Co-Exploring Structured Sparsification and Low-Rank Tensor Decomposition for Compact DNNs
IEEE TRANSACTIONS ON NEURAL NETWORKS AND LEARNING SYSTEMS(2024)
摘要
Sparsification and low-rank decomposition are two important techniques to compress deep neural network (DNN) models. To date, these two popular yet distinct approaches are typically used in separate ways; while their efficient integration for better compression performance is little explored, especially for structured sparsification and decomposition. In this article, we perform systematic co-exploration on structured sparsification and decomposition toward compact DNN models. We first investigate and analyze several important design factors for joint structured sparsification and decomposition, including operational sequence, decomposition format, and optimization procedure. Based on the observations from our analysis, we then propose CEPD, a unified DNN compression framework that can co-explore the benefits of structured sparsification and tensor decomposition in an efficient way. Empirical experiments demonstrate the promising performance of our proposed solution. Notably, on the CIFAR-10 dataset, CEPD brings 0.72%-0.45% accuracy increase over the baseline ResNet-56 and MobileNetV2 models, respectively, and meanwhile, the computational costs are reduced by 43.0%-44.2%, respectively. On the ImageNet dataset, our approach can enable 0.10%-1.39% accuracy increase over the baseline ResNet-18 and ResNet-50 models with 59.4%-54.6% fewer parameters, respectively.
更多查看译文
关键词
Model compression,sparsification,tensor decomposition
AI 理解论文
溯源树
样例
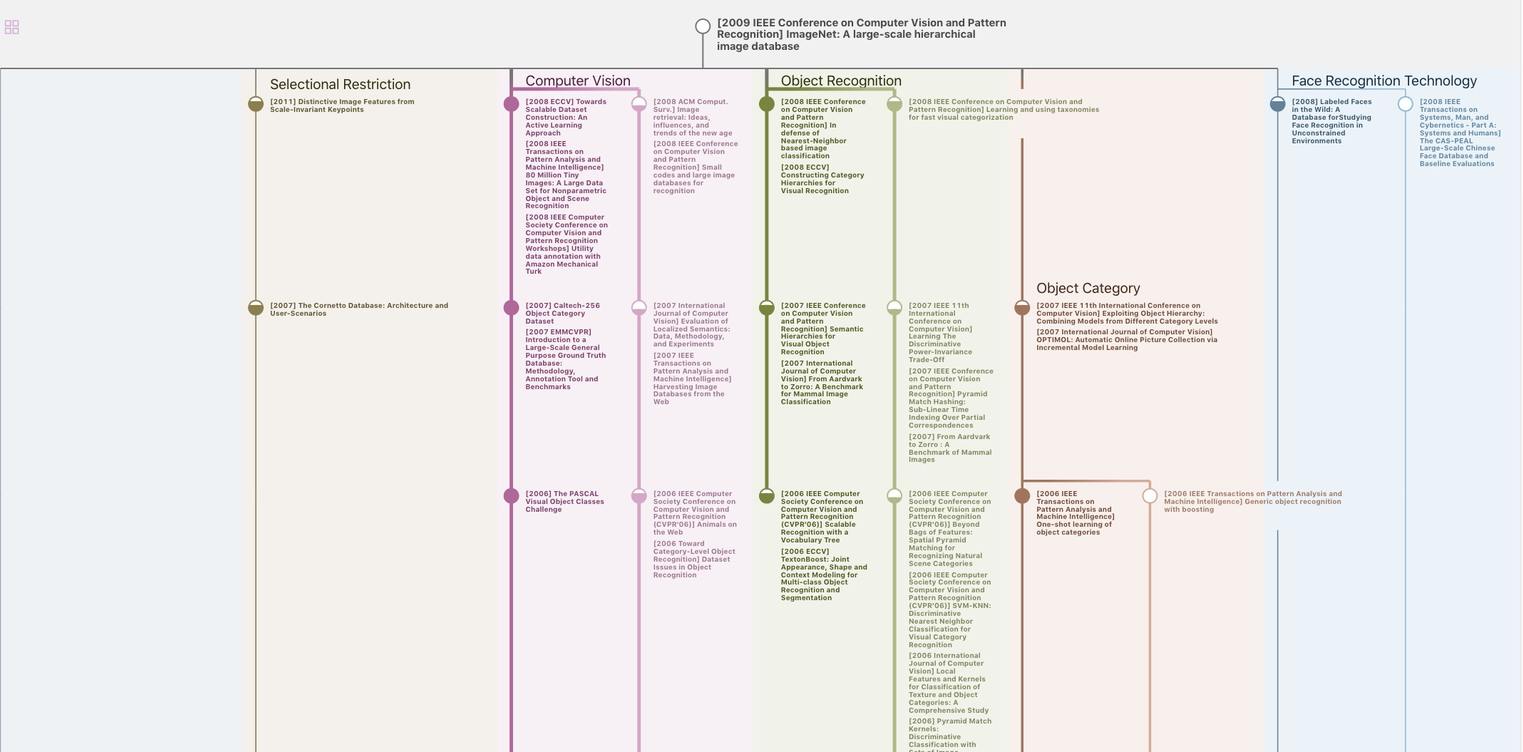
生成溯源树,研究论文发展脉络
Chat Paper
正在生成论文摘要