Forecasting of Stock Volatility Using Deep Learning Model with Likelihood-Based Loss Function
2023 IEEE Fifth International Conference on Advances in Electronics, Computers and Communications (ICAECC)(2023)
摘要
Predicting the volatility of financial assets can be helpful, and volatility is employed in a variety of financial contexts. Volatility in the stock sector is a widely used indicator of overall market risk. Time-series anticipating in the economic sector is exceedingly difficult because of the intricacy and fluctuation of financial fields. In this paper, we anticipate stock index volatility using a deep-neural-network (DNN) and a long-short-term-memory (LSTM) model. Distance loss function is widely used in related research to train machine-learning (ML) strategies, although this has two drawbacks. The initial is that their designs cannot be reasonably contrasted to econometric ones, and the second is that they create mistakes when utilizing predicted volatility as the estimated aim. We also incorporate a probability-based loss-function (LF) to train the deep-learning (DL) approaches and evaluate the models based on the likelihood of the test sample, which allows us to address the aforementioned two issues. In comparison to the econometric and the DL strategies with distance LF, our results show that the volatility can be predicted more accurately by the LSTM model in the two DL approaches with likelihood-based LF.
更多查看译文
关键词
Deep learning,LSTM,DNN,Time series,Forecasting,Volatility
AI 理解论文
溯源树
样例
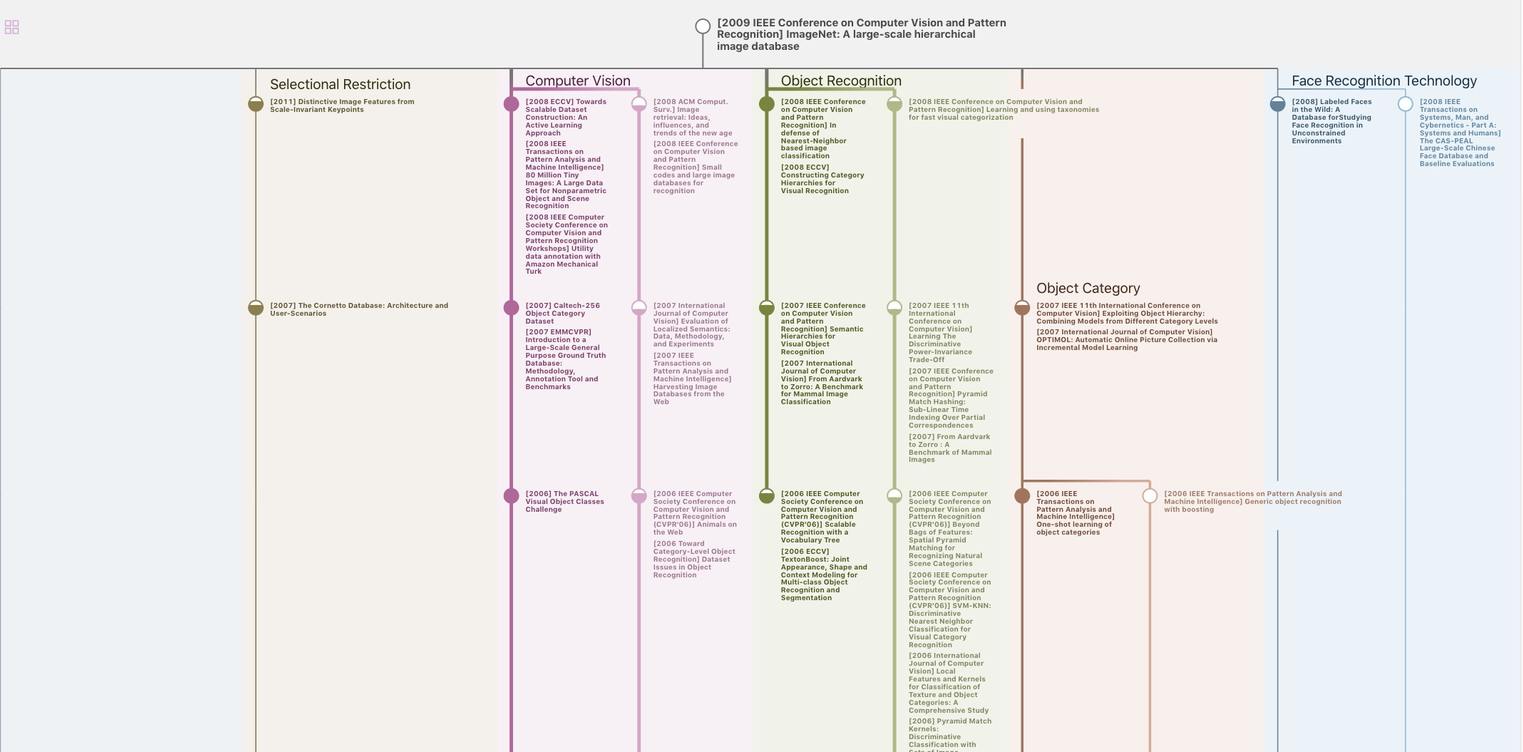
生成溯源树,研究论文发展脉络
Chat Paper
正在生成论文摘要