Exploring Parameter Dependence of Atomic Minima with Implicit Differentiation
arxiv(2024)
摘要
Interatomic potentials are essential to go beyond ab initio size limitations, but simulation results depend sensitively on potential parameters. Forward propagation of parameter variation is key for uncertainty quantification, whilst backpropagation has found application for emerging inverse problems such as fine-tuning or targeted design. Here, the implicit derivative of functions defined as a fixed point is used to Taylor expand the energy and structure of atomic minima in potential parameters, evaluating terms via automatic differentiation, dense linear algebra or a novel sparse operator approach. The latter allows efficient forward and backpropagation through relaxed structures of arbitrarily large systems. The implicit expansion accurately predicts lattice distortion and defect formation energies and volumes with classical and machine-learning potentials, enabling high-dimensional uncertainty propagation without prohibitive overhead. We then show how the implicit derivative can be used to solve challenging inverse problems, minimizing an implicit loss to fine-tune potentials and stabilize solute-induced structural rearrangements at dislocations in tungsten.
更多查看译文
AI 理解论文
溯源树
样例
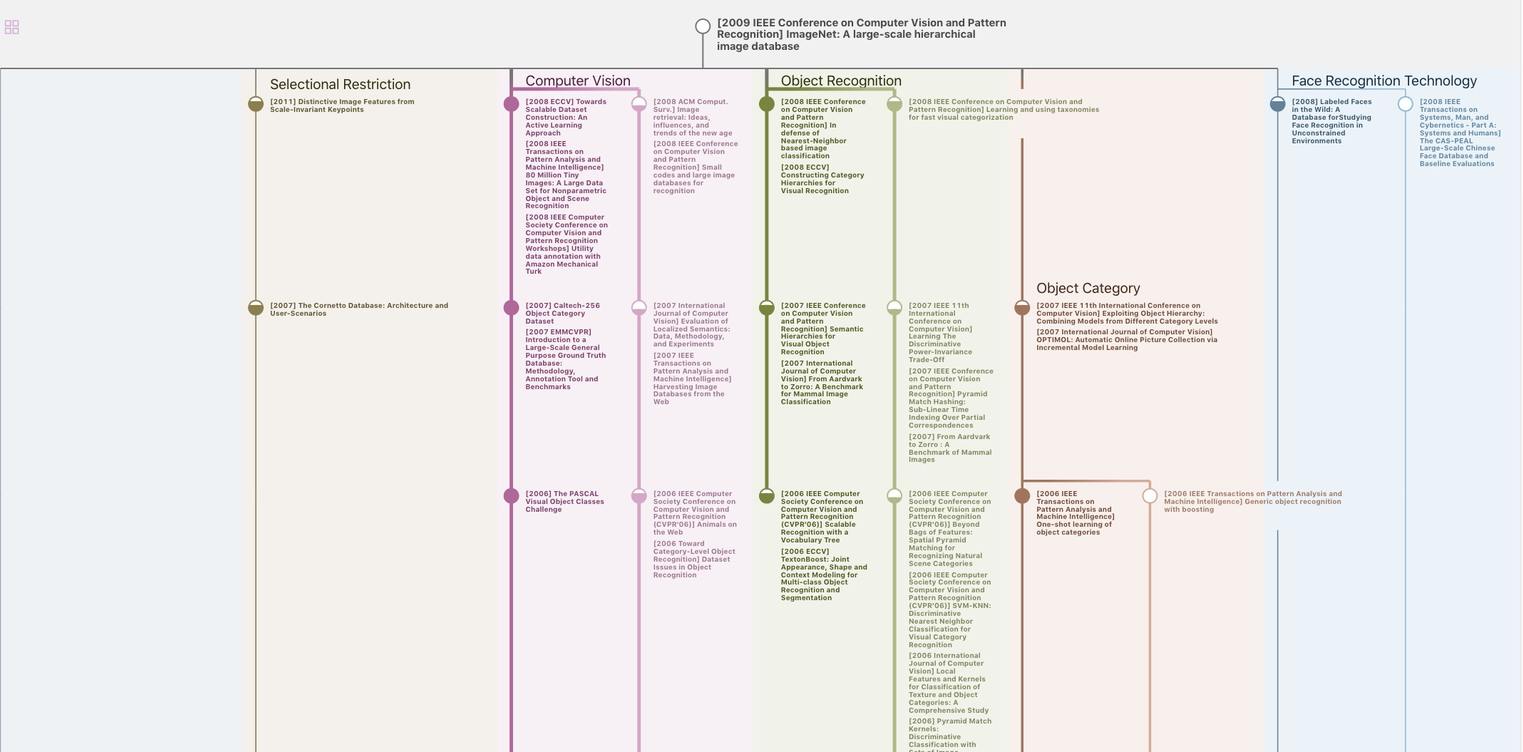
生成溯源树,研究论文发展脉络
Chat Paper
正在生成论文摘要