Measure for Semantics and Semantically Constrained Pose Optimization: A Review
IEEE Transactions on Instrumentation and Measurement(2024)
摘要
Simultaneous localization and mapping (SLAM) in robotics is a fundamental problem. The use of visual odometry (VO) enhances scene recognition in the task of ego-localization within an unknown environment. Semantically meaningful information permits data association and dense mapping to be conducted based on entities representing landmarks rather than manually designed, low-level geometric clues and has inspired various feature descriptors for semantically ensembled SLAM applications. This article illuminates the insights into the measure for semantics and the semantically constrained pose optimization. The concept of semantic extractor and the matched framework are initially presented. As the latest advances in computer vision and the learning-based deep feature acquisition are closely related, the semantic extractor is especially described in a deep learning paradigm. The methodologies pertinent to our explorations for object association and semantics-fused constraining that is amenable for use in a least-squares framework are summarized in a systematic way. By a collection of problem formulations and principle analyses, our review exhibits a fairly unique perspective in semantic SLAM. We further discuss the challenges of semantic uncertainty and explicitly introduce the term ‘semantic reasoning’. Some technology outlooks regarding semantic reasoning are simultaneously given. We argue that for intelligent tasks of robots such as object grasping, dynamic obstacle avoidance, and object-target navigation, semantic reasoning might guide the complex scene understanding under the framework of semantic SLAM directly to a solution.
更多查看译文
关键词
semantic extractor,object association,camera pose optimization,deep learning,semantic reasoning
AI 理解论文
溯源树
样例
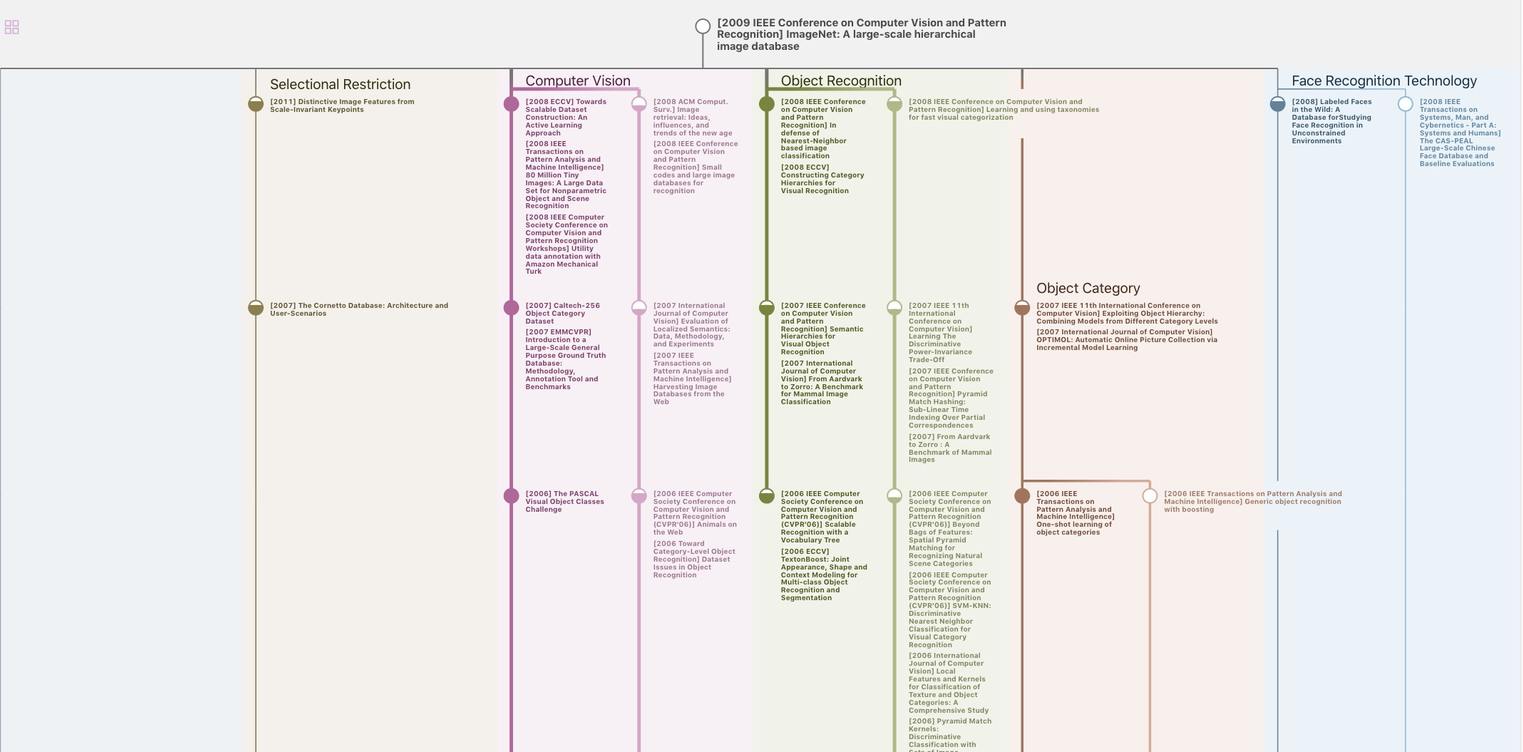
生成溯源树,研究论文发展脉络
Chat Paper
正在生成论文摘要