Improving Denitrification Estimation by Joint Inclusion of Suspended Particles and Chlorophyll a in Aquaculture Ponds
Journal of Environmental Management(2024)
关键词
Denitrification,Suspended particles (SPS),Chlorophyll a (Chla),Estimation model,Aquaculture ponds
AI 理解论文
溯源树
样例
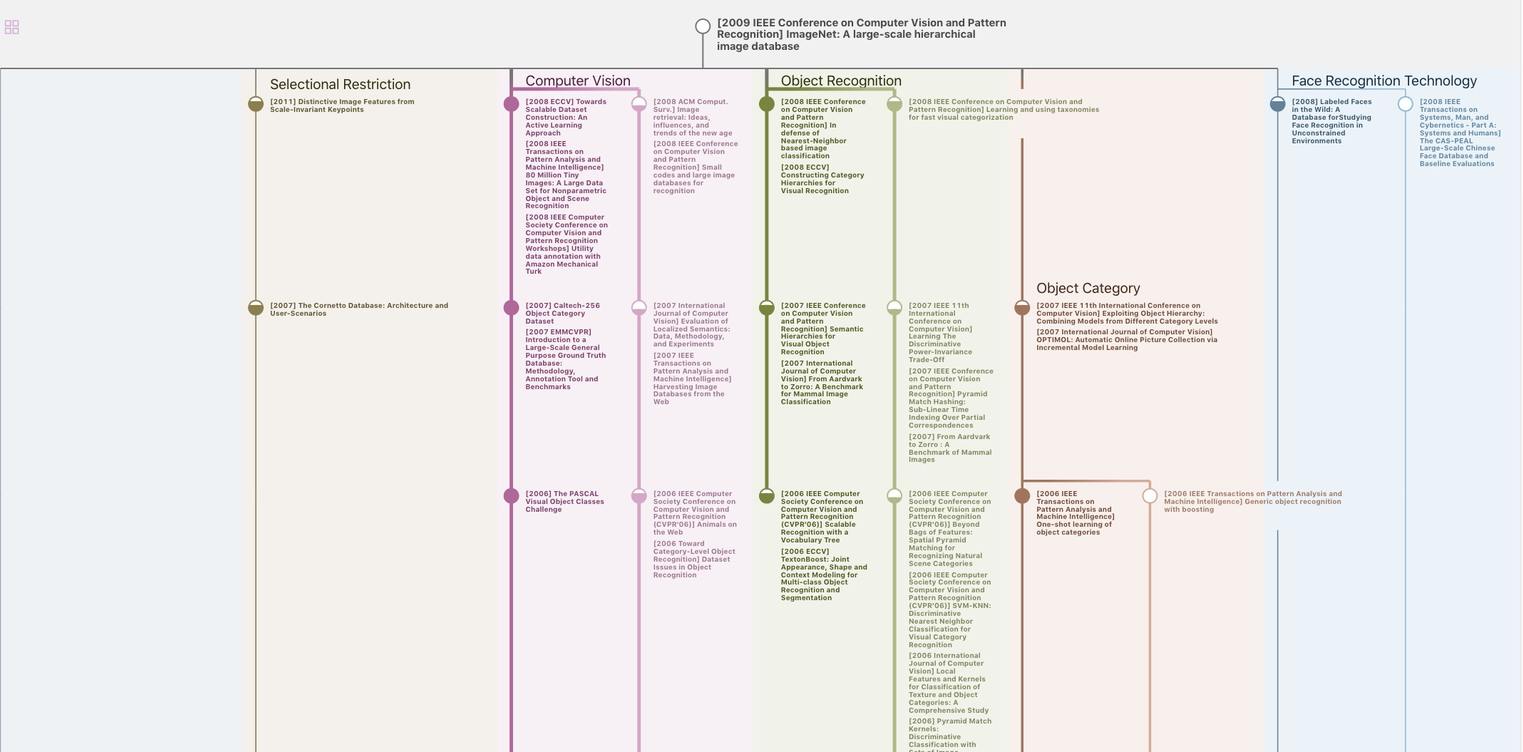
生成溯源树,研究论文发展脉络
Chat Paper
正在生成论文摘要