Vision-Based Real-Time Shape Estimation of Self-Occluding Soft Parallel Robots Using Neural Networks
IEEE Robotics and Automation Letters(2024)
摘要
Vision-based shape estimation is promising to enhance applications of soft robots due to its versatility, cost-effectiveness, and simple calibration. However, most prevailing vision-based shape estimation approaches are inappropriate for soft robots with complex 3-D structures mainly because of self-occlusion and closed-loop kinematic chains. In this work, we propose a learning-based shape estimation approach for a custom-built soft parallel robot with the self-occlusion condition. In this sense, we design a conventional neural network (CNN) to map external visual images to robot shapes parameterized by B-spline curves. To train the designed CNN, we create a dataset comprising pairs of multi-view images and corresponding shape curves. Image augmentation is applied to enhance the robustness of the CNN, and a customized centerline extraction algorithm calculates shape curves. Moreover, an efficient redeployment method of the trained CNN is developed based on deep transfer learning. The experimental results demonstrate that the proposed approach shows high precision (2.91 mm error at the end effector), computational efficiency (24.44 Hz), and robustness to uncertain observation conditions. Further, the developed redeployment method contributes to a 40 % reduction in training data and a 75 % reduction in training time.
更多查看译文
关键词
Soft robotics,visual learning,shape estimation,deep learning methods
AI 理解论文
溯源树
样例
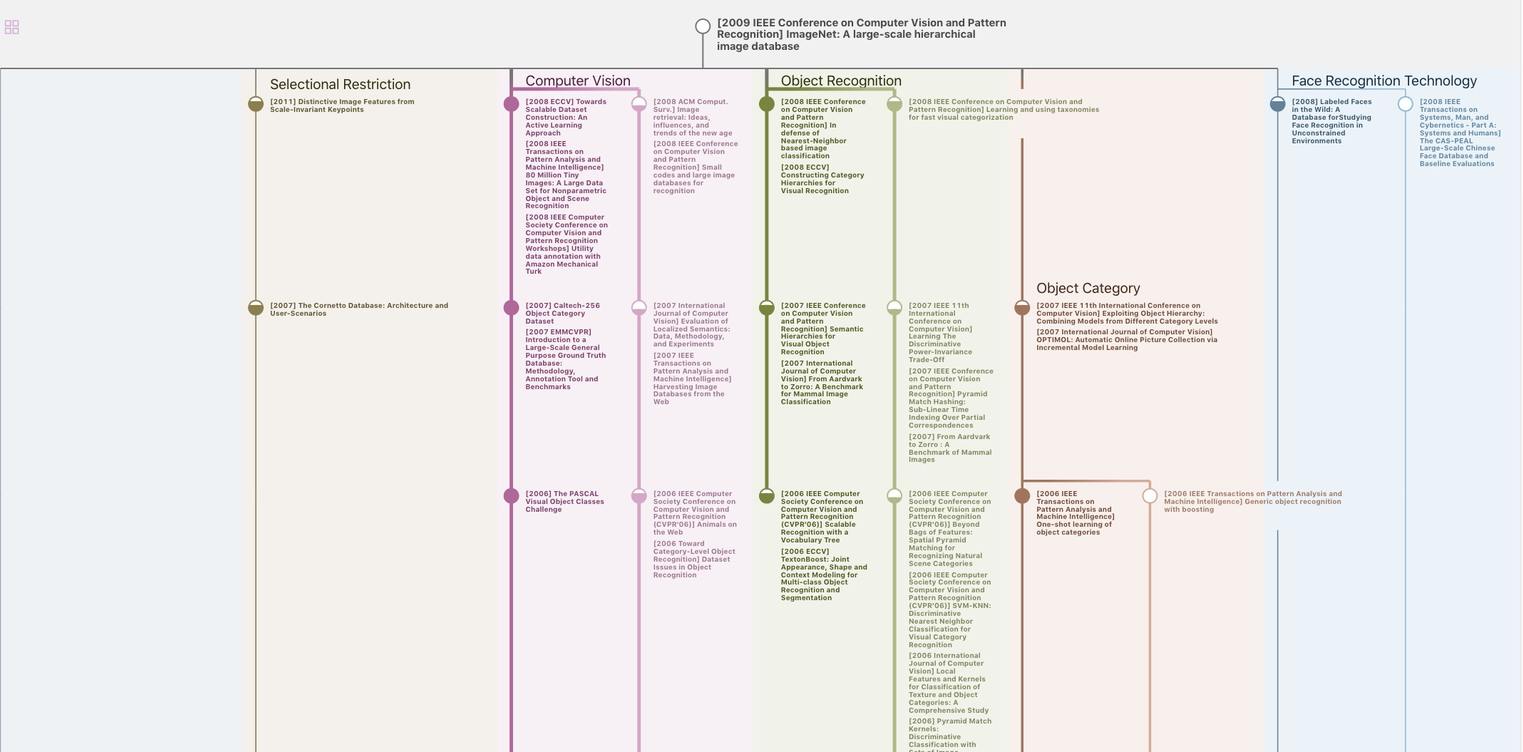
生成溯源树,研究论文发展脉络
Chat Paper
正在生成论文摘要