Benefits from Different Modes of Slow and Deep Breathing on Vagal Modulation
IEEE journal of translational engineering in health and medicine(2024)
摘要
Slow and deep breathing (SDB) is a relaxation technique that can increase vagal activity. Respiratory sinus arrhythmia (RSA) serves as an index of vagal function usually quantified by the high-frequency power of heart rate variability (HRV). However, the low breathing rate during SDB results in deviations when estimating RSA by HRV. Besides, the impact of the inspiration-expiration (I: E) ratio and guidelines ways (fixed breathing rate or intelligent guidance) on SDB is not yet clear. In our study, 30 healthy people (mean age = 26.5 years, 17 females) participated in three SDB modes, including 6 breaths per minute (bpm) with an I:E ratio of 1:1/ 1:2, and intelligent guidance mode (I:E ratio of 1:2 with guiding to gradually lower breathing rate to 6 bpm). Parameters derived from HRV, multimodal coupling analysis (MMCA), Poincar & eacute; plot, and detrended fluctuation analysis were introduced to examine the effects of SDB exercises. Besides, multiple machine learning methods were applied to classify breathing patterns (spontaneous breathing vs. SDB) after feature selection by max-relevance and min-redundancy. All vagal-activity markers, especially MMCA-derived RSA, statistically increased during SDB. Among all SDB modes, breathing at 6 bpm with a 1:1 I:E ratio activated the vagal function the most statistically, while the intelligent guidance mode had more indicators that still significantly increased after training, including SDRR and MMCA-derived RSA, etc. About the classification of breathing patterns, the Naive Bayes classifier has the highest accuracy (92.2%) with input features including LFn, CPercent, pNN50, $\alpha 2$ , SDRatio, $\alpha 1$ , and LF. Our study proposed a system that can be applied to medical devices for automatic SDB identification and real-time feedback on the training effect. We demonstrated that breathing at 6 bpm with an I:E ratio of 1:1 performed best during the training phase, while intelligent guidance mode had a more long-lasting effect.
更多查看译文
关键词
Heart rate variability,Training,Monitoring,Baroreflex,Biomedical monitoring,Resonant frequency,Resonance,Slow deep breathing,heart rate variability (HRV),inspiration-expiration ratio,multimodal coupling analysis (MMCA),detrended fluctuation analysis (DFA)
AI 理解论文
溯源树
样例
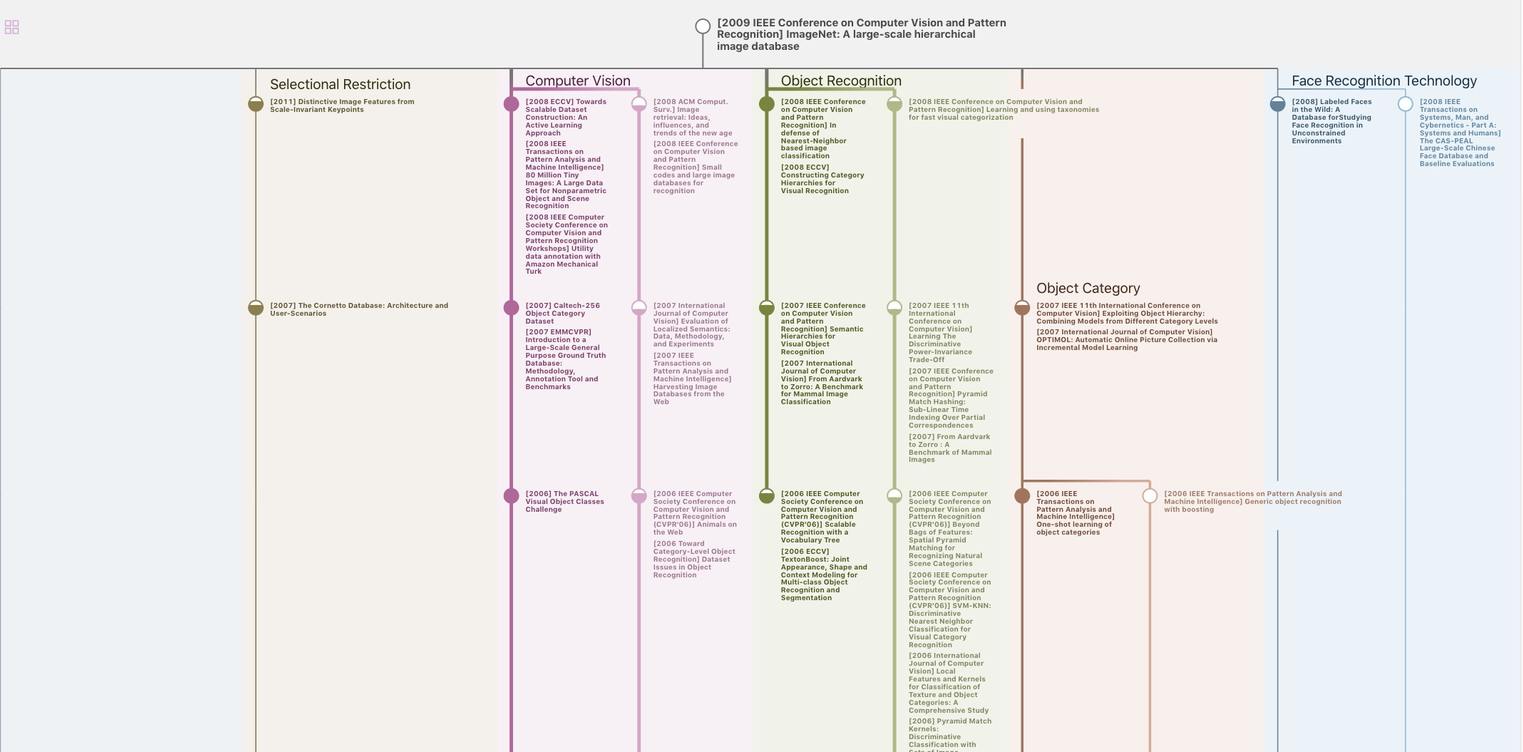
生成溯源树,研究论文发展脉络
Chat Paper
正在生成论文摘要