MetaCirc: A Meta-learning Approach for Statistical Leakage Estimation Improvement in Digital Circuits
2024 IEEE International Symposium on Circuits and Systems (ISCAS)(2024)
摘要
Aggressive scaling down of transistor dimensions has made process-aware circuit modeling a crucial task. Achieving accurate circuit modeling requires lengthy and resource-intensive simulations. Machine Learning-based surrogate models, offering computational efficiency and speed, are viable alternatives to traditional simulators. This paper introduces a meta-learning approach designed to accurately capture process-induced variations in the leakage power of VLSI circuits. The impact of a wide range of fluctuations in operating conditions, including temperature (-55°C to 125°C) and supply voltage (±10%) has also been incorporated for leakage modeling. The proposed meta-learning model is versatile, enhancing the performance of underlying baseline machine-learning models while eliminating the need for time-consuming hyperparameter optimization. Our experiments on leakage estimation using 16 and 7 nanometer FinFET technology nodes demonstrate an average improvement of up to 50% and 48% in Mean Absolute Percentage Error compared to stand-alone baseline models.
更多查看译文
关键词
Meta-learning,Machine Learning,Circuit modeling,Statistical Leakage Analysis,Deep Learning
AI 理解论文
溯源树
样例
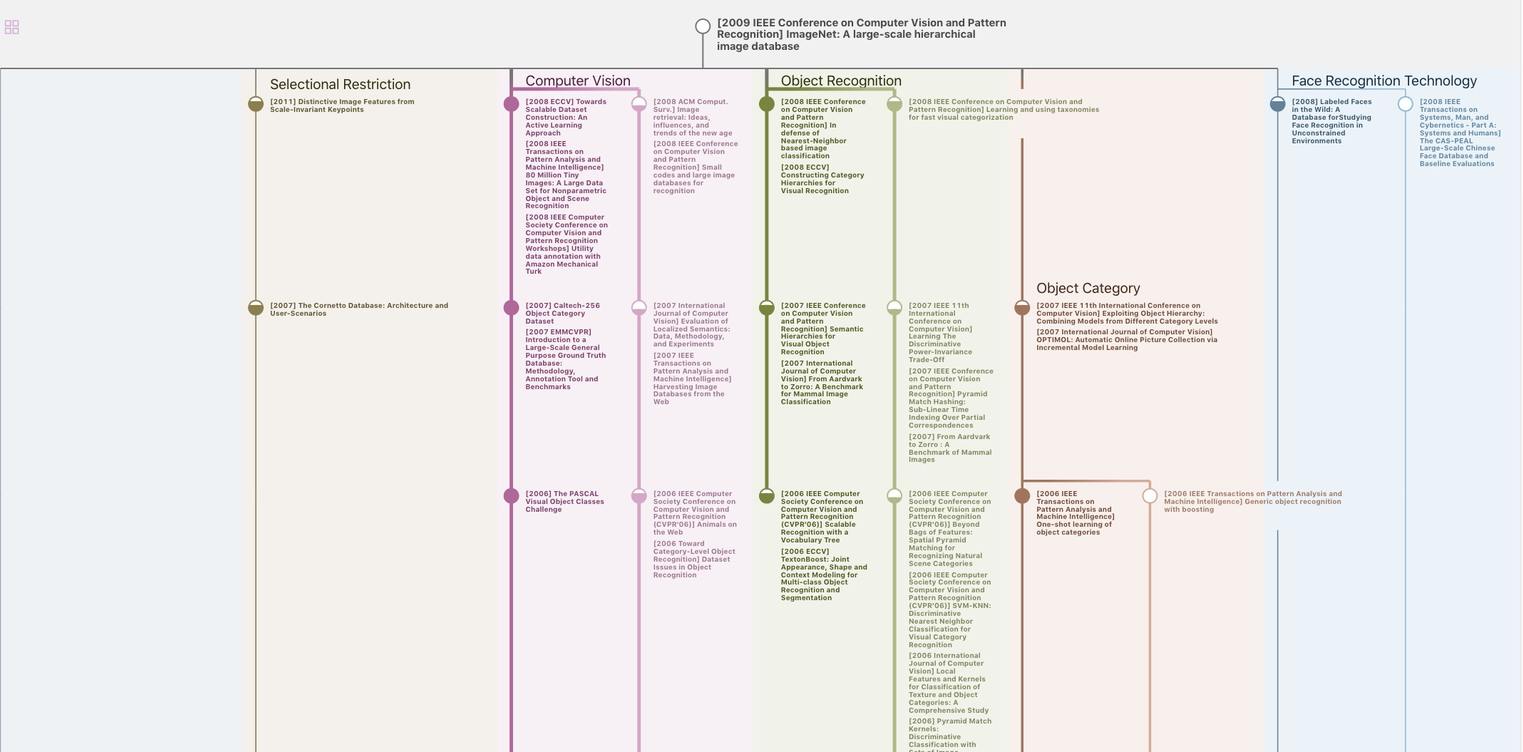
生成溯源树,研究论文发展脉络
Chat Paper
正在生成论文摘要