Dual-former
Digital Signal Processing(2024)
摘要
Recently, image restoration transformers have achieved comparable performance with previous state-of-the-art CNNs. In this work, we present Dual-former whose critical insight is to combine the powerful global modeling ability of self-attention modules and the local modeling ability of convolutions in an overall architecture. With convolution-based Local Feature Extraction modules equipped in the encoder and the decoder, we only adopt a novel Hybrid Transformer Block in the latent layer to model the long-distance dependence in spatial dimensions and handle the uneven distribution between channels. Such a design eliminates the substantial computational complexity in previous image restoration transformers and achieves superior performance on multiple image restoration tasks. Experiments demonstrate that Dual-former achieves a 1.91 dB gain over the state-of-the-art MAXIM method on the Indoor dataset for single image dehazing while consuming only 4.2% GFLOPs as MAXIM. For single image deraining, it exceeds the SOTA method by 0.1 dB PSNR on the average results of five datasets with only 21.5% GFLOPs. Dual-former also substantially surpasses the latest methods on various tasks, with fewer parameters.
更多查看译文
关键词
Image restoration,Local feature extraction,Hybrid self-attention,Adaptive control module,Multi-branch feed-forward network
AI 理解论文
溯源树
样例
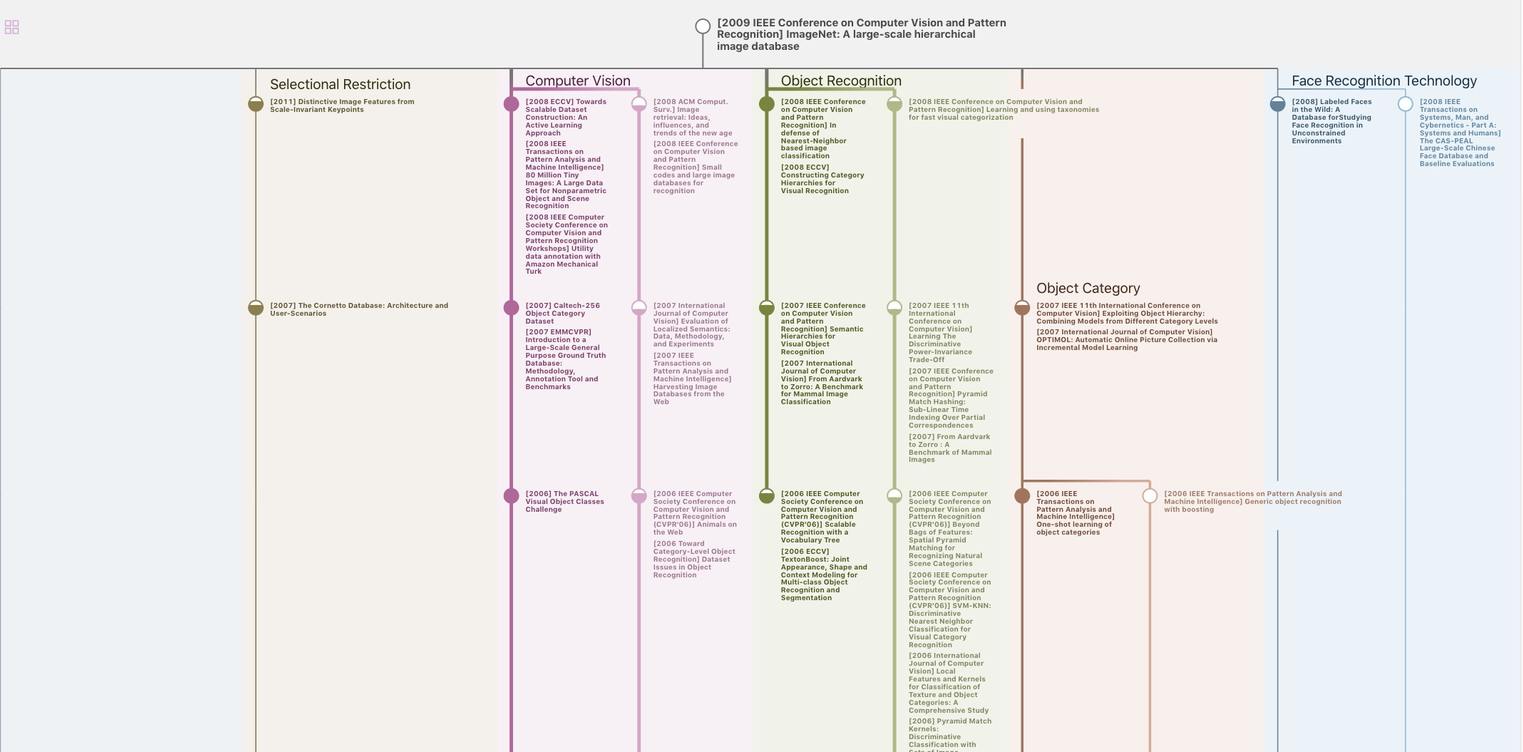
生成溯源树,研究论文发展脉络
Chat Paper
正在生成论文摘要