Defending Learning Systems Against Mean-Shift Perturbations
IEEE Transactions on Artificial Intelligence(2024)
摘要
Evasion attacks on cyber-enabled machine learning (ML) models have recently gained significant traction for their ability to swiftly compel ML models to deviate from their original decisions without substantially affecting model accuracy during the testing phase. In this article, we initially present a meticulously formulated theoretical framework for a novel and potent evasion attack, leveraging mean-shift perturbation. This attack demonstrates remarkable efficiency in deceiving a wide array of ML models. Subsequently, the urgency of fortifying against such evasion attacks is underscored. It’s worth noting that existing defenses are predominantly model-driven, and their efficacy diminishes when concurrently deployed as a universal defense against both poisoning and evasion attacks. Moreover, empirical evidence from various studies suggests that a single defense mechanism falls short in safeguarding learning models against the myriad forms of adversarial attacks. To alleviate these challenges, we introduced Adaptive Ensemble of Filters (AEF), a defense framework characterized by its robust, transferable, model-agnostic, input distribution-independent, and cross-model-supportive nature. The AEF strategically selects filters to safeguard a target ML model from various well-known poisoning (e.g., Metapoison) and evasion (utilizing mean-shift perturbations, JSMA, FGSM, PGD, BIM, and C&W) attacks, establishing itself as a universal defense against diverse adversarial attacks. Theoretical analysis assures the existence of optimal filter ensembles across different input distributions and adversarial attack landscapes, without encountering mode collapses and vanishing gradients. Our claims are substantiated through validation on three publicly available image datasets—MNIST, CIFAR-10, and EuroSAT.
更多查看译文
关键词
Adversarial Attacks,Evasion,Poisoning,Mean-shift Perturbation,Adaptive Filter Ensembles (AEF)
AI 理解论文
溯源树
样例
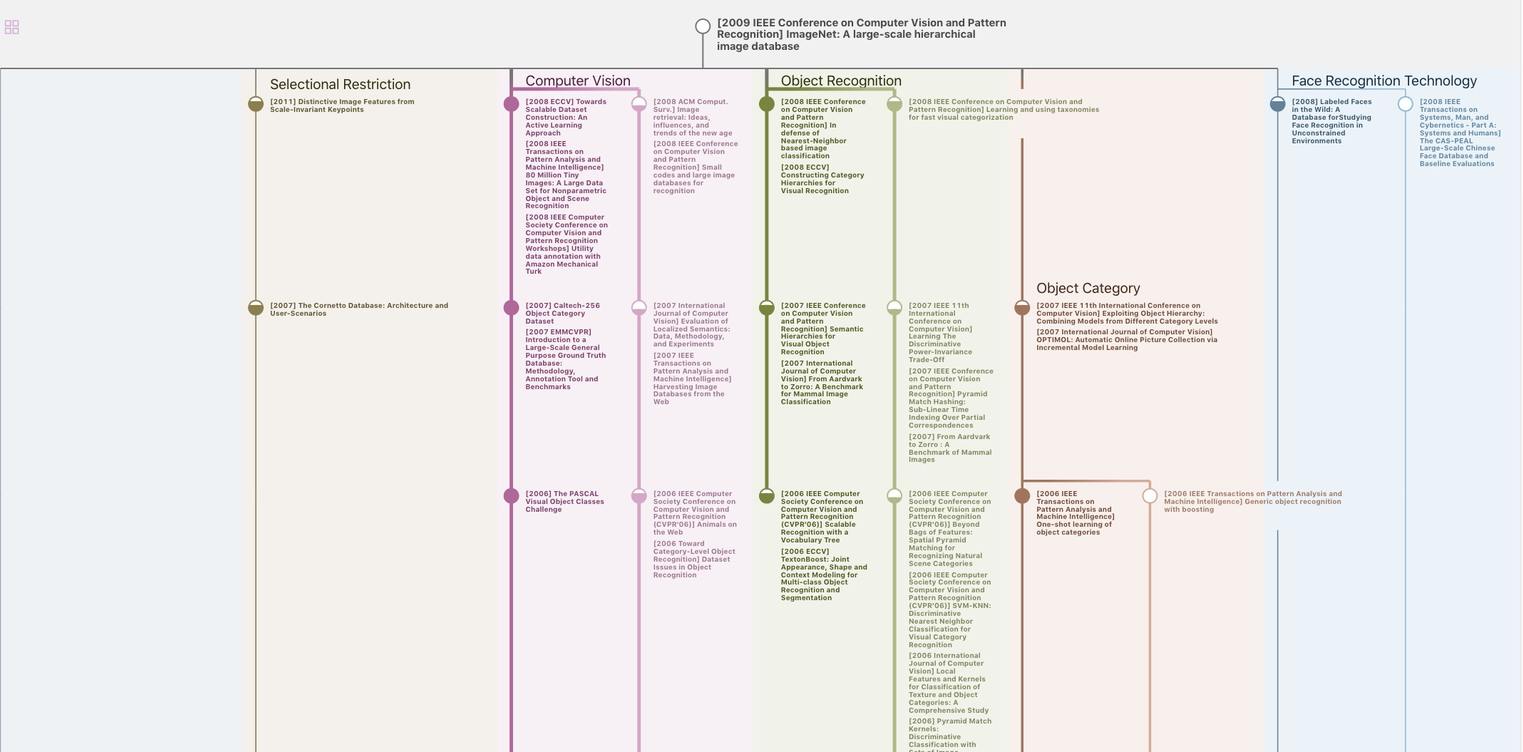
生成溯源树,研究论文发展脉络
Chat Paper
正在生成论文摘要