GNN-Aided Distributed GAN with Partially Observable Social Graph
2024 IEEE Wireless Communications and Networking Conference (WCNC)(2024)
摘要
The proliferation of edge computing has facilitated the edge-based artificial intelligence-generated content (AIGC) for ubiquitous and distributed end devices. To exemplify, we focus on the distributed implementation of one established instance, generative adversarial network (GAN), yielding the distributed GAN task. Practically speaking, this task usually is impeded by concerns including the unknown latency (of processing and transmission), the fairness requirement induced by heterogeneous distributed data and the limited energy budget of end devices. Besides, an often neglected factor is how to exploit feedback from networked end devices among which social ties indicate the flow of shared information. In practice, such social ties are partially observable to lack of exact knowledge of users, e.g., resulted from scarce historical data and privacy issues. Under this setting, we propose an online algorithm via integration of 1) online learning aided by graph neural network (GNN), aiming to recover social ties with GNN-based edge prediction, for accelerated learning of uncertainty and 2) online control to adaptively guarantee the constraints. We theoretically show that it not only achieves a sub-linear regret with guaranteed energy consumption and fairness but also leads to a superior global GAN. We also conduct simulations to justify its outperformance over online baselines.
更多查看译文
关键词
Generative Adversarial Networks,Partial Observation,Social Graph,Energy Consumption,Social Ties,Privacy Issues,Online Learning,Energy Budget,Graph Neural Networks,Online Control,End Devices,Theoretical Analysis,Negative Samples,Global Model,Online Training,Algorithm Design,Nodes In The Graph,Graph Data,Technical Report,Local Dataset,Edge Server,Device Selection,Processing Latency,Local Training,Transmission Latency,Positive Edges,Generative Adversarial Networks Model,Queue Length
AI 理解论文
溯源树
样例
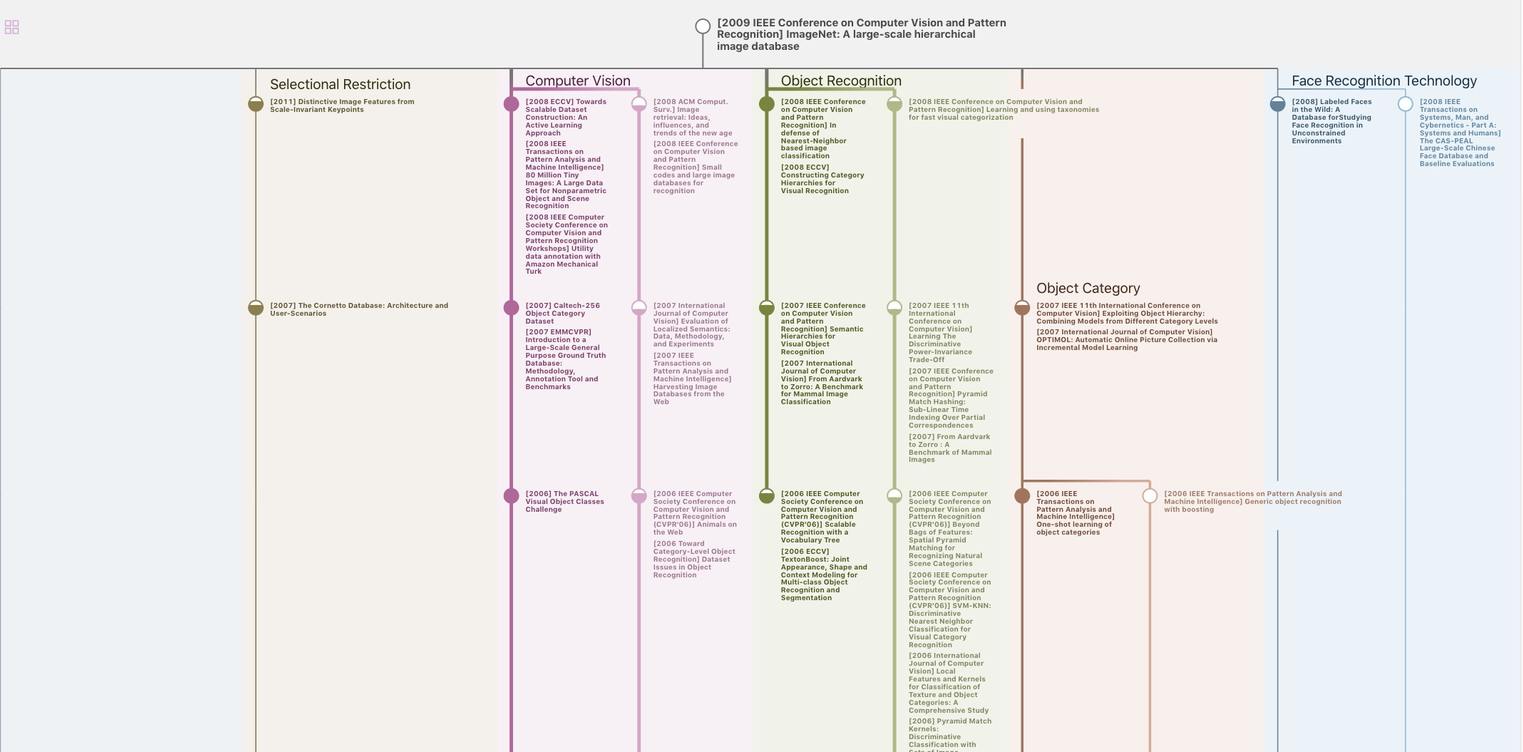
生成溯源树,研究论文发展脉络
Chat Paper
正在生成论文摘要