Feature Selection of Acoustic Signals for Leak Detection in Water Pipelines
Tunnelling and Underground Space Technology(2024)
摘要
Water pipelines often have small cracks and leaks over time due to various degradation process. Acoustic emission technique is an outstanding method in leak detection for low cost and carrying out easily. Currently, the existing leak detection methods using acoustic signals consider only the contribution of individual features without considering the interactions between features in the feature selection process. To address this gap, this study introduces a generalized feature selection framework called Maximal Discernibility and Minimal Redundancy and Improved Sequential Floating Forward Selection (MDMR_ISFFS). In this study, time and frequency domain features are extracted from acoustic leakage signals observed in real water supply pipes. The effectiveness of the feature selection method is verified by different machine learning methods and interpretability methods. Moreover, the method proposed in this paper is also compared with other feature selection methods. The results demonstrate that four key features (i.e., Mean of frequency, Zero-crossing Rate, Peak frequency and Mean) are identified as the crucial features consistently regardless of the classifiers. All five classification models using MDMR_ISFFS can achieve high leak detection accuracies, ranging from approximately 94 % to 98 %. When compared to other feature selection methods (i.e., DFS_SFFS, KL distance and original feature set), the proposed MDMR_ISFFS can achieve the highest accuracy with a smaller number of features. Moreover, the study reveals significant interactions among the four key leakage features. In summary, this research provides valuable insights for selecting key leak features in actual pipeline leak detection.
更多查看译文
关键词
Acoustic signals,Leak detection,Feature selection,Feature interaction,Water pipelines
AI 理解论文
溯源树
样例
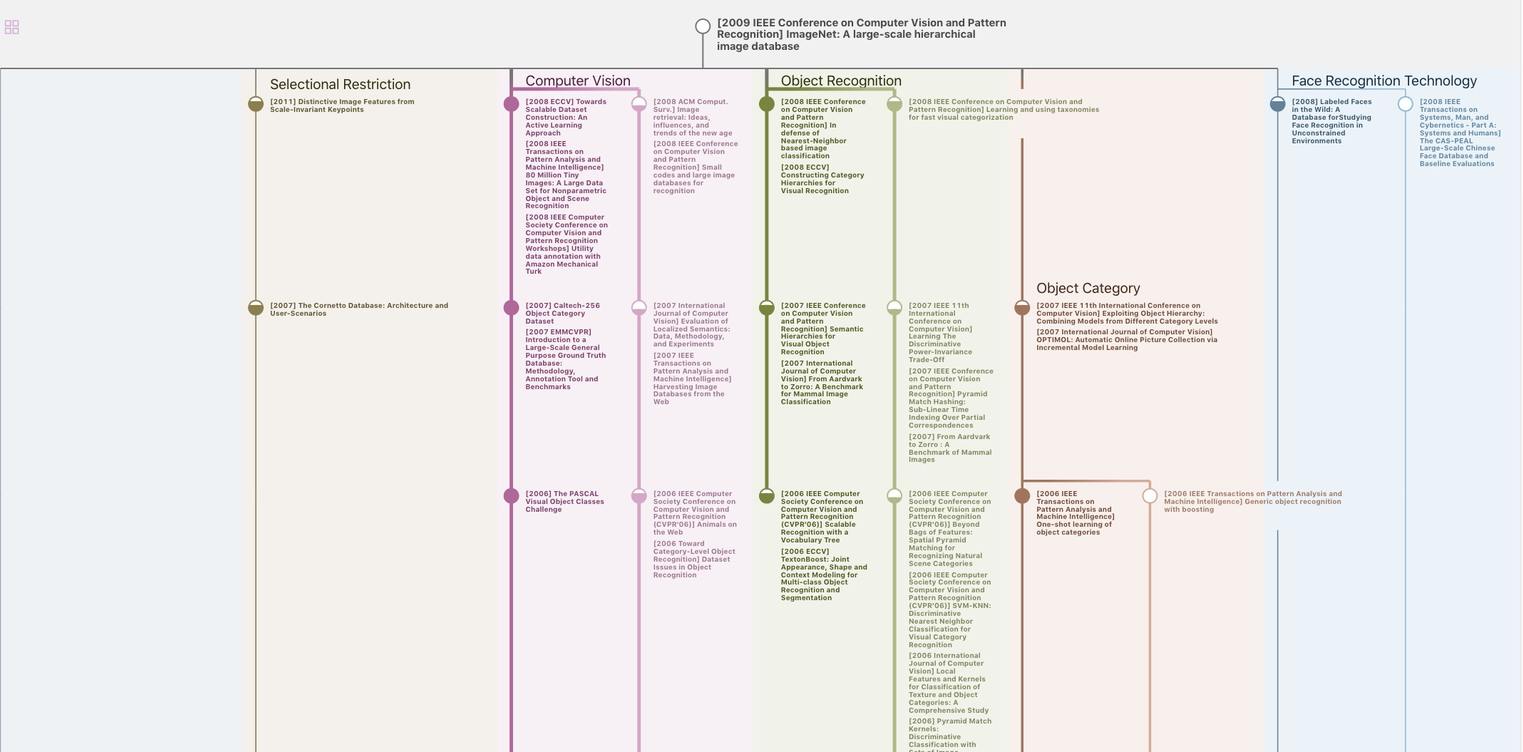
生成溯源树,研究论文发展脉络
Chat Paper
正在生成论文摘要