Reading Between the Lines: Machine Learning Ensemble and Deep Learning for Implied Threat Detection in Textual Data
International Journal of Computational Intelligence Systems(2024)
摘要
With the increase in the generation and spread of textual content on social media, natural language processing (NLP) has become an important area of research for detecting underlying threats, racial abuse, violence, and implied warnings in the content. The subtlety and ambiguity of language make the development of effective models for detecting threats in text a challenging task. This task is further complicated when the threat is not explicitly conveyed. This study focuses on the task of implied threat detection using an explicitly designed machine-generated dataset with both linguistic and lexical features. We evaluated the performance of different machine learning algorithms on these features including Support Vector Machines, Logistic Regression, Naive Bayes, Decision Tree, and K-nearest neighbors. The ensembling approaches of Adaboost, Random Forest, and Gradient Boosting were also explored. Deep learning modeling was performed using Long Short-Term Memory, Deep Neural Networks (DNN), and Bidirectional Long Short-Term Memory (BiLSTM). Based on the evaluation, it was observed that classical and ensemble models overfit while working with linguistic features. The performance of these models improved when working with lexical features. The model based on logistic regression exhibited superior performance with an F1 score of 77.13
更多查看译文
关键词
Implied threat detection,Text mining,Linguistic feature analysis,Feature engineering,Social media,Text classification
AI 理解论文
溯源树
样例
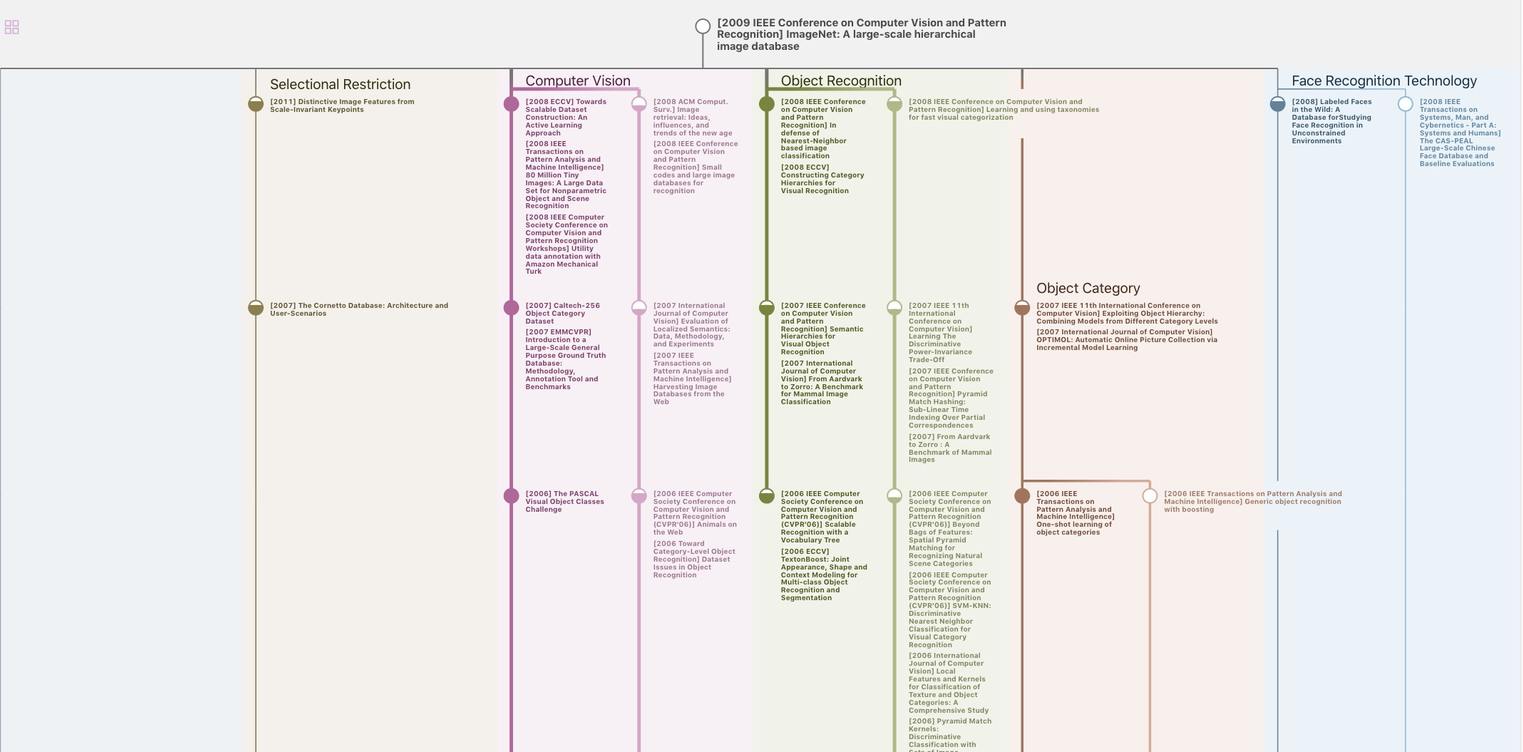
生成溯源树,研究论文发展脉络
Chat Paper
正在生成论文摘要