Multiparametric Magnetic Resonance Imaging (Mri)-Based Radiomics Model Explained by the Shapley Additive Explanations (SHAP) Method for Predicting Complete Response to Neoadjuvant Chemoradiotherapy in Locally Advanced Rectal Cancer: a Multicenter Retrospective Study.
QUANTITATIVE IMAGING IN MEDICINE AND SURGERY(2024)
摘要
Background:Predicting the response to neoadjuvant chemoradiotherapy (nCRT) before initiating treatment is essential for tailoring therapeutic strategies and monitoring prognosis in locally advanced rectal cancer (LARC). In this study, we aimed to develop and validate radiomic-based models to predict clinical and pathological complete responses (cCR and pCR, respectively) by incorporating the Shapley Additive exPlanations (SHAP) method for model interpretation. Methods:A total of 285 patients with complete pretreatment clinical characteristics and T1-weighted (T1W) and T2-weighted (T2W) magnetic resonance imaging (MRI) at 3 centers were retrospectively recruited. The features of tumor lesions were extracted by PyRadiomics and selected using least absolute shrinkage and selection operator (LASSO) algorithm. The selected features were used to build multilayer perceptron (MLP) models alone or combined with clinical features. Area under the receiver operating characteristic curve (AUC), decision curve, and calibration curve were applied to evaluate performance of models. The SHAP method was adopted to explain the prediction models. Results:The radiomic-based models all showed better performances than clinical models. The clinical-radiomic models showed the best differentiation on cCR and pCR with mean AUCs of 0.718 and 0.810 in the validation set, respectively. The decision curves of the clinical-radiomic models showed its values in clinical application. The SHAP method powerfully interpreted the prediction models both at a holistic and individual levels. Conclusions:Our study highlights that the radiomic-based prediction models have more excellent abilities than clinical models and can effectively predict treatment response and optimize therapeutic strategies for patients with LARCs.
更多查看译文
关键词
Radiomics,locally advanced rectal cancer (LARC),SHapley Additive exPlanations (SHAP),machine learning,complete response
AI 理解论文
溯源树
样例
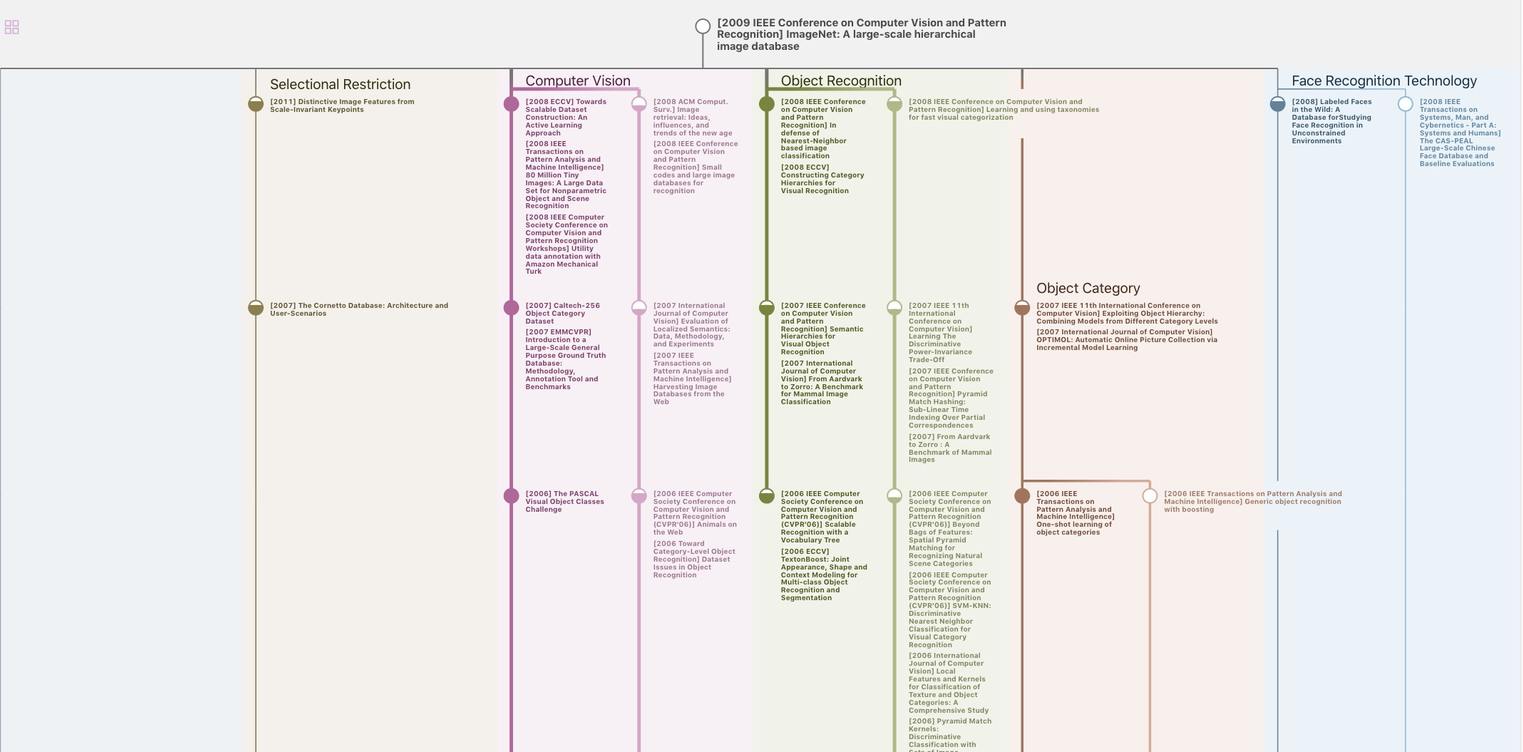
生成溯源树,研究论文发展脉络
Chat Paper
正在生成论文摘要