Privacy Preservation for Federated Learning in Health Care
Patterns(2024)
摘要
Artificial intelligence (AI) shows potential to improve health care by leveraging data to build models that can inform clinical workflows. However, access to large quantities of diverse data is needed to develop robust generalizable models. Data sharing across institutions is not always feasible due to legal, security, and privacy concerns. Federated learning (FL) allows for multi-institutional training of AI models, obviating data sharing, albeit with different security and privacy concerns. Specifically, insights exchanged during FL can leak information about institutional data. In addition, FL can introduce issues when there is limited trust among the entities performing the compute. With the growing adoption of FL in health care, it is imperative to elucidate the potential risks. We thus summarize privacy-preserving FL literature in this work with special regard to health care. We draw attention to threats and review mitigation approaches. We anticipate this review to become a health-care researcher’s guide to security and privacy in FL.
更多查看译文
关键词
federated learning,health care,privacy,security,review article
AI 理解论文
溯源树
样例
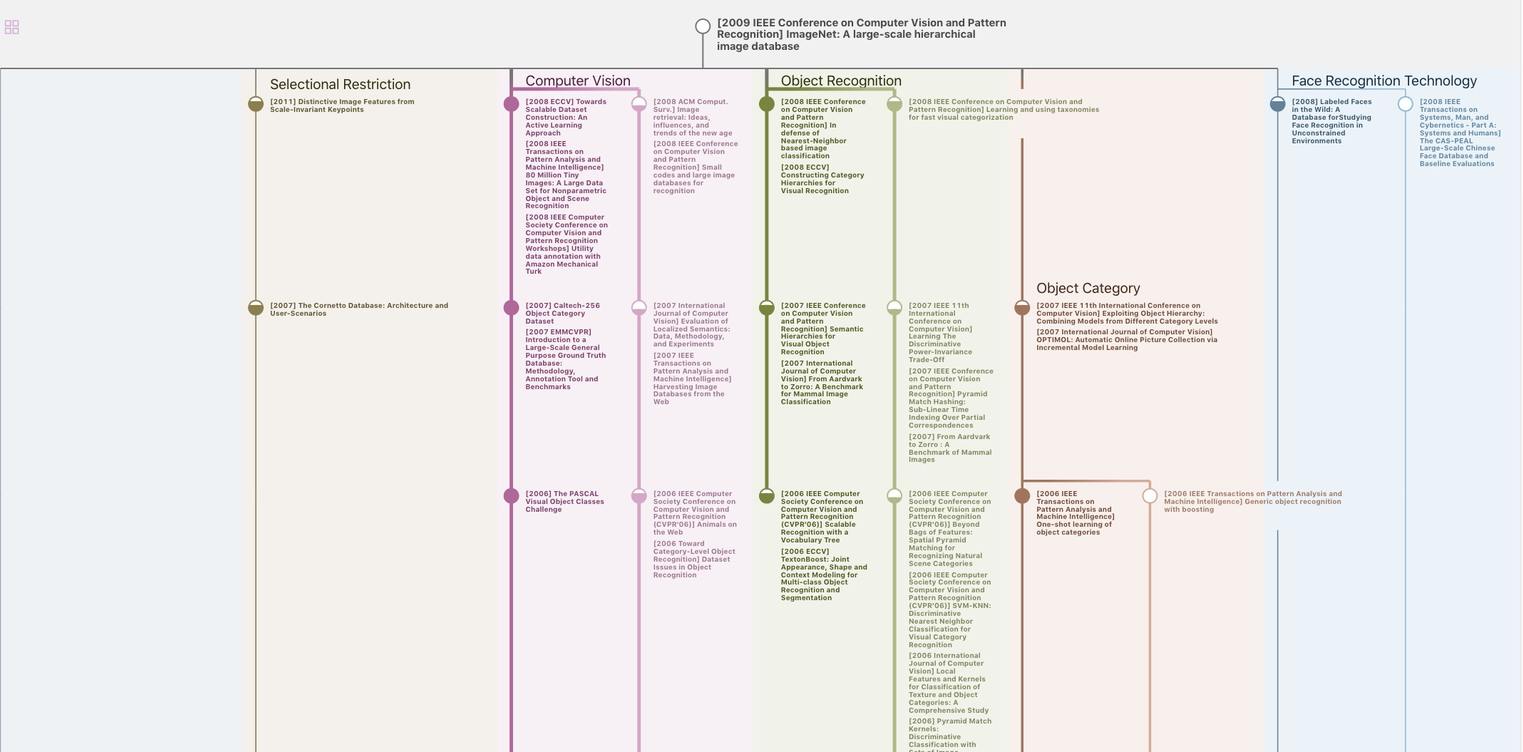
生成溯源树,研究论文发展脉络
Chat Paper
正在生成论文摘要