Learning Theory Convergence Rates for Observers and Controllers in Native Space Embedding.
2023 AMERICAN CONTROL CONFERENCE, ACC(2023)
摘要
This paper derives rates of convergence of approximations of observers and controllers arising in the native space embedding method for adaptive estimation and control of a class of nonlinear ordinary differential equations (ODEs) that feature functional uncertainty. The native space embedding method views the nonlinear ODE as a type of distributed parameter system (DPS), and ideal controllers are derived from the DPS representation. Implementable estimators or controllers for the ODE are obtained by approximation of the DPS using history-dependent, scattered bases in the native space. The basis functions are defined in terms of their centers of approximation. This paper shows that for a large collection of choices of the native space, it is possible to derive convergence rates for implementable schemes that are expressed in terms of the fill distance of the centers of approximation in a subset that supports the observation or measurement process. The error bounds are derived in terms of the power function of the reproducing kernel and resemble those derived recently in machine learning theory and Bayesian estimation as applied to discrete stochastic systems.
更多查看译文
关键词
adaptive control,distributed parameter system,native space
AI 理解论文
溯源树
样例
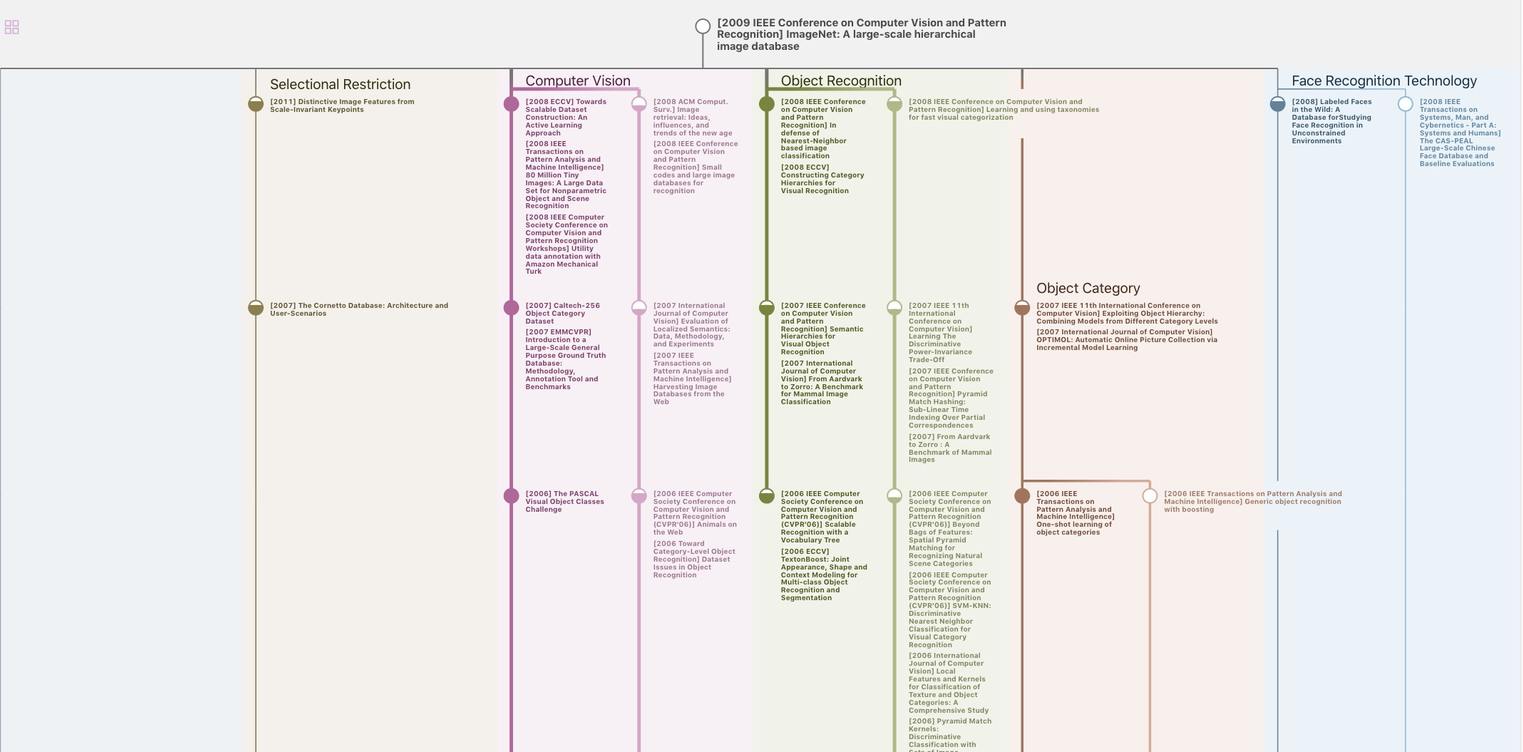
生成溯源树,研究论文发展脉络
Chat Paper
正在生成论文摘要