Uncertainty Estimation in Click-Through Rate Prediction with Deep Neural Gaussian Process
2024 IEEE 6th Advanced Information Management, Communicates, Electronic and Automation Control Conference (IMCEC)(2024)
摘要
Click-through rate (CTR) prediction is a vital task in online advertising, where accurately estimating the probability of a user clicking on an advertisement is crucial for effective campaign management. Uncertainty estimation plays a significant role in CTR prediction by providing insights into the reliability and robustness of the predicted click probabilities. In this paper, we propose a novel approach, Deep Neural Gaussian Process(DNGP), to address uncertainty estimation in CTR prediction. By combining the expressive power of deep neural networks with the probabilistic framework of Gaussian processes, DNGP captures complex nonlinear patterns and provide principled uncertainty estimates. Our experimental results on public dataset and real-world dataset demonstrate that DNGP outperform traditional methods in terms of uncertainty quantification.
更多查看译文
关键词
CTR prediction,uncertainty estimation,gaus-sian process
AI 理解论文
溯源树
样例
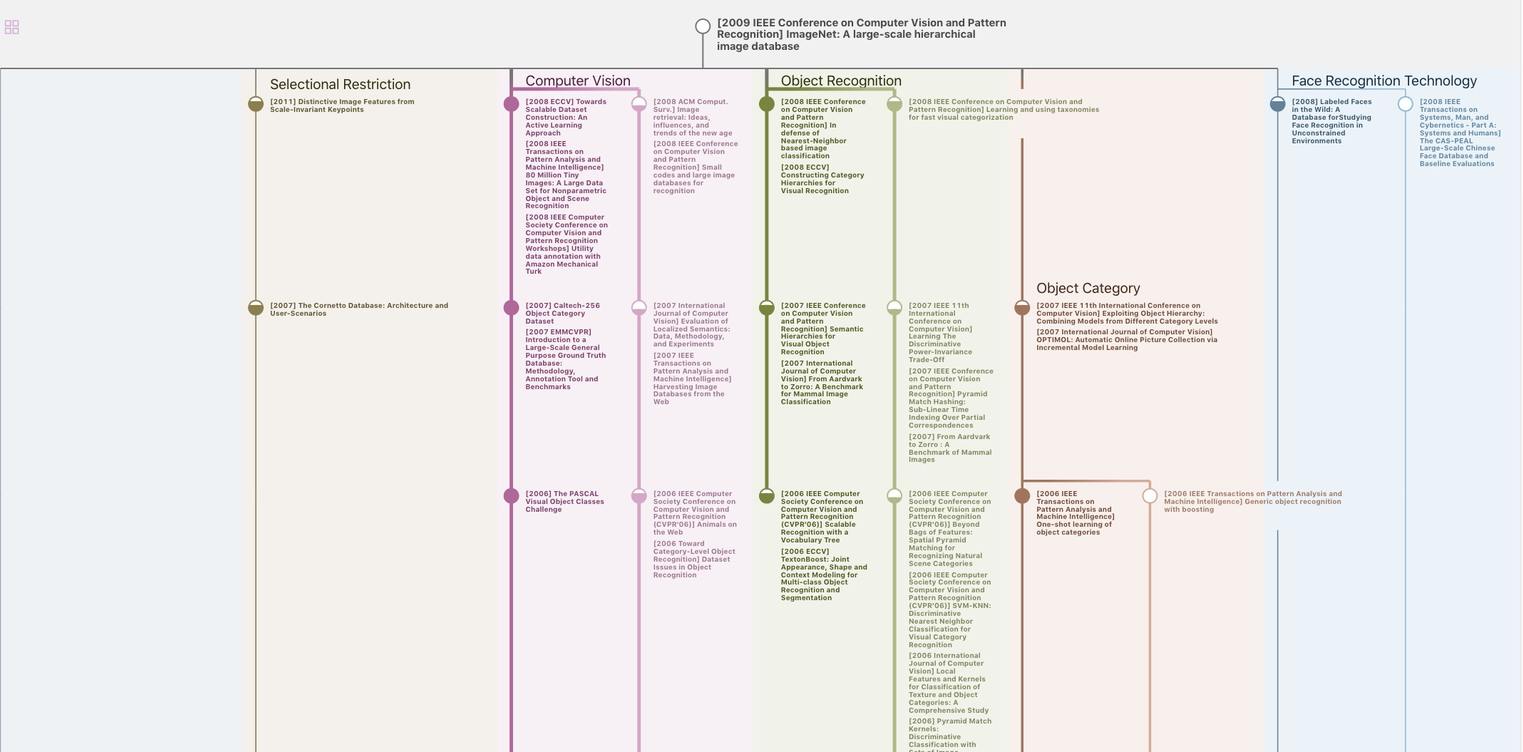
生成溯源树,研究论文发展脉络
Chat Paper
正在生成论文摘要