Semantic Information Collaboration Network for Semantic Change Detection in Remote Sensing Images
IEEE journal of selected topics in applied earth observations and remote sensing(2024)
摘要
Semantic change detection (SCD) extends the traditional change detection (CD) task to simultaneously identify the change areas and their corresponding land cover categories in bi-temporal images. This “from-to” change information holds significant value in numerous practical applications and is increasingly garnering attention in the remote sensing domain. However, prevalent challenges such as loss of detail and class imbalance significantly hinder the efficacy of SCD applications. To address this challenge, we propose a novel semantic information collaboration network (SIC-Net). This network incorporates a detail capture path and a spatial-temporal semantic coordination module aiming to effectively execute SCD by fusing detailed information with contextual features and harnessing synergies between binary CD and semantic segmentation. Additionally, we introduce a pseudo-label growth algorithm to mitigate the substantial loss of sample category information. The experimental results on two widely used SCD datasets demonstrate that SIC-Net outperforms other methods across various evaluation metrics, achieving SeK of 23.96% and 61.29%, respectively. These findings not only validate the effectiveness of the SIC-Net but also provide new ideas and directions for future research in the field of remote sensing, particularly in SCD.
更多查看译文
关键词
Semantics,Task analysis,Feature extraction,Remote sensing,Semantic segmentation,Land surface,Accuracy,Change detection,pseudo-label growth algorithm (PLGA),semantic change detection (SCD),semantic coordination,semantic segmentation,spatial detail
AI 理解论文
溯源树
样例
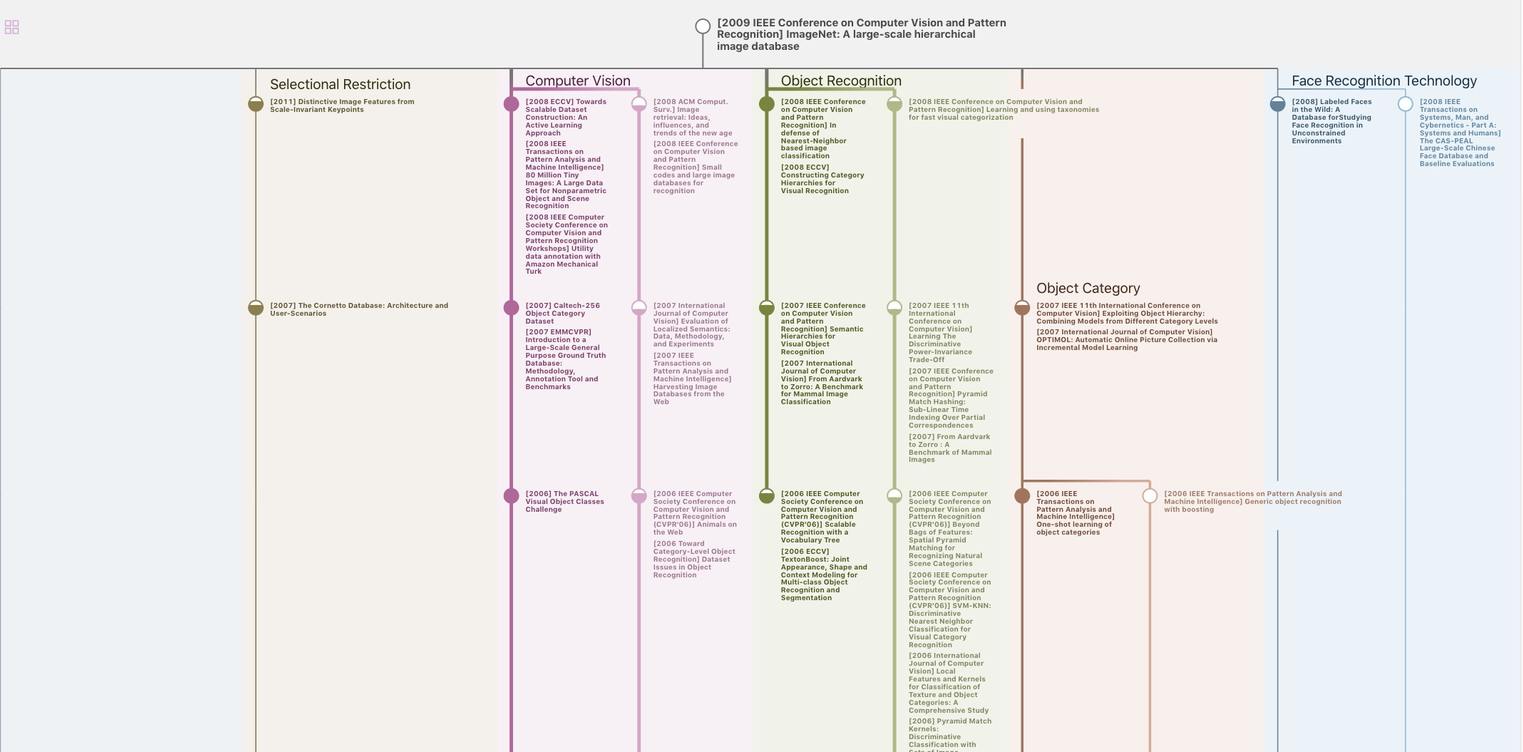
生成溯源树,研究论文发展脉络
Chat Paper
正在生成论文摘要