A High-Accurate H2S Recognition Method Based on Pearson Correlation Coefficient Feature Extraction of Dynamic Response
IEEE Sensors Journal(2024)
摘要
Metal oxide sensors have traditionally relied on constant temperature heating, which solely captures the changes in sensor resistance and ignores signals originating from processes like surface adsorption, redox reactions, and multi-gas competition. In this study, a high-accurate H 2 S recognition method based on Pearson correlation coefficient feature extraction of dynamic response was proposed. 10 feature parameters that varied significantly with species and concentration of gases were extracted and feature vectors using the Pearson correlation coefficient method were designed to analyze the dynamic response under three different square wave heating modes. Furthermore, the effectiveness of the features extracted from the dynamic response in recognizing single gases by KNN, SVM, and ANN was studied, the application of ANN in predicting single gas concentration was investigated, and the results demonstrated that the accuracy of single gas recognition can reach 99.99%, and the minimum error of H 2 S concentration is less than 3 ppm. The application of BPNN in predicting the composition and concentration of two-component gas mixtures was further explored, and the accuracy of H 2 S recognition can reach 99%, with an error of less than 1 ppm in H 2 S concentration. Additionally, the trained ANN was successfully deployed on mobile terminals through the lightweight TensorFlow Lite framework to achieve high-accurate recognition and concentration prediction for H 2 S gas.
更多查看译文
关键词
Dynamic response,Pearson correlation coefficient,feature extraction,machine learning,mobile terminal algorithms
AI 理解论文
溯源树
样例
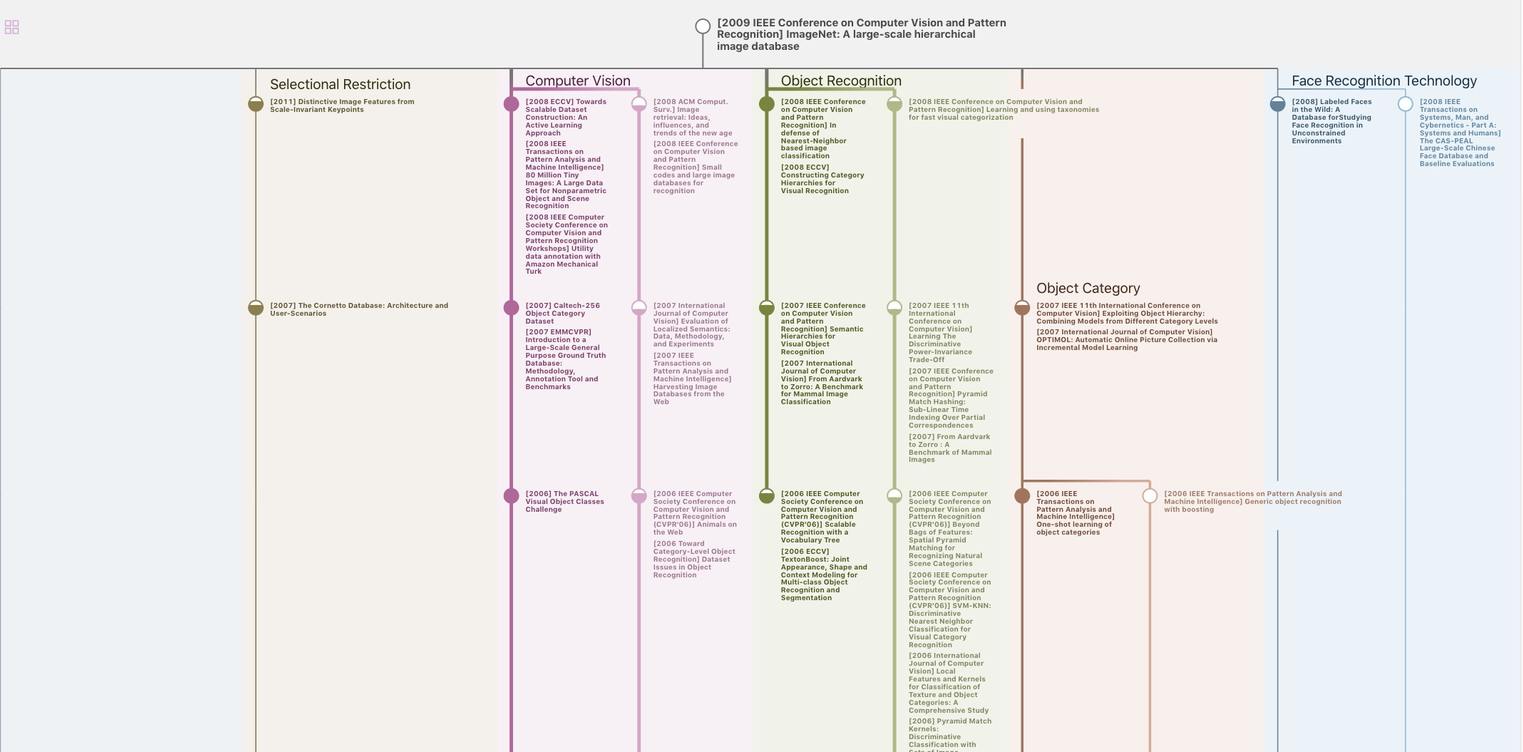
生成溯源树,研究论文发展脉络
Chat Paper
正在生成论文摘要