A Deep Neural Network for Operator Learning Enhanced by Attention and Gating Mechanisms for Long-Time Forecasting of Tumor Growth
ENGINEERING WITH COMPUTERS(2024)
摘要
Forecasting tumor progression and assessing the uncertainty of predictions play a crucial role in clinical settings, especially for determining disease outlook and making informed decisions about treatment approaches. In this work, we propose TGM-ONets, a deep neural operator learning (PI-DeepONet) based computational framework, which combines bioimaging and tumor growth modeling (TGM) for enhanced prediction of tumor growth. Deep neural operators have recently emerged as a powerful tool for learning the solution maps between the function spaces, and they have demonstrated their generalization capability in making predictions based on unseen input instances once trained. Incorporating the physics laws into the loss function of the deep neural operator can significantly reduce the amount of the training data. The novelties of the design of TGM-ONets include the employment of a convolutional block attention module (CBAM) and a gating mechanism (i.e., mixture of experts (MoE)) to extract the features of the input images. Our results show that the TGM-ONets not only can capture the detailed morphological characteristics of the mild and aggressive tumors within and outside the training domain but also can be used to predict the long-term dynamics of both mild and aggressive tumor growth for up to 6 months with a maximum error of less than 6.7 × 10^-2 for unseen input instances with two or three snapshots added. We also systematically study the effects of the number of training snapshots and noisy data on the performance of TGM-ONets as well as quantify the uncertainty of the model predictions. We demonstrate the efficiency and accuracy by comparing the performance of TGM-ONets with three state-of-the-art (SOTA) baseline models. In summary, we propose a new deep learning model capable of integrating the TGM and sequential observations of tumor morphology to improve the current approaches for predicting tumor growth and thus provide an advanced computational tool for patient-specific tumor prognosis.
更多查看译文
关键词
Physics-informed deep operator networks (PI-DeepONet),Convolutional block attention module (CBAM),Mixture of experts (MoE),Tumor growth model (TGM),Phase-field approach,Uncertainty quantification (UQ),Long-time prediction
AI 理解论文
溯源树
样例
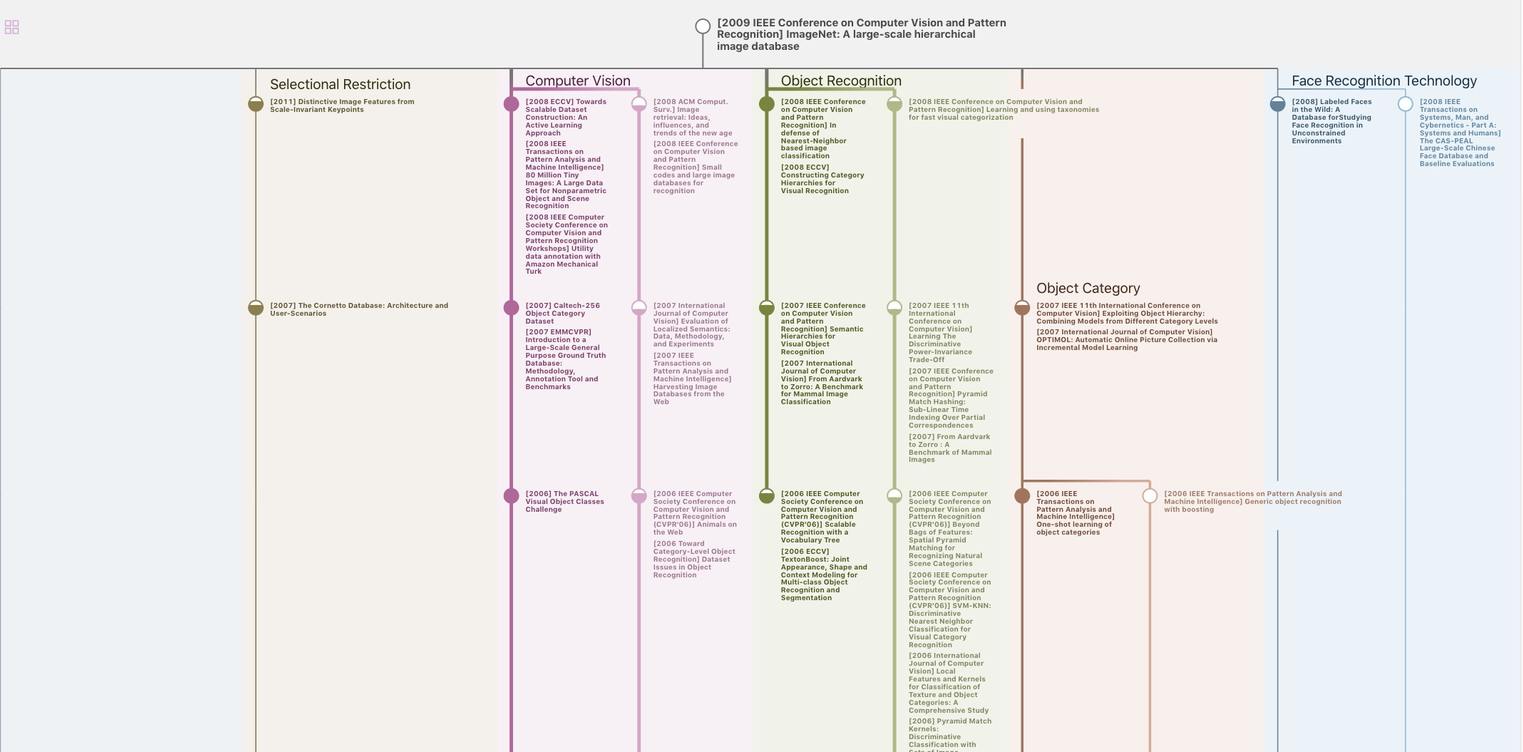
生成溯源树,研究论文发展脉络
Chat Paper
正在生成论文摘要