Classification of Raw Single-Trial EEG/ERP Signals of a Combined Subjects' Data Sample in Response to the Presentation of Mathematical Sums, Correct and Incorrect Answers to Them, Using CNNs
2024 V International Conference on Neural Networks and Neurotechnologies (NeuroNT)(2024)
摘要
The use of convolutional neural networks (CNNs) is one of the effective approaches for classification of electroencephalographic (EEG) data. In this paper we perform a comparative analysis of CNN application for classification of 3 classes of data (single-trial approach) - raw signal of brain event-related potentials (ERP) in response to correct, incorrect answers to the mathematical problems and presentation of the math sums themselves. Single-trial sample sets of all subjects (20 subjects) formed the total data sample for classification, in which ~100 samples from each subject were included in each class (correct/incorrect answer, presentation of math sums). Non-overlapping 80% and 20% samples were used for training and testing, respectively. The paper tests the ResNet18 architecture and classification accuracy of the raw EEG signals for ERP windows of 200 to 500 ms duration (in 100 ms steps) from the onset of stimulus presentation. The best classification accuracy reached 72 ± 3 %. Classification into correct and incorrect answers gave the highest mistakes, at the same time error of distinguishing visual samples of ERPs with the presentation of mathematical sums was about twice lower. Classification of the raw EEG/ ERP signal and its characteristics in response to the presentation of correct/erroneous answers can be used in the organization of feedback for brain-computer interfaces (BCIs).
更多查看译文
关键词
EEG,ERP,classification,single-trial approach,convolutional neural networks,ResNet18,BCI
AI 理解论文
溯源树
样例
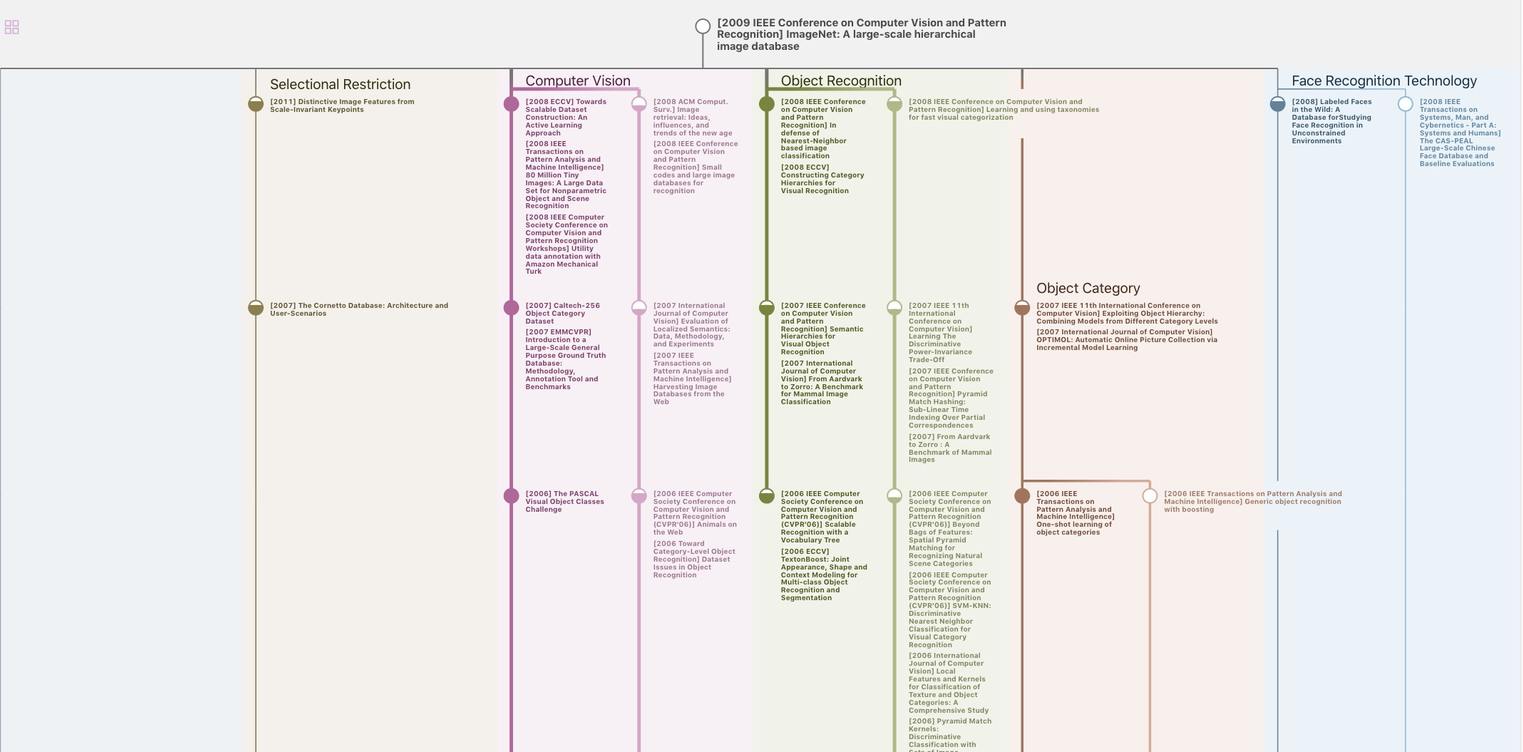
生成溯源树,研究论文发展脉络
Chat Paper
正在生成论文摘要