Uncalibrated Multi-view 3D Human Pose Estimation with Geometry Driven Attention
2024 IEEE 18th International Conference on Automatic Face and Gesture Recognition (FG)(2024)
摘要
To make up for the inherent challenging nature of 3D pose estimation, most multi-view frameworks rely on camera calibration, often leading to impractical or constrained architectures. Accurate human pose estimation is key to en-hancing human-computer interaction, gaming, health, sport and surveillance systems. By capturing precise and reliable body positions, our approach enables efficient and innovative downstream tasks. We leverage monocular 3D pose estimations and a novel geometry driven attention mechanism inside of a transformer lightweight architecture to produce high precision, occlusion aware refined 3D poses, with varying number of uncalibrated cameras. Our method shows competitive results on the in-lab dataset Human3.6M and in the in-the-wild environment of SkiPose PTZ-Camera, both in camera frames or in a disentangled person centric referential allowing practical downstream uses. Our approach matches state-of-the-art performance on Human3.6M, while being at least 3 times lighter. On the SkiPose base acquired under particularly difficult conditions, our results exceed those of the state of the art by being at least 3 times faster.
更多查看译文
关键词
Pose Estimation,Human Pose Estimation,Attention Mechanism,Monocular,Camera Frame,Camera Calibration,3D Pose,Athletes,Single Image,3D Coordinates,3D Position,Vector Core,Linear Layer,2D Projection,Single Camera,Camera Angle,Transformer Architecture,Positional Encoding,Mean Aggregation,Precise Calibration,Aggregation Module,2D Pose,Embedding Module,2D Keypoints,Masking Strategy,3D Skeleton,3D Input
AI 理解论文
溯源树
样例
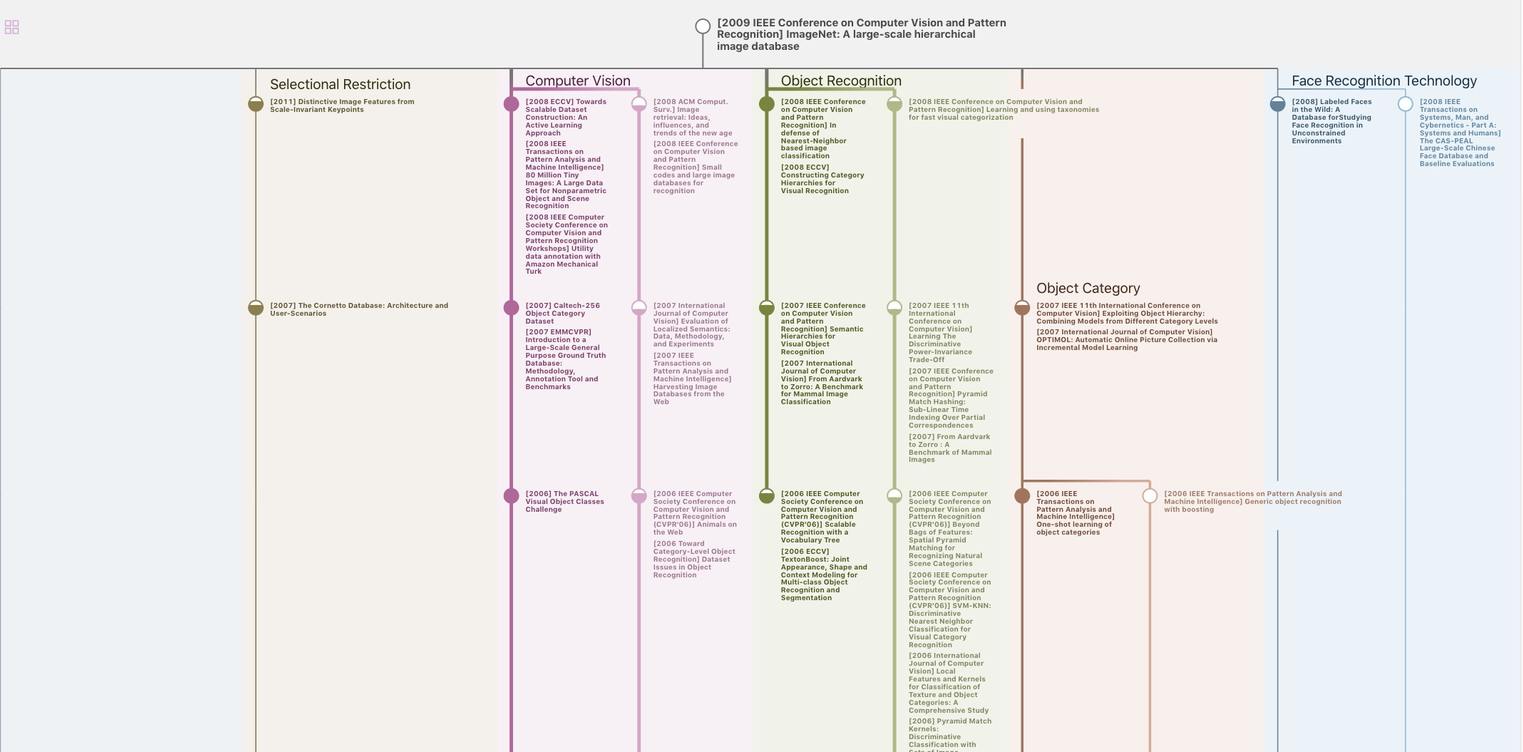
生成溯源树,研究论文发展脉络
Chat Paper
正在生成论文摘要