Numerical Framework for Predicting Fatigue Scatter in Additively Manufactured Parts
International Journal of Mechanical Sciences(2024)
摘要
The layer-based deposition mechanism during electron beam powder bed fusion (PBF-EB) inevitably induces significant surface irregularities, which often act as stress concentrators under cyclic fatigue load, resulting in early fatigue failure and scattered lifetime. Reliable design of additively manufactured metallic lattice structures necessitates a comprehensive understanding of the fatigue behaviour of micro-sized structural parts, typically obtained through expensive and time-consuming full-scale fatigue testing. This study proposed a deep learning-based framework to predict defect-induced fatigue scatter in micro-sized as-built titanium parts produced with PBF-EB technique. The surface morphology synthesized by generative adversarial networks (GANs) was incorporated with finite element modelling to investigate defect-induced stress concentrations. A fatigue damage model, based on continuum damage mechanics and theory of critical distances, was developed to predict fatigue lifetime. The synthesized morphology showed good agreement with experimental results in roughness evaluation. Using the proposed framework, the fatigue scatter was successfully reproduced, and a probabilistic lifetime design curve was developed accordingly to facilitate reliable and cost-effective fatigue design. The primary contributions of this study are the first-time application of a GAN architecture for synthesizing realistic morphology of micro-sized as-built PBF-EB Ti6Al4V parts with significant surface irregularities, and the investigation of defect-induced fatigue scatter using synthetic morphology and numerical methods. The results provide new insights into the effects of surface irregularity on stress concentration and scattering of lifetime in micro-sized as-built titanium parts.
更多查看译文
关键词
Fatigue lifetime prediction,Deep learning,Additive manufacturing,Finite element analysis,Generative adversarial networks,Surface defect
AI 理解论文
溯源树
样例
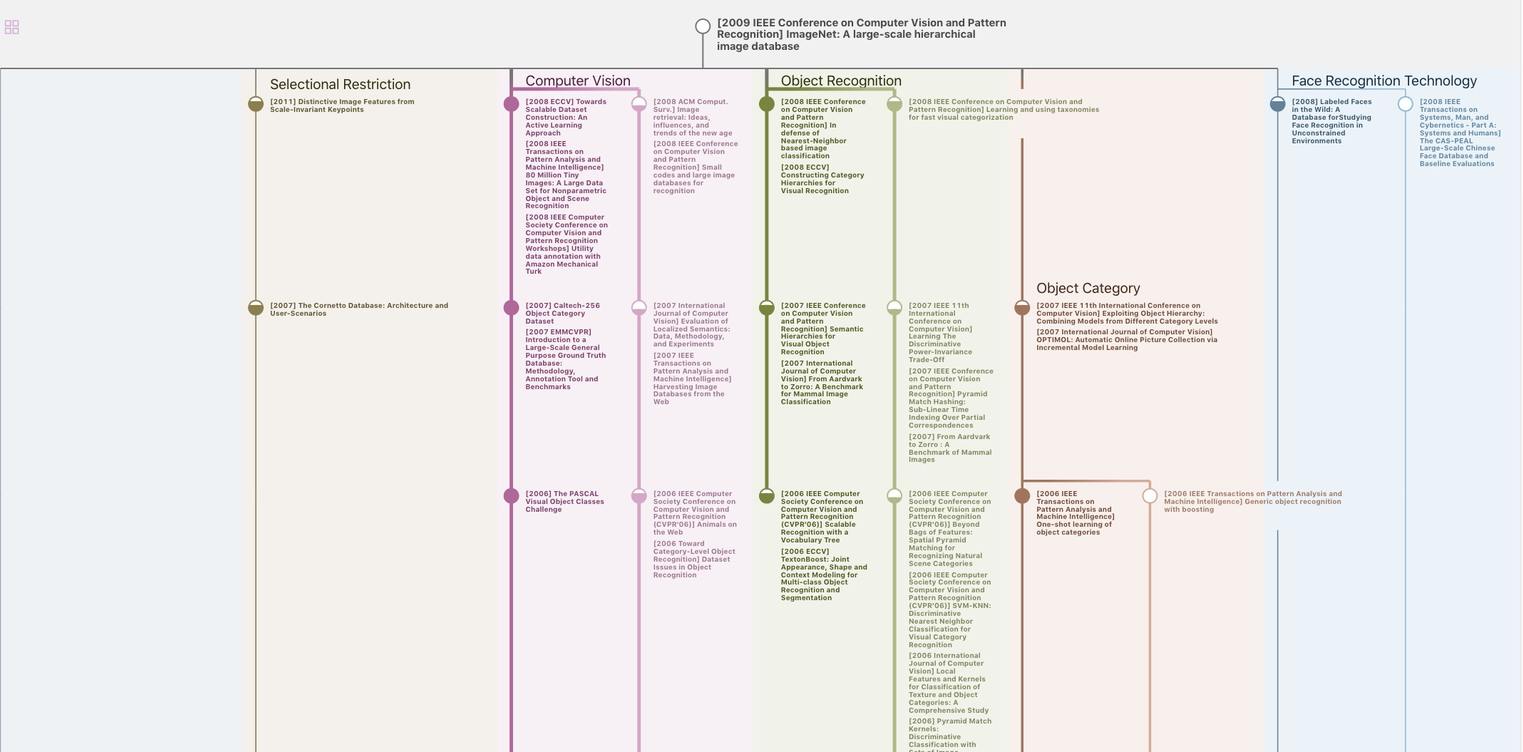
生成溯源树,研究论文发展脉络
Chat Paper
正在生成论文摘要