for the whole life cycle of a working condition. The RUL of bearing is predicted under three different operating conditions. The results show that the predicted residual useful life curve is in good agreement with the actual curve. This method realizes the prediction of RUL of rolling bearings under different working conditions. The LSTM network model has high accuracy and generalization ability in predicting RUL of bearings. Comparison of prediction results with BP network, the effectiveness of LSTM network in remaining useful life and health inspection is further verified.
MoreTranslated text
Key words
Rolling bearings,RUL prediction,LSTM,feature parameter
AI Read Science
Must-Reading Tree
Example
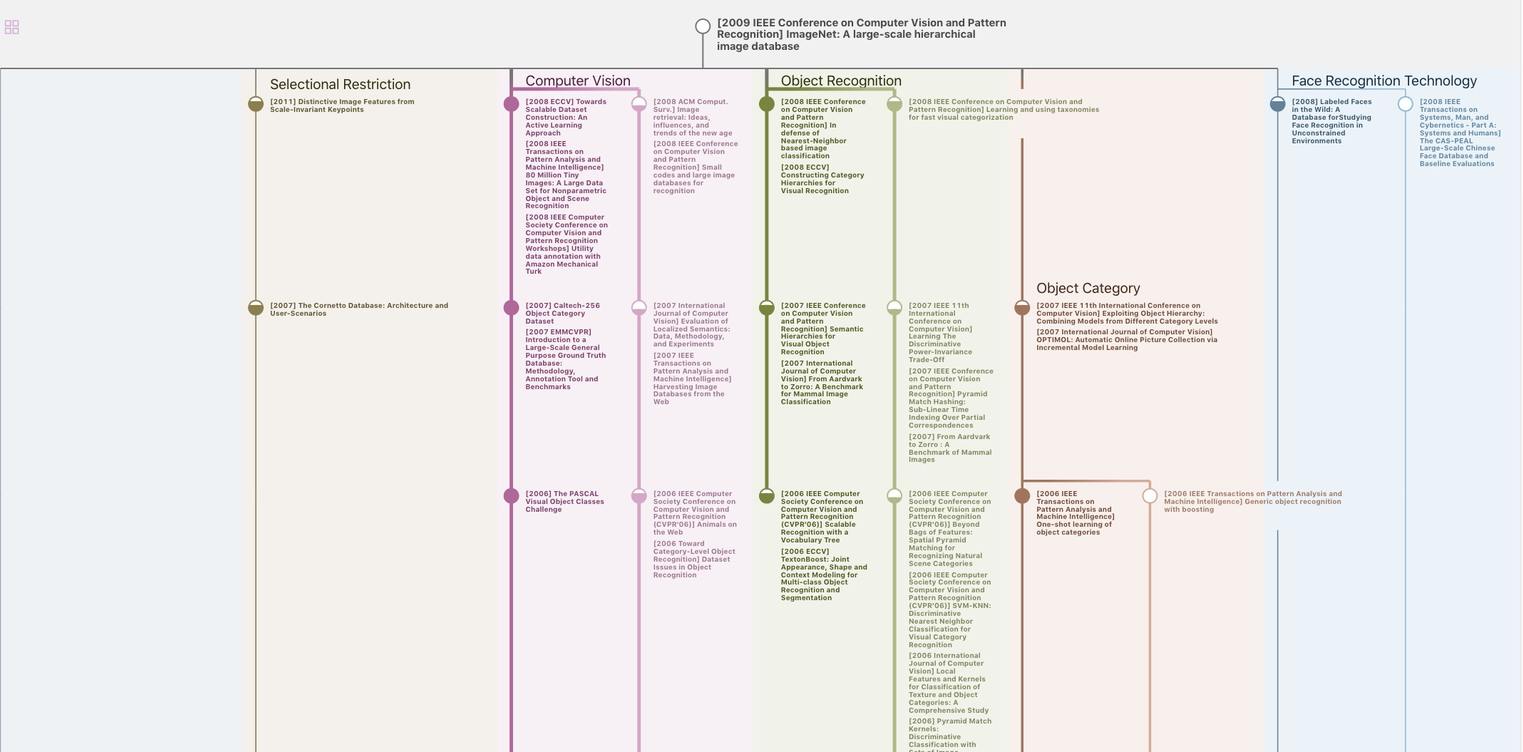
Generate MRT to find the research sequence of this paper
Chat Paper
Summary is being generated by the instructions you defined