SKADA-Bench: Benchmarking Unsupervised Domain Adaptation Methods with Realistic Validation
CoRR(2024)
摘要
Unsupervised Domain Adaptation (DA) consists of adapting a model trained on a labeled source domain to perform well on an unlabeled target domain with some data distribution shift. While many methods have been proposed in the literature, fair and realistic evaluation remains an open question, particularly due to methodological difficulties in selecting hyperparameters in the unsupervised setting. With SKADA-Bench, we propose a framework to evaluate DA methods and present a fair evaluation of existing shallow algorithms, including reweighting, mapping, and subspace alignment. Realistic hyperparameter selection is performed with nested cross-validation and various unsupervised model selection scores, on both simulated datasets with controlled shifts and real-world datasets across diverse modalities, such as images, text, biomedical, and tabular data with specific feature extraction. Our benchmark highlights the importance of realistic validation and provides practical guidance for real-life applications, with key insights into the choice and impact of model selection approaches. SKADA-Bench is open-source, reproducible, and can be easily extended with novel DA methods, datasets, and model selection criteria without requiring re-evaluating competitors. SKADA-Bench is available on GitHub at https://github.com/scikit-adaptation/skada-bench.
更多查看译文
AI 理解论文
溯源树
样例
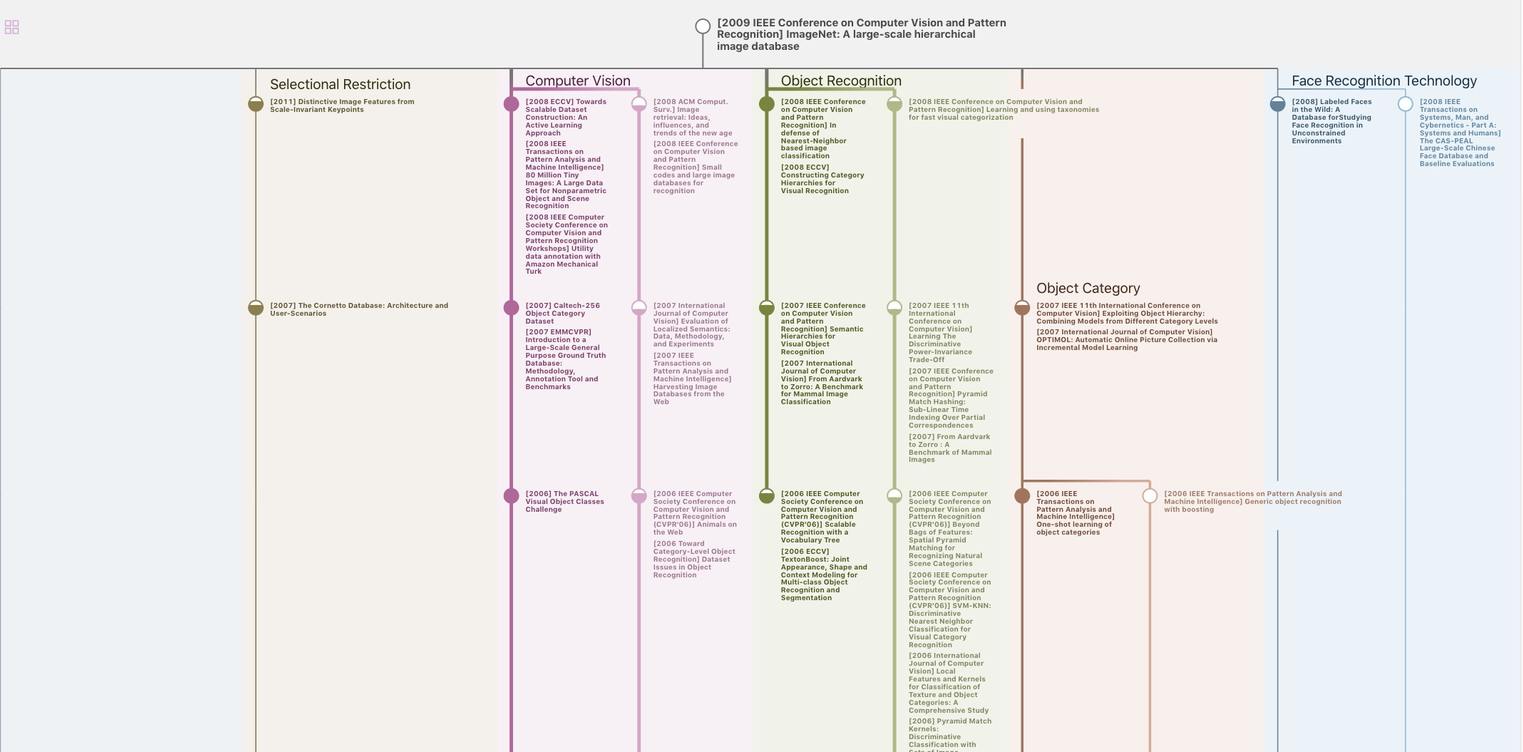
生成溯源树,研究论文发展脉络
Chat Paper
正在生成论文摘要