Hierarchical Prototype-Aligned Graph Neural Network for Cross-Scene Hyperspectral Image Classification
Remote Sens(2024)
摘要
The objective of cross-scene hyperspectral image (HSI) classification is to develop models capable of adapting to the “domain gap” that exists between different scenes, enabling accurate object classification in previously unseen scenes. Many researchers have devised various domain adaptation techniques aimed at aligning the statistical or spectral distributions of data from diverse scenes. However, many previous studies have overlooked the potential benefits of incorporating spatial topological information from hyperspectral imagery, which could provide a more accurate representation of the inherent data structure in HSIs. To overcome this issue, we introduce an innovative approach for cross-scene HSI classification, founded on hierarchical prototype graph alignment. Specifically, this method leverages prototypes as representative embedded representations of all samples within the same class. By employing multiple graph convolution and pooling operations, multi-scale domain alignment is attained. Beyond statistical distribution alignment, we integrate graph matching to effectively reconcile semantic and topological information. Experimental results on several datasets achieve significantly improved accuracy and generalization capabilities for cross-scene HSI classification tasks.
更多查看译文
关键词
cross-scene,hyperspectral image classification,domain adaptation,prototype graph alignment,graph convolution,graph matching
AI 理解论文
溯源树
样例
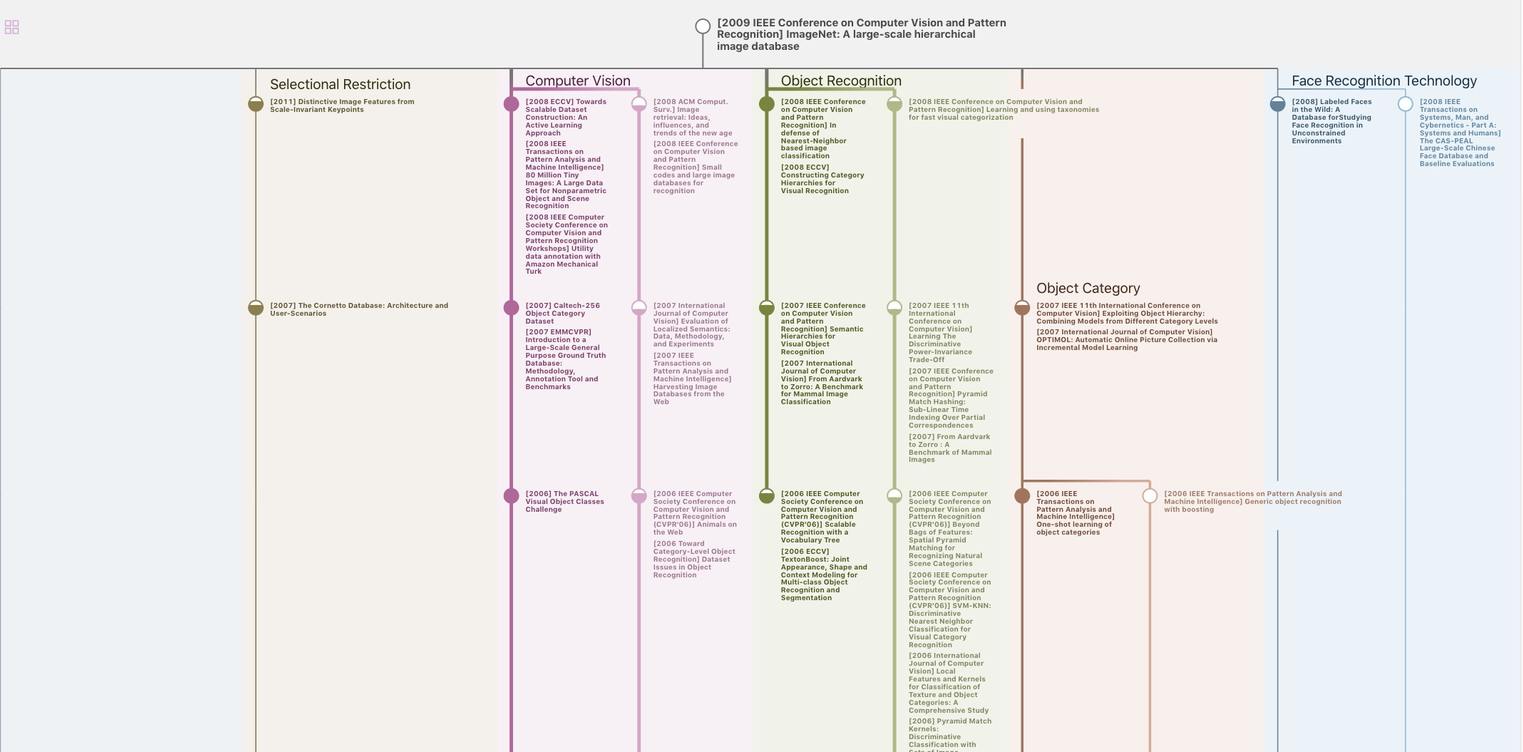
生成溯源树,研究论文发展脉络
Chat Paper
正在生成论文摘要