DP-MP: a novel cross-subject fatigue detection framework with DANN-based prototypical representation and mix-up pairwise learning.
Journal of neural engineering(2024)
摘要
OBJECTIVE:Electroencephalography (EEG) is widely recognized as an effective method for detecting fatigue. However, practical applications of EEG for fatigue detection in real-world scenarios are often challenging, particularly in cases involving subjects not included in the training datasets, owing to bio-individual differences and noisy labels. This study aims to develop an effective framework for cross-subject fatigue detection by addressing these challenges.
APPROACH:In this study, we propose a novel framework, termed DP-MP, for cross-subject fatigue detection, which utilizes a Domain-Adversarial Neural Network (DANN)-based prototypical representation in conjunction with Mix-up pairwise learning. Our proposed DP-MP framework aims to mitigate the impact of bio-individual differences by encoding fatigue-related semantic structures within EEG signals and exploring shared fatigue prototype features across individuals. Notably, to the best of our knowledge, this work is the first to conceptualize fatigue detection as a pairwise learning task, thereby effectively reducing the interference from noisy labels. Furthermore, we propose the Mix-up pairwise learning (MixPa) approach in the field of fatigue detection, which broadens the advantages of pairwise learning by introducing more diverse and informative relationships among samples.
RESULTS:Cross-subject experiments were conducted on two benchmark databases, SEED-VIG and FTEF, achieving state-of-the-art performance with average accuracies of 88.14% and 97.41%, respectively. These promising results demonstrate our model's effectiveness and excellent generalization capability.
SIGNIFICANCE:This is the first time EEG-based fatigue detection has been conceptualized as a pairwise learning task, offering a novel perspective to this field. Moreover, our proposed DP-MP framework effectively tackles the challenges of bio-individual differences and noisy labels in the fatigue detection field and demonstrates superior performance. Our work provides valuable insights for future research, promoting the application of brain-computer interfaces for fatigue detection in real-world scenarios.
.
更多查看译文
AI 理解论文
溯源树
样例
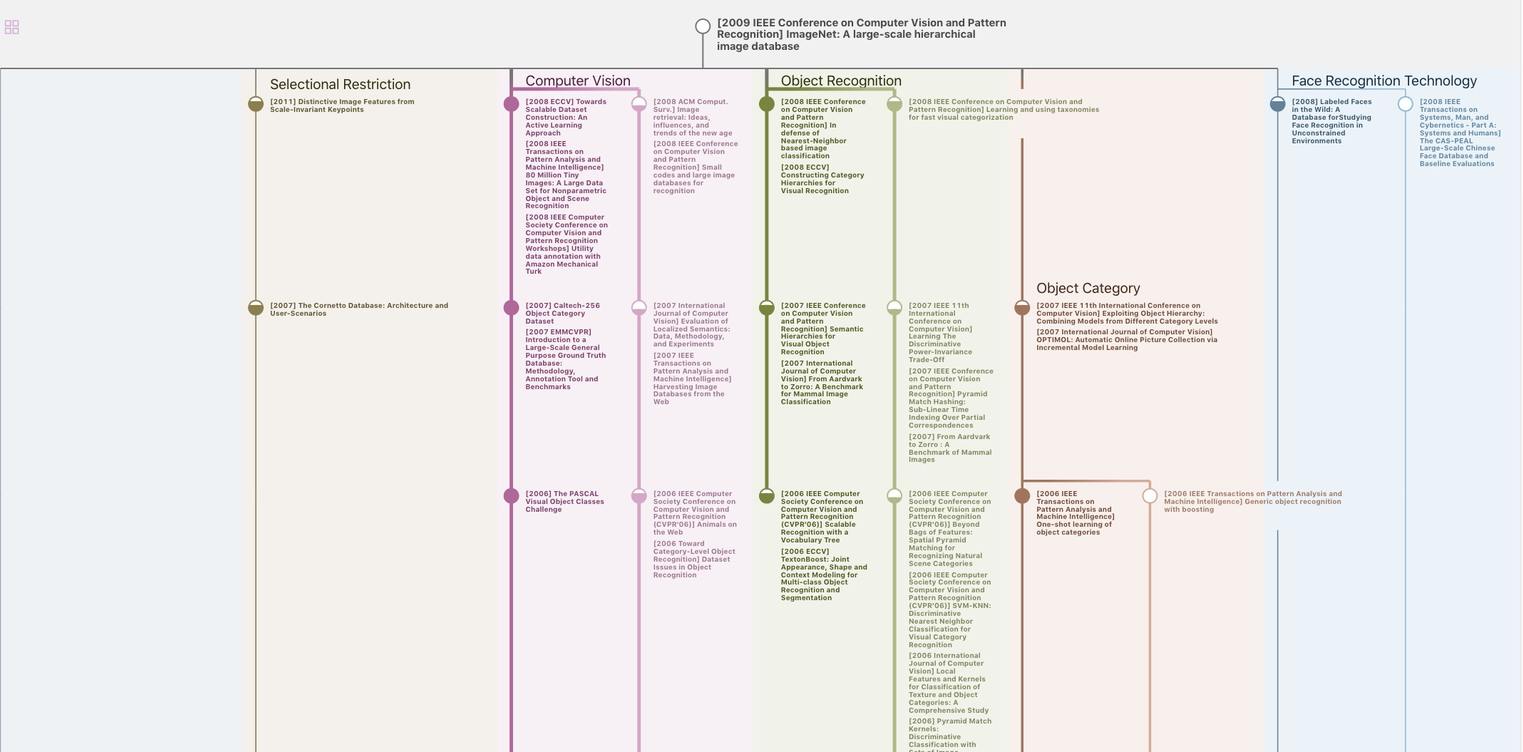
生成溯源树,研究论文发展脉络
Chat Paper
正在生成论文摘要