Needle Tracking in Low-Resolution Ultrasound Volumes Using Deep Learning
International journal of computer assisted radiology and surgery(2024)
摘要
Clinical needle insertion into tissue, commonly assisted by 2D ultrasound imaging for real-time navigation, faces the challenge of precise needle and probe alignment to reduce out-of-plane movement. Recent studies investigate 3D ultrasound imaging together with deep learning to overcome this problem, focusing on acquiring high-resolution images to create optimal conditions for needle tip detection. However, high-resolution also requires a lot of time for image acquisition and processing, which limits the real-time capability. Therefore, we aim to maximize the US volume rate with the trade-off of low image resolution. We propose a deep learning approach to directly extract the 3D needle tip position from sparsely sampled US volumes. We design an experimental setup with a robot inserting a needle into water and chicken liver tissue. In contrast to manual annotation, we assess the needle tip position from the known robot pose. During insertion, we acquire a large data set of low-resolution volumes using a 16 × 16 element matrix transducer with a volume rate of 4 Hz. We compare the performance of our deep learning approach with conventional needle segmentation. Our experiments in water and liver show that deep learning outperforms the conventional approach while achieving sub-millimeter accuracy. We achieve mean position errors of 0.54 mm in water and 1.54 mm in liver for deep learning. Our study underlines the strengths of deep learning to predict the 3D needle positions from low-resolution ultrasound volumes. This is an important milestone for real-time needle navigation, simplifying the alignment of needle and ultrasound probe and enabling a 3D motion analysis.
更多查看译文
关键词
Volumetric ultrasound imaging,Deep learning,Needle tip detection,Real-time,Sparse feature learning
AI 理解论文
溯源树
样例
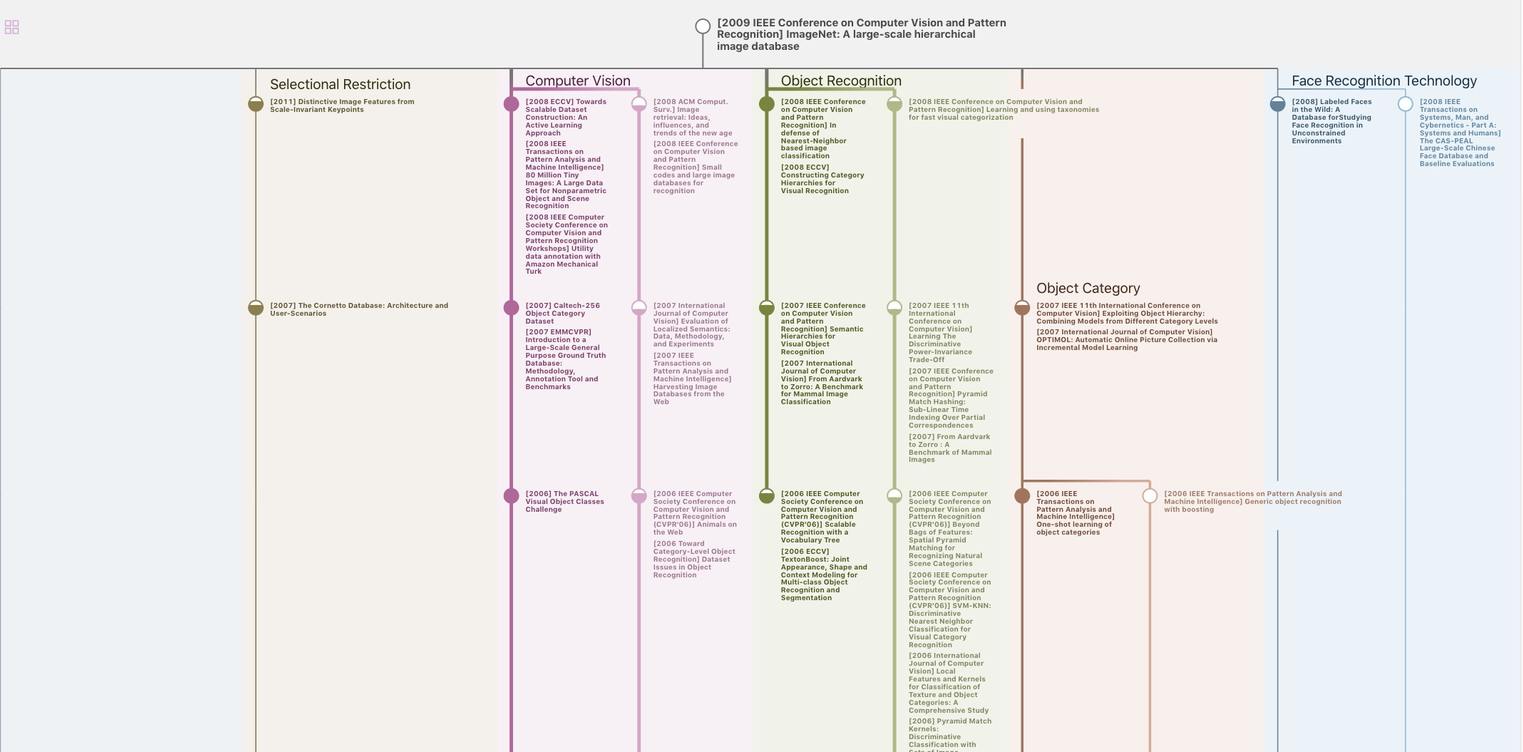
生成溯源树,研究论文发展脉络
Chat Paper
正在生成论文摘要