Language models, like humans, show content effects on reasoning tasks
PNAS NEXUS(2024)
摘要
The Abstract reasoning is a key ability for an intelligent system. Large language models (LMs) achieve above-chance performance on abstract reasoning tasks but exhibit many imperfections. However, human abstract reasoning is also imperfect. Human reasoning is affected by our real-world knowledge and beliefs, and shows notable "content effects"; humans reason more reliably when the semantic content of a problem supports the correct logical inferences. These content-entangled reasoning patterns are central to debates about the fundamental nature of human intelligence. Here, we investigate whether language models-whose prior expectations capture some aspects of human knowledge-similarly mix content into their answers to logic problems. We explored this question across three logical reasoning tasks: natural language inference, judging the logical validity of syllogisms, and the Wason selection task. We evaluate state of the art LMs, as well as humans, and find that the LMs reflect many of the same qualitative human patterns on these tasks-like humans, models answer more accurately when the semantic content of a task supports the logical inferences. These parallels are reflected in accuracy patterns, and in some lower-level features like the relationship between LM confidence over possible answers and human response times. However, in some cases the humans and models behave differently-particularly on the Wason task, where humans perform much worse than large models, and exhibit a distinct error pattern. Our findings have implications for understanding possible contributors to these human cognitive effects, as well as the factors that influence language model performance.
更多查看译文
关键词
language models,content effects,reasoning,logic,cognitive science
AI 理解论文
溯源树
样例
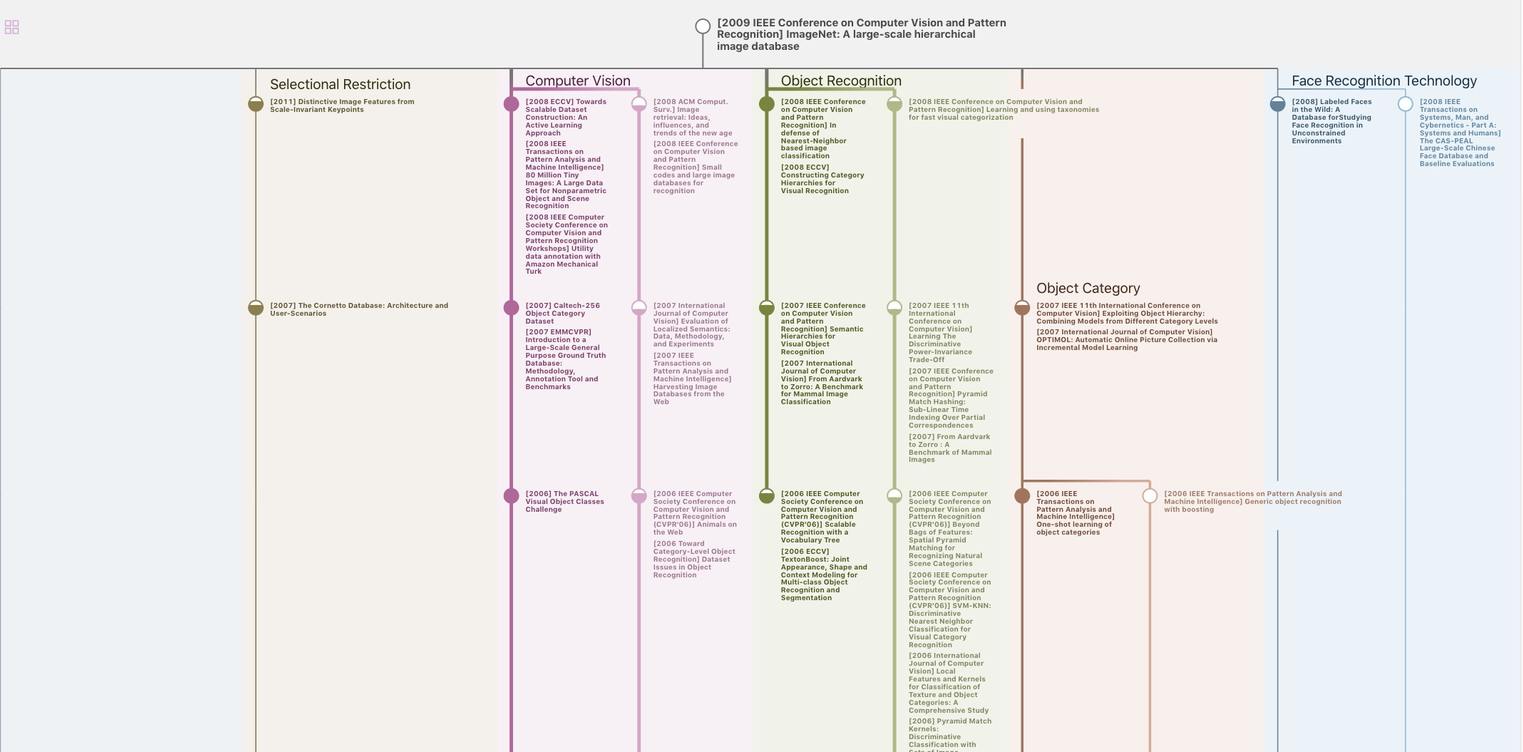
生成溯源树,研究论文发展脉络
Chat Paper
正在生成论文摘要