Real-time Unsupervised Monitoring of Earth Pressure Balance Shield-Induced Sinkholes in Mixed-Face Ground Conditions Via Convolutional Variational Autoencoders
Tunnelling and underground space technology(2024)
摘要
This study introduces a real-time unsupervised monitoring framework for monitoring sinkhole formation events during earth pressure balance (EPB) shield tunneling operations. A feature extractor (FE) is constructed by coupling variational Autoencoders structure with convolutional neural network layers (VAE-CNN) to manage the complexity of EPB operational data, including non-linearity and temporal dependencies. The monitoring framework consists of two main phases: offline modeling and online monitoring. In the offline modeling phase, an FE model is trained using data-intensive techniques to define a subspace characterizing the behavior of multivariate data without sinkhole formations. The squared prediction error (SPE) statistics and the control limits are computed for detection. During the online monitoring phase, unseen EPB data is propagated to generate SPE values and determine sinkhole events based on whether these values surpass the control limit. Sensor validity index violation counts were used to isolate the most influential variables, while the results demonstrated the superiority of the proposed VAE-CNN method, achieving a 100% detection rate and a 0.9% false alarm rate. The influential variables identified include cutter resolutions per minute, jack speed, screw pressure, torque, and cutter seal components. The monitoring system shows great potential for early warnings during EPB operations to mitigate sinkhole formation risks.
更多查看译文
关键词
Autoencoders,Sinkhole formation,Earth pressure balance,Shield tunneling,Tunnel construction,Unsupervised monitoring
AI 理解论文
溯源树
样例
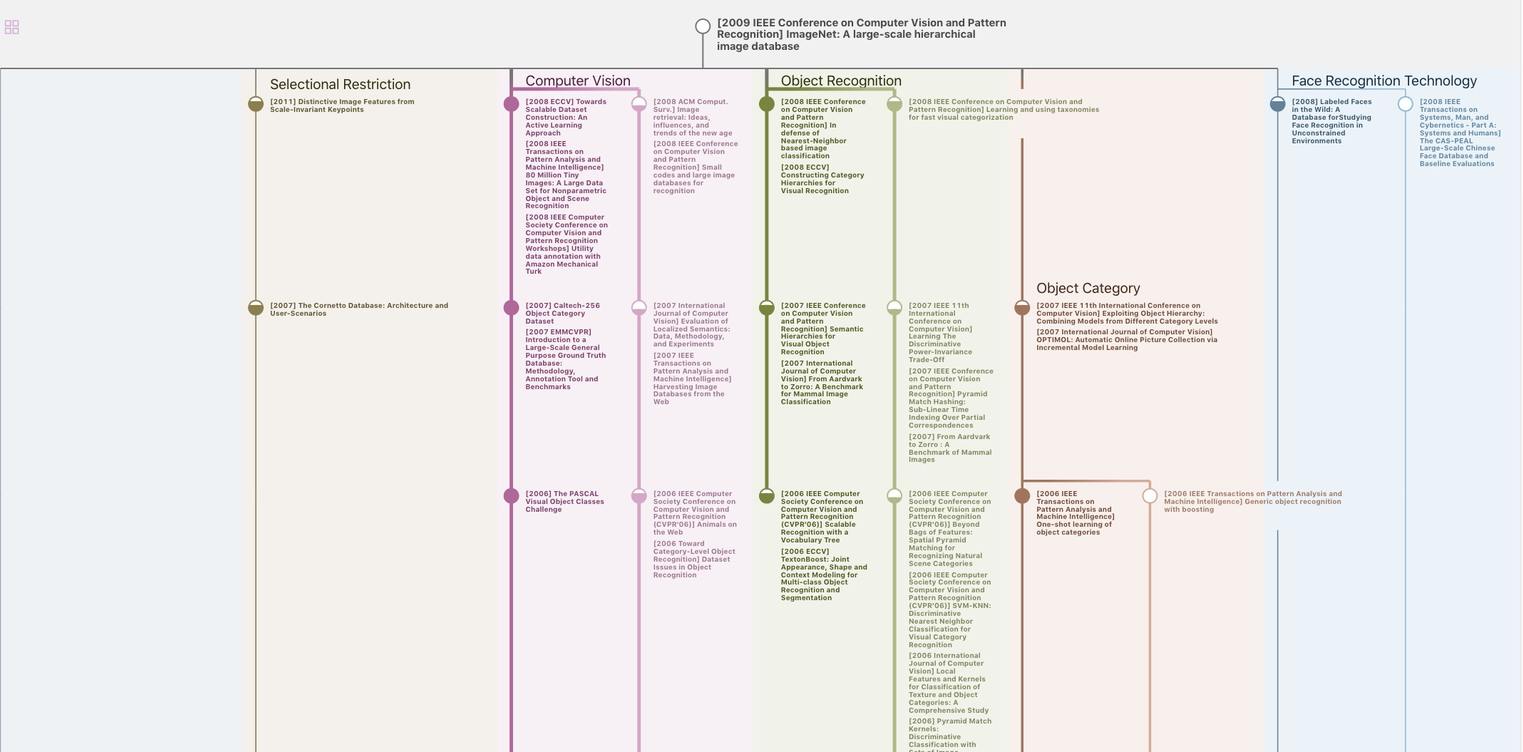
生成溯源树,研究论文发展脉络
Chat Paper
正在生成论文摘要