Spiking Auto-Encoder Using Error Modulated Spike Timing Dependant Plasticity
2024 IEEE International Symposium on Circuits and Systems (ISCAS)(2024)
摘要
Auto-encoders are capable of performing input re-construction through an encoder-decoder structure. These net-works can serve many purposes such as noise removal and anomaly detection, whilst being trained without the need for labelled data. Spiking auto-encoders can utilise asynchronous spikes to potentially improve power and simplify the required hardware. In this work, we propose an efficient spiking auto-encoder with novel error-modulated STDP learning. Our auto-encoder uses the Time To First Spike (TTFS) encoding scheme and needs to update all synaptic weights only once per input. Also, it needs only an average of 8 spikes in its hidden layer for reconstruction, leading to a very sparse and hence potentially power-efficient implementation. We demonstrate decent reconstruction ability for MNIST and the challenging Caltech Face/Motorbike datasets and achieve excellent noise removal from MNIST images.
更多查看译文
关键词
STDP,Spiking Neural Networks,Spiking Auto-encoder
AI 理解论文
溯源树
样例
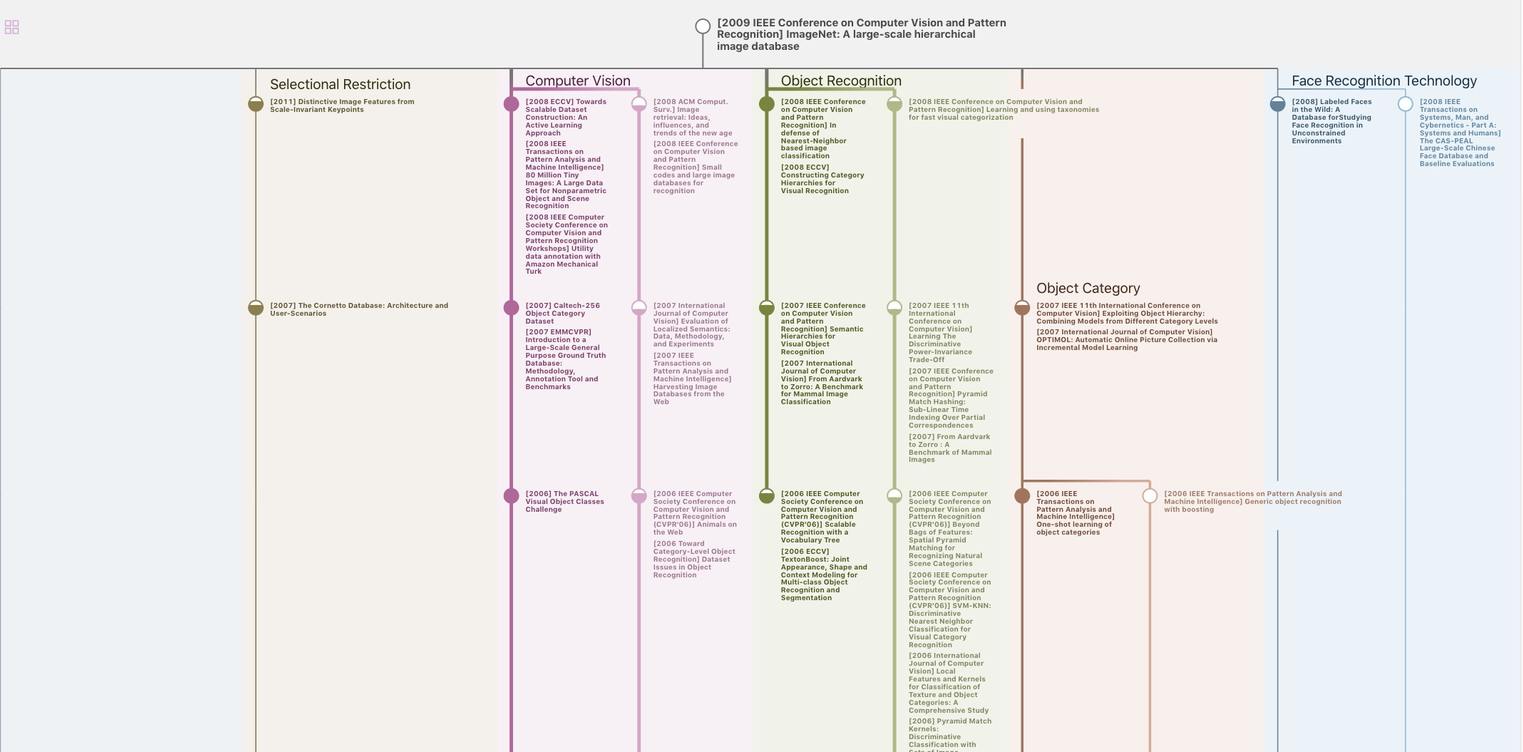
生成溯源树,研究论文发展脉络
Chat Paper
正在生成论文摘要