Intelligent Fault Diagnosis of Rolling Bearing Based on the Depth Feature Fusion Network
IEEE Access(2024)
摘要
An intelligent fault diagnosis method of rolling bearings based on a depth feature fusion network was proposed to solve the problems of a single original signal, large noise interference and difficult diagnosis of varying working conditions of rolling bearings. Using various transform domain signals can make the input contain comprehensive information, extract useful fault features, and conduct feature fusion through the attention mechanism feature fusion method to enhance the ability to obtain effective features. In addition, an improved dense network integrates the pooling layer with dense blocks and introduces a multi-scale convolution kernel and squeeze excitation module. It can promote feature reuse, reduce the dimensions of the feature map, and automatically measure the importance of the weight of each feature channel, enhancing the useful features of the current task and suppressing useless features. Hence, the model has an optimum feature extraction capability. The bearing dataset of Case Western Reserve University was selected to verify the fault diagnosis ability of the proposed method. The recognition accuracy rates under ideal, anti-noise and generalisation tests were 99.75%, 97.81% and 96.88%, respectively. The recognition accuracy rate was higher than that of the other depth learning models. Experiments and comparative analysis showed that the proposed method has good anti-noise and generalisation abilities compared to traditional methods.
更多查看译文
关键词
rolling bearing,intelligent fault diagnosis,depth feature fusion,transform domain signal,improved dense network,attention mechanism feature fusion method
AI 理解论文
溯源树
样例
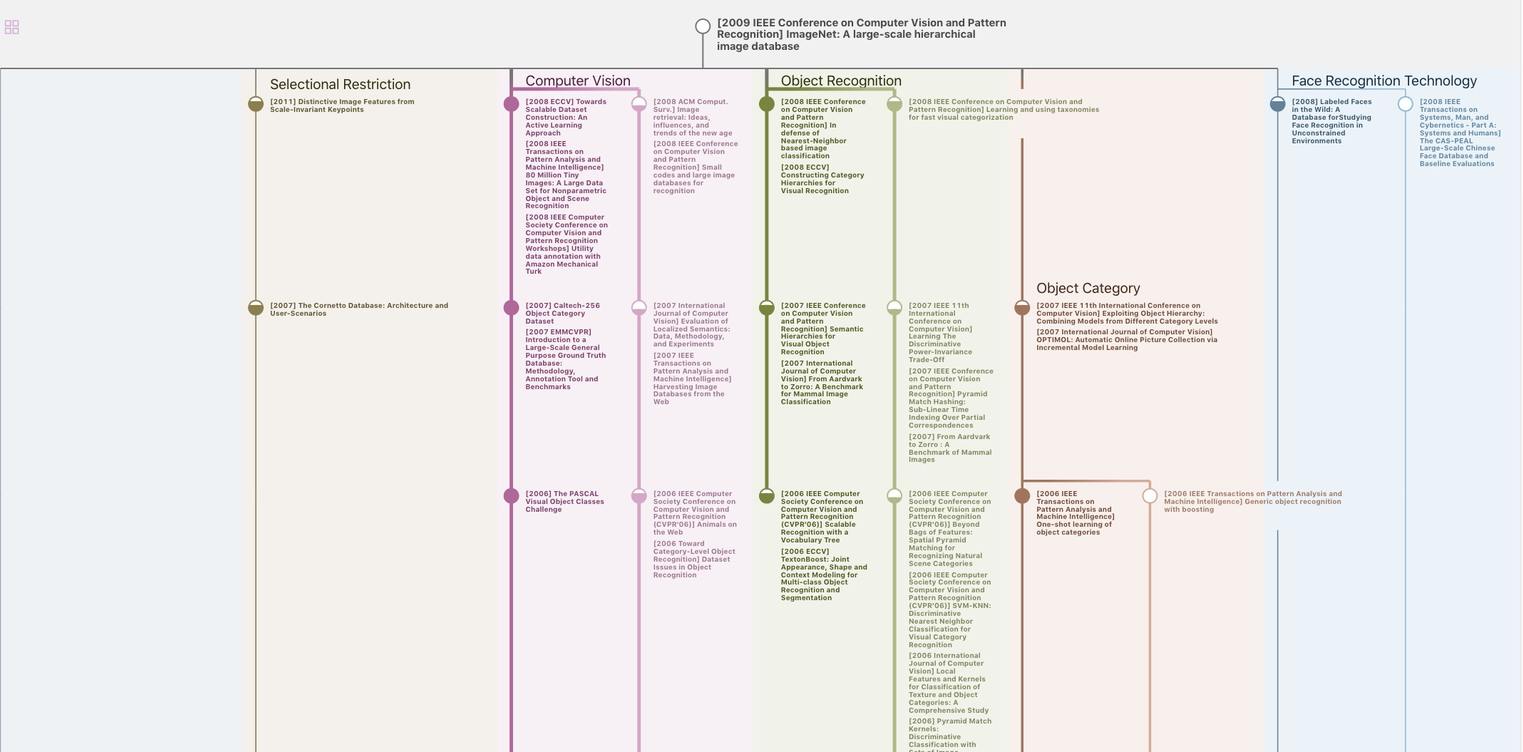
生成溯源树,研究论文发展脉络
Chat Paper
正在生成论文摘要