From Easy to Hard: Learning Curricular Shape-aware Features for Robust Panoptic Scene Graph Generation
INTERNATIONAL JOURNAL OF COMPUTER VISION(2024)
摘要
Panoptic Scene Graph Generation (PSG) aims to generate a comprehensive graph-structure representation based on panoptic segmentation masks. Despite remarkable progress in PSG, almost all existing methods neglect the importance of shape-aware features, which inherently focus on the contours and boundaries of objects. To bridge this gap, we propose a model-agnostic Curricular shApe-aware FEature (CAFE) learning strategy for PSG. Specifically, we incorporate shape-aware features (i.e., mask features and boundary features) into PSG, moving beyond reliance solely on bbox features. Furthermore, drawing inspiration from human cognition, we propose to integrate shape-aware features in an easy-to-hard manner. To achieve this, we categorize the predicates into three groups based on cognition learning difficulty and correspondingly divide the training process into three stages. Each stage utilizes a specialized relation classifier to distinguish specific groups of predicates. As the learning difficulty of predicates increases, these classifiers are equipped with features of ascending complexity. We also incorporate knowledge distillation to retain knowledge acquired in earlier stages. Due to its model-agnostic nature, CAFE can be seamlessly incorporated into any PSG model. Extensive experiments and ablations on two PSG tasks under both robust and zero-shot PSG have attested to the superiority and robustness of our proposed CAFE, which outperforms existing state-of-the-art methods by a large margin.
更多查看译文
关键词
Panoptic scene graph generation,Robust learning,Shape-aware features,Curriculum learning,Novel class discovery
AI 理解论文
溯源树
样例
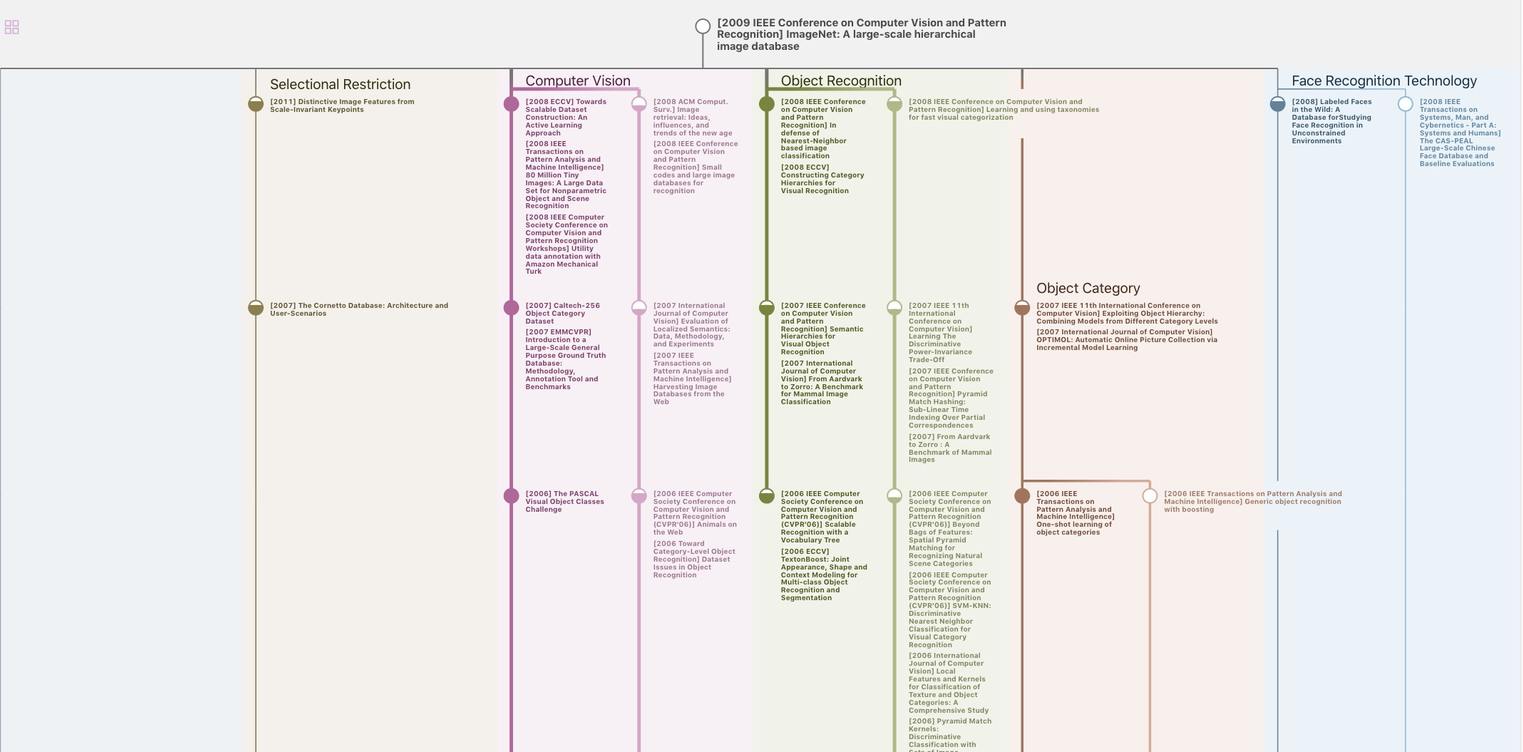
生成溯源树,研究论文发展脉络
Chat Paper
正在生成论文摘要