Untangling the Unrestricted Web: Automatic Identification of Multilingual Registers
arxiv(2024)
摘要
This article explores deep learning models for the automatic identification of registers - text varieties such as news reports and discussion forums - in web-based datasets across 16 languages. Web register (or genre) identification would provide a robust solution for understanding the content of web-scale datasets, which have become crucial in computational linguistics. Despite recent advances, the potential of register classifiers on the noisy web remains largely unexplored, particularly in multilingual settings and when targeting the entire unrestricted web. We experiment with a range of deep learning models using the new Multilingual CORE corpora, which includes 16 languages annotated using a detailed, hierarchical taxonomy of 25 registers designed to cover the entire unrestricted web. Our models achieve state-of-the-art results, showing that a detailed taxonomy in a hierarchical multi-label setting can yield competitive classification performance. However, all models hit a glass ceiling at approximately 80 web registers and the inherent uncertainty in labeling some documents. By pruning ambiguous examples, we improve model performance to over 90 multilingual models outperform monolingual ones, particularly benefiting languages with fewer training examples and smaller registers. Although a zero-shot setting decreases performance by an average of 7 not linked to specific registers or languages. Instead, registers show surprising similarity across languages.
更多查看译文
AI 理解论文
溯源树
样例
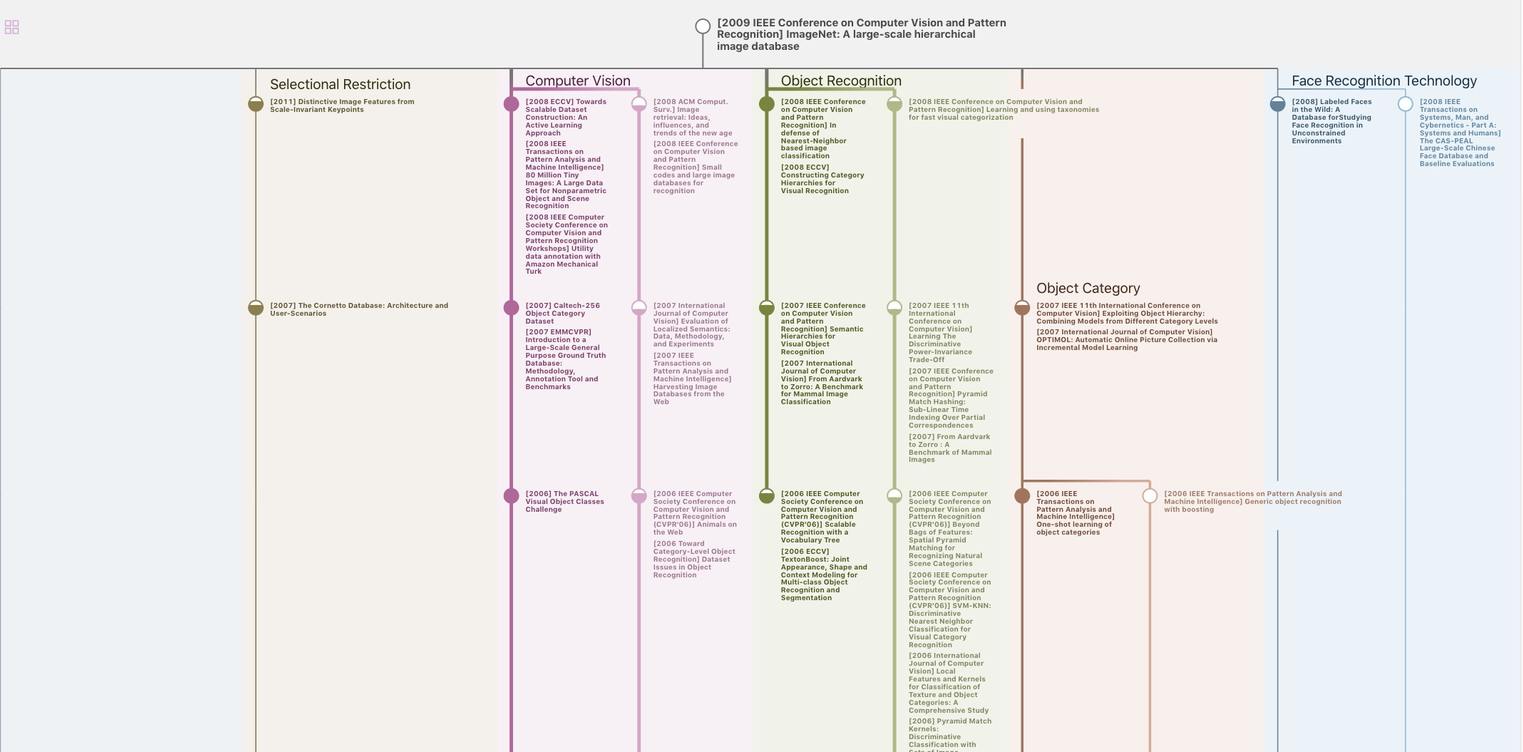
生成溯源树,研究论文发展脉络
Chat Paper
正在生成论文摘要