Deep-Learning-Based View Interpolation Toward Improved TomoSAR Focusing
IEEE GEOSCIENCE AND REMOTE SENSING LETTERS(2024)
摘要
Synthetic aperture radar tomography (TomoSAR) uses several coregistered images from different perspectives to reconstruct a power spectrum pattern (PSP) perpendicular to the line of sight (PLOS), enabling the estimation of a 3-D representation of the area. Classical estimators exhibit ambiguities and other undesired effects that are stronger for sparser and smaller stacks. To mitigate the limitations arising from a restricted number of acquisitions, we propose using a deep neural network (NN) to synthesize artificial tracks (i.e., images not contained in the original stack). The presented method utilizes a convolutional NN with an encoder-decoder architecture. We evaluate the proposed approach on real TomoSAR data from an airborne campaign over a forest region. The view estimation improves the tomographic results, offering robustness to scenarios affected by temporal decorrelation, which other classical methods, such as cubic convolution (CC), do not provide.
更多查看译文
关键词
Artificial neural networks,Training,Tomography,Interpolation,Radar tracking,Focusing,Vectors,Deep learning (DL),interpolation,synthetic aperture radar (SAR),tomography
AI 理解论文
溯源树
样例
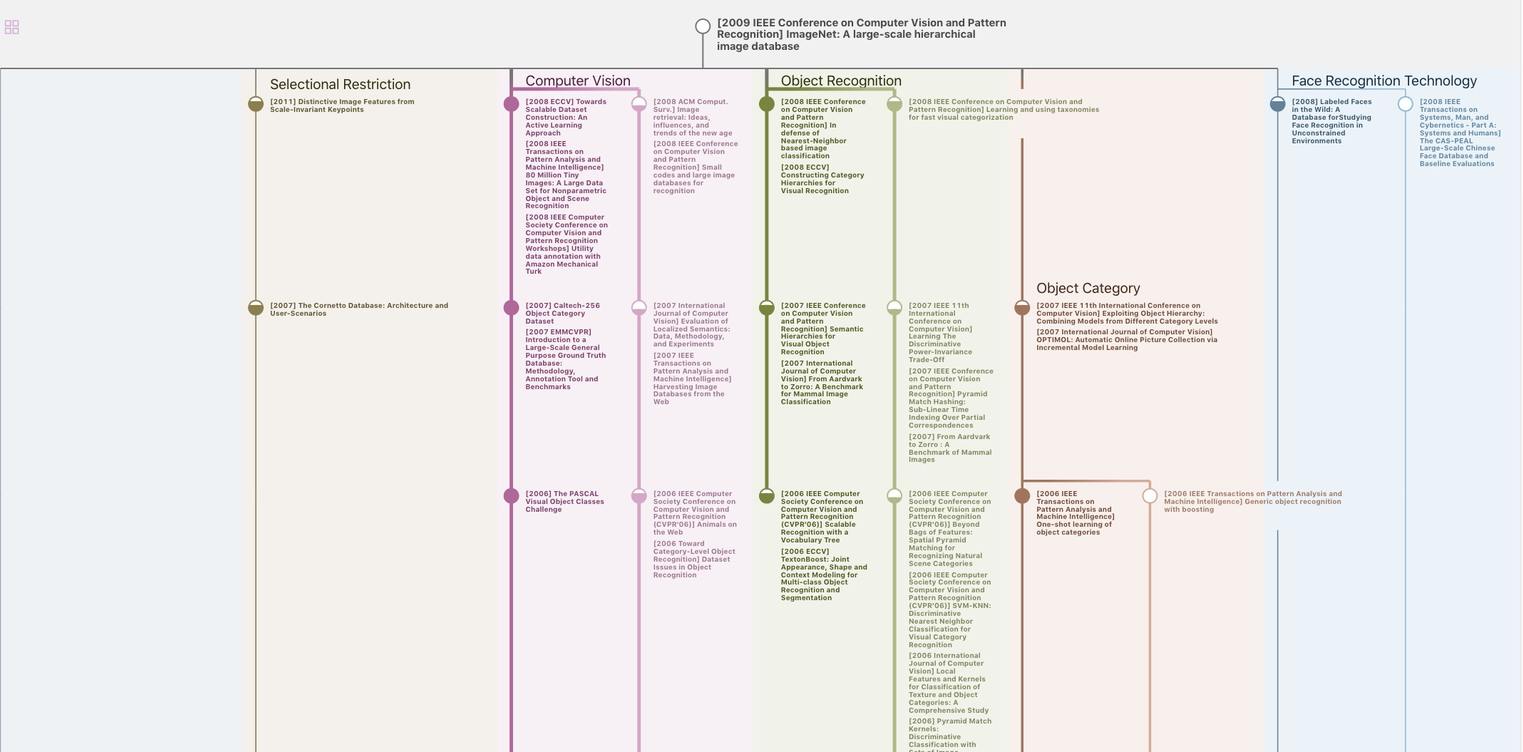
生成溯源树,研究论文发展脉络
Chat Paper
正在生成论文摘要