U2Net-MGP: A Lightweight and Efficient Visual Perception Algorithm for Consumer Electronic Accessories
IEEE Transactions on Consumer Electronics(2024)
摘要
In the assembly of consumer electronic products, target detection methods offer details on the target’s location and category, but precise positioning with the robotic arm’s end-effector demands pixel-level edge contour data of the target. As a result, we’ve developed U2Net-MGP, a lightweight and efficient visual perception model. This model effectively captures edge contours for positioning consumer electronic components. Firstly, the residual U-blocks (RSU) in U2Net have been replaced with the ghost convolution residual U-blocks (GRSU) as designed in this paper. This change reduces the complexity of the model while improving accuracy. Furthermore, we’ve introduced polarized self-attention and created a polarized self-attention feature fusion module (PFF). This innovation enables the model to capture both local and global information effectively, enhances the modeling capacity of the feature data, and ultimately improves the accuracy of pixel regression. In this paper, we conducted ablation experiments and comparative experiments on the consumer electronics components dataset. The results reveal that the U2Net-MGP model is both compact and efficacious, markedly bolstering segmentation capability while reducing to 72.3% of the baseline model’s size. Relative to the original, it manifests increases of 3.2%, 5.3%, 3.6%, and 4.2% in the precision, recall, Fβ, and mean absolute error, respectively.
更多查看译文
关键词
Consumer Electronics,Salient object segmentation,Ghost convolution,Polarized self-attention mechanism,Multi-scale feature fusion
AI 理解论文
溯源树
样例
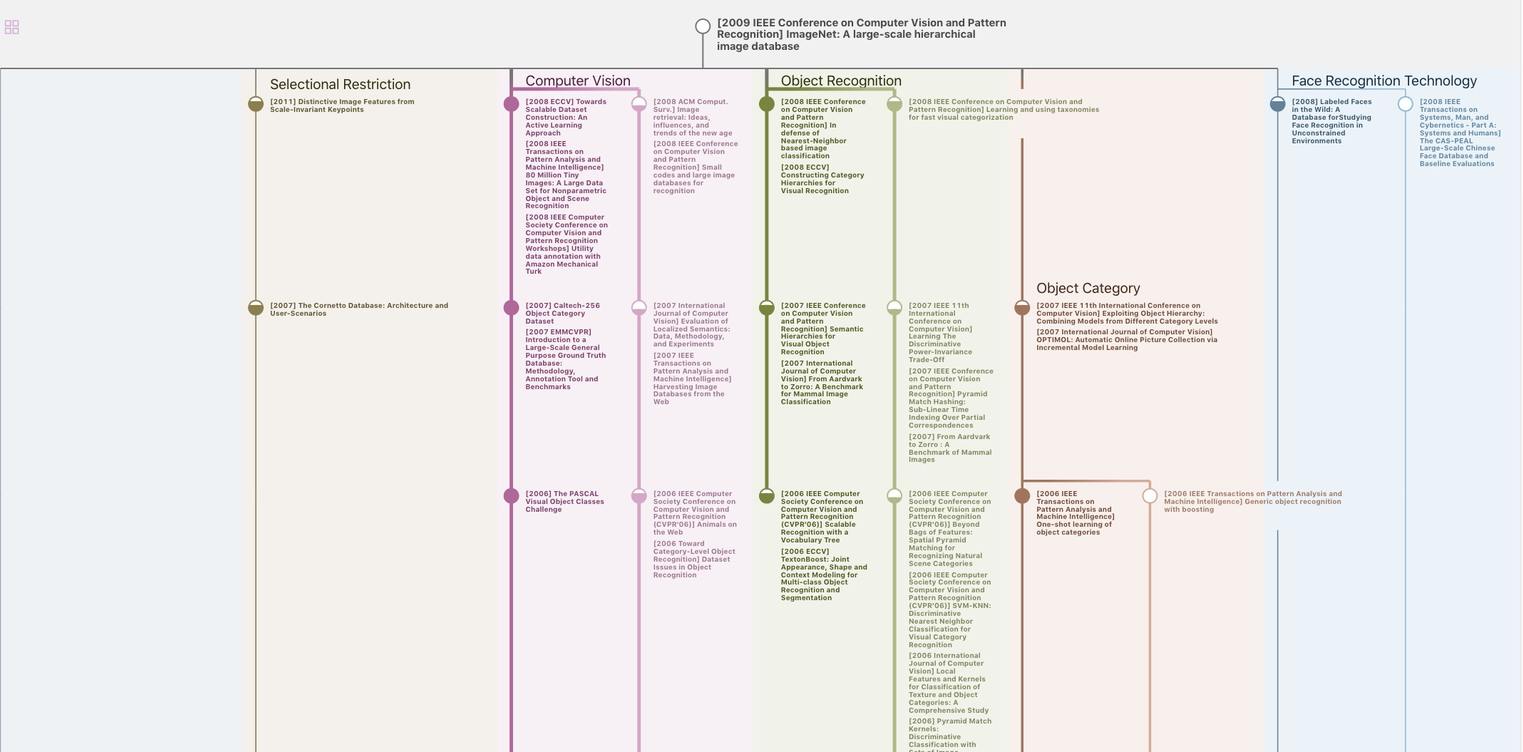
生成溯源树,研究论文发展脉络
Chat Paper
正在生成论文摘要