A Novel Point Cloud Registration Method for Multimedia Communication in Automated Driving Metaverse
ACM Transactions on Internet Technology(2024)
摘要
The development of the Metaverse offers more possibilities for autonomous driving. This is mainly reflected in the fact that the scene reconstructed based on multiple sensors can help the autonomous vehicle establish a Metaverse world based on its own real situation. When multiple vehicles build such a Metaverse world in the same scene, they can exchange their information including the perception of the vehicle’s condition and the surrounding environment, which means the creation of a Metaverse world containing all vehicles. Thus, an indirect Human-Human Multimedia Communications based on the Metaverse is realized, in which the autonomous driving system acts as a multimedia to provide a medium for the exchange of information between vehicles. The establishment of the Metaverse is based on stable and high-quality scene reconstruction. Accurate scene information can bring high quality Human-Human Multimedia Communications. Achieving this requires accurate scene reconstruction and vehicle positioning, both of which depend on accurate point cloud registration. In this work, we propose a robust registration method that is based on semantic information and scaling constraints. Our method consists of three steps. Firstly, we filter out points that might mislead the point cloud registration process by leveraging semantic information. Secondly, we obtain a more accurate initial matrix using TEASER++, which is based on semantic information and feature descriptors. Finally, we use semantic information and scaling to constrain the nearest neighbor matching and filter out error matches to obtain a higher quality registration. By following these steps, our method overcomes the memory problem faced by TEASER++ when there are large-scale point clouds, and greatly reduces its running time. Meanwhile, our algorithm achieved superior point cloud registration results compared to two state-of-the-art robust registration techniques: Globally optimal Iterative Closest Point (Go-ICP) and Generalized Iterative Closest Point (GICP).
更多查看译文
关键词
Point Cloud Registration,Semantic Information,Scale Constraints,Human-Human Multimedia Communications,Metaverse
AI 理解论文
溯源树
样例
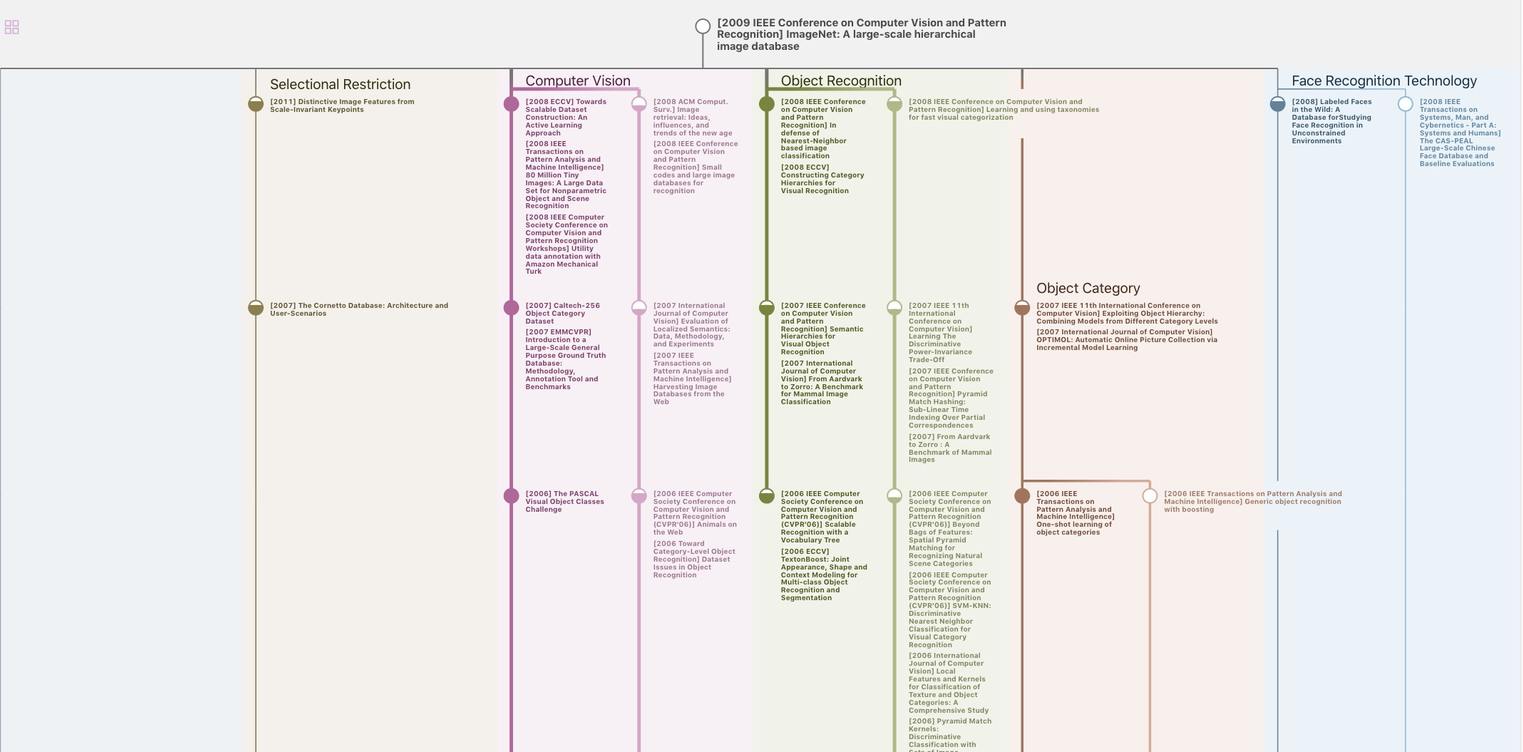
生成溯源树,研究论文发展脉络
Chat Paper
正在生成论文摘要