An Accelerated Stochastic ADMM for Nonconvex and Nonsmooth Finite-Sum Optimization
Automatica (Journal of IFAC)(2024)
摘要
The nonconvex and nonsmooth finite-sum optimization problem with linear constraint has attracted much attention in the fields of artificial intelligence, computer, and mathematics, due to its wide applications in machine learning and the lack of efficient algorithms with convincing convergence theories. A popular approach to solve it is the stochastic Alternating Direction Method of Multipliers (ADMM), but most stochastic ADMM-type methods focus on convex models. In addition, the variance reduction (VR) and acceleration techniques are useful tools in the development of stochastic methods due to their simplicity and practicability in providing acceleration characteristics of various machine learning models. However, it remains unclear whether accelerated SVRG-ADMM algorithm (ASVRG-ADMM), which extends SVRG-ADMM by incorporating momentum techniques, exhibits a comparable acceleration characteristic or convergence rate in the nonconvex setting. To fill this gap, we consider a general nonconvex nonsmooth optimization problem and study the convergence of ASVRG-ADMM. By utilizing a well-defined potential energy function, we establish its sublinear convergence rate O ( 1 / T ), where T denotes the iteration number. Furthermore, under the additional Kurdyka–Lojasiewicz (KL) property which is less stringent than the frequently used conditions for showcasing linear convergence rates, such as strong convexity, we show that the ASVRG-ADMM sequence almost surely has a finite length and converges to a stationary solution with a linear convergence rate. Several experiments on solving the graph-guided fused lasso problem and regularized logistic regression problem validate that the proposed ASVRG-ADMM performs better than the state-of-the-art methods.
更多查看译文
关键词
Nonconvex and nonsmooth optimization,Acceleration technique,Stochastic ADMM,Linear convergence
AI 理解论文
溯源树
样例
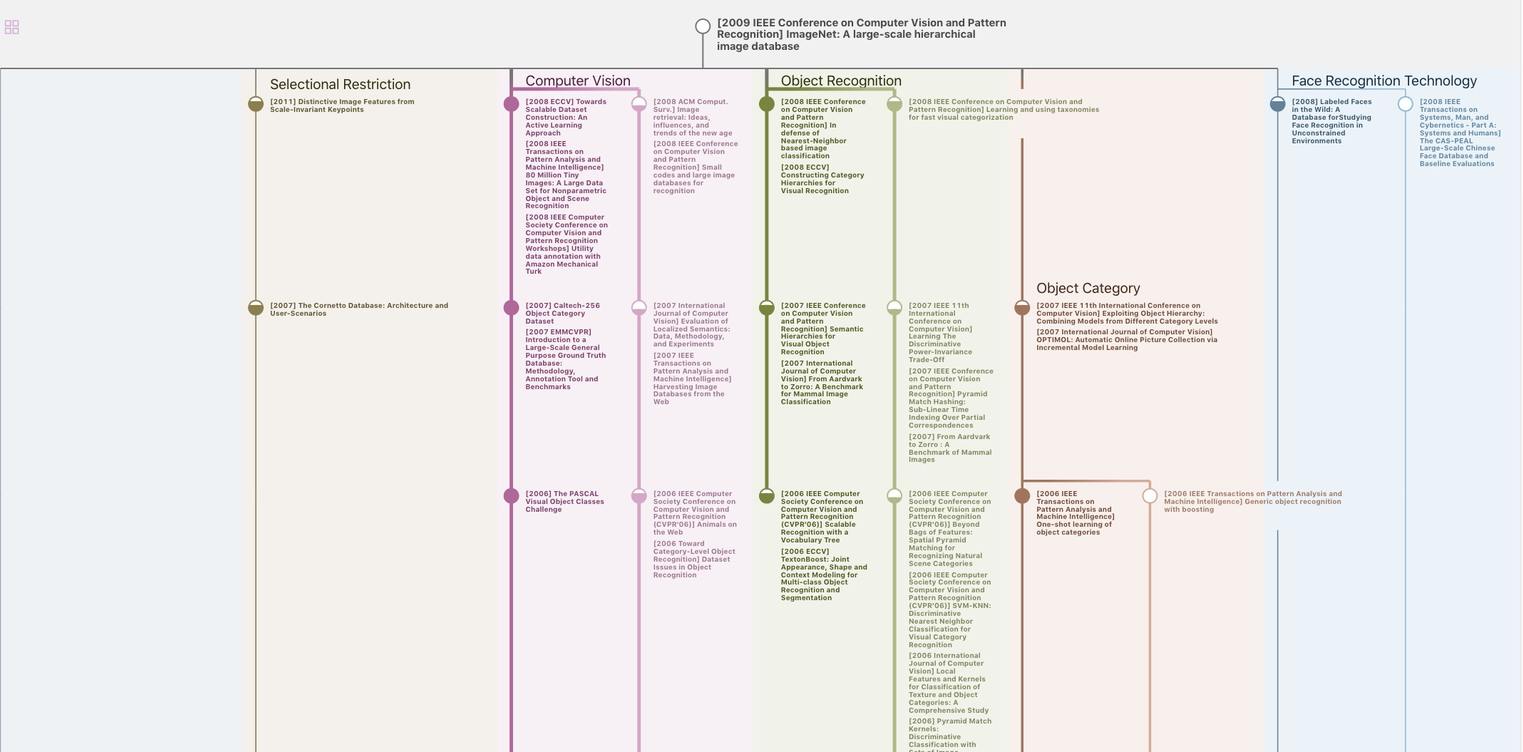
生成溯源树,研究论文发展脉络
Chat Paper
正在生成论文摘要