Abnormal Fastener Recognition Via Dual-branch Supervised Contrastive Learning Network with Hard Feature Synthesis
IEEE Sensors Journal(2024)
Abstract
Visual recognition of abnormal fasteners is of vital practical significance for rails to maintain the safe operation. However, affected by imaging conditions and other complex external factors, the captured fastener images within the same class may possess quite different characteristics, while those of different classes might have similar visual appearances, making it still a great challenge for the inspection system to achieve accurate recognition of them. To this end, this paper presents a dual-branch supervised contrastive learning (DSCL) network, in which the contrastive learning branch is designed to facilitate extracting discriminative features, and the classification branch utilizes the resulting features for classifier optimization and abnormal fastener recognition. Considering the intra-class diversity and inter-class similarity of fastener images, an intuitive and feasible hard feature synthesis strategy is instantiated by linearly interpolating projections of the same or different categories of fastener images, which in essence simulates the representations of indistinguishable samples in the training process of the model and thus contributes to strengthening its recognition capability. Extensive experiments are conducted on the constructed real-world fastener image dataset, and the results demonstrate that DSCL outperforms the state-of-the-art methods in recognizing abnormal fasteners with a relatively lower computational complexity.
MoreTranslated text
Key words
Abnormal fastener recognition,supervised contrastive learning,intra-class diversity,inter-class similarity,hard feature synthesis
AI Read Science
Must-Reading Tree
Example
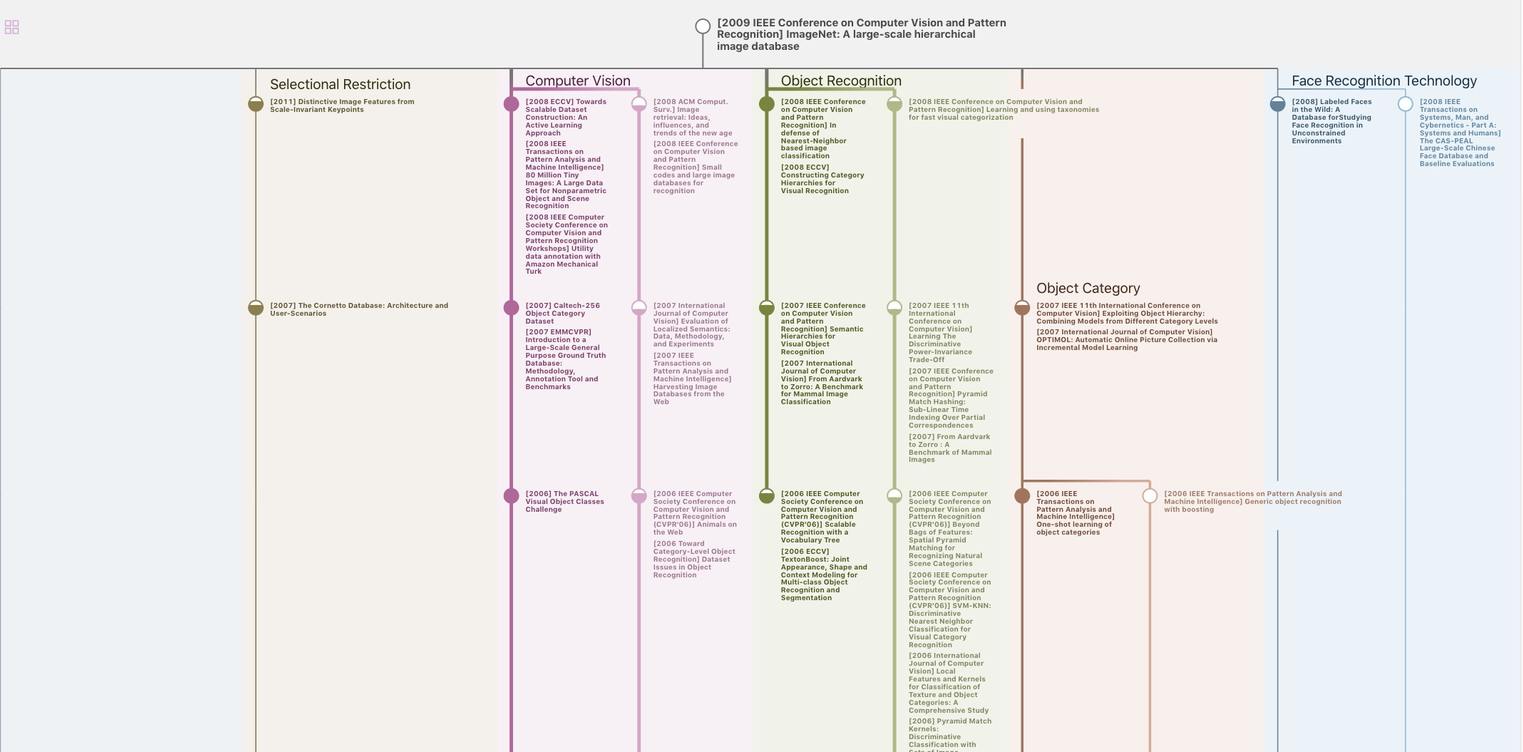
Generate MRT to find the research sequence of this paper
Chat Paper
Summary is being generated by the instructions you defined