Learning Local-Global Representation for Scribble-based RGB-D Salient Object Detection Via Transformer
IEEE Transactions on Circuits and Systems for Video Technology(2024)
摘要
Manual scribbles have been introduced to RGB-D Salient Object Detection (SOD) as a credible indicator for salient regions and backgrounds, helping to strike a balance between detection accuracy and labeling efficiency. Previous works address this task by constructing loss functions on semantics, edges, and structures to distinguish salient pixels from the background. However, using local representations extracted by CNNs or Transformers and the incomplete scribble annotations are ineffective in capturing the global contexts of salient objects, and thus cause inaccurate predictions in cluttered regions. In this paper, we propose a local-global representation learning framework by incorporating multi-perception information to boost scribble-based RGB-D SOD. Our system is composed of three sub-modules: Local Representation Aggregation (LRA), Global Representation Initialization (GRI) and Dual Transformer Decoder (DTD). The LRA module first conducts integration of multi-scale, multi-modal local representations extracted from RGB images and depth maps. The GRI module then learns inter- and intra-image representations to capture the global contexts of salient regions from different aspects. Finally, the DTD module alternately updates local-global representations through a dual Transformer architecture. Experimental results on six benchmarks demonstrate that the proposed method performs favorably against state-of-the-art scribble-based RGB-D SOD approaches and is competitive with the fully-supervised approaches.
更多查看译文
关键词
RGB-D Salient Object Detection,Weakly Supervised Learning,Local-Global Representation Learning,Vision Transformer
AI 理解论文
溯源树
样例
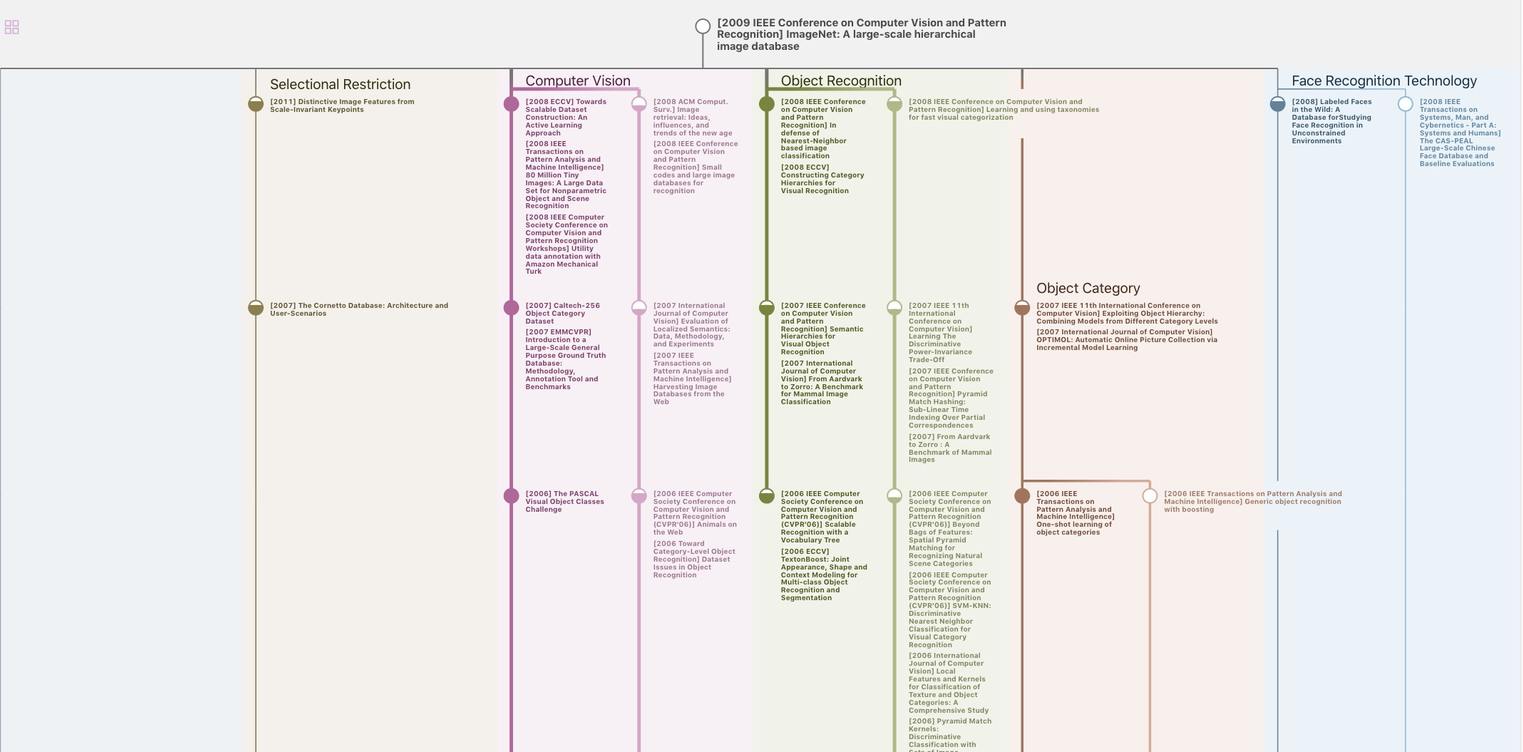
生成溯源树,研究论文发展脉络
Chat Paper
正在生成论文摘要