Exploiting Wavelet Scattering Transform & 1D-CNN for Unmanned Aerial Vehicle Detection
IEEE Signal Processing Letters(2024)
摘要
Recent advancements in Unmanned Aerial Vehicles (UAVs) have prompted concerns regarding their potential misuse. Deep-learning techniques offer superior detection capabilities compared to traditional rule-based approaches, provided the training dataset is diverse and sufficiently large. We present a UAV acoustic dataset featuring a range of UAVs, from toys to high-speed models, recorded in an open field simulating airport environments. To ensure robust multi-conditional training, the dataset is augmented with noise at specific signal-to-noise ratios (SNRs). Further, we introduce a network called WST-CNN, which has a non-trainable Wavelet Scattering Transform (WST) layer with fixed initializations as the first layer in a Convolutional Neural Network (CNN). With raw audio as an input, exempting any pre-processing, WST provides a multi-resolution time-frequency representation resilient to noise and signal deformation. As a secondary result, we introduce a 1D-F-CNN network utilizing distinctive acoustic features for UAV detection.
更多查看译文
关键词
Unmanned aerial vehicles,dataset,end-to-end,wavelet scattering transform,convolutional neural network
AI 理解论文
溯源树
样例
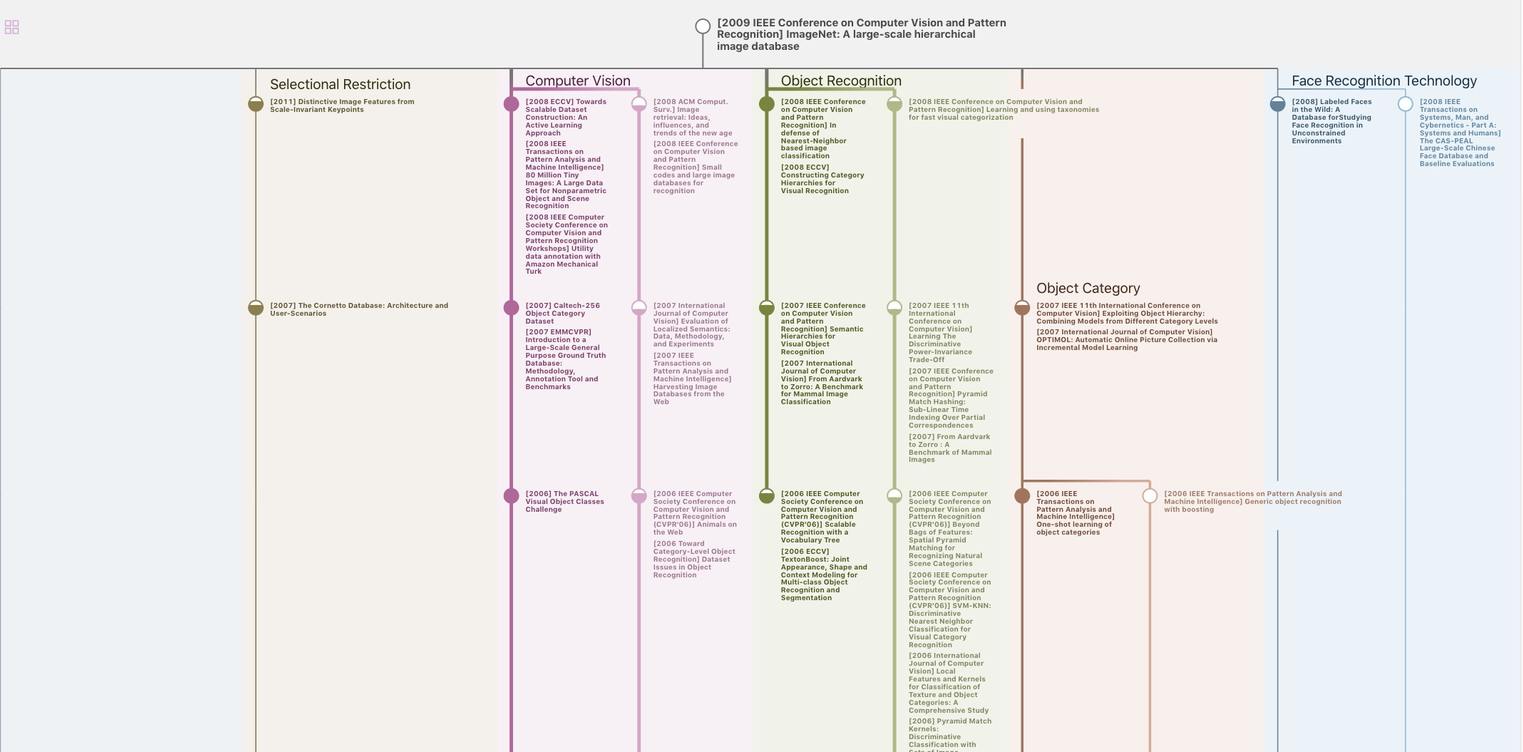
生成溯源树,研究论文发展脉络
Chat Paper
正在生成论文摘要