Machine Learning Assisted Designing of Conjugated Organic Chromophores, Light Absorption, and Emission Behavior Prediction
SPECTROCHIMICA ACTA PART A-MOLECULAR AND BIOMOLECULAR SPECTROSCOPY(2024)
Key words
Machine learning,Conjugated organic chromophores,Light absorption,Light emission,Synthetic accessibility
AI Read Science
Must-Reading Tree
Example
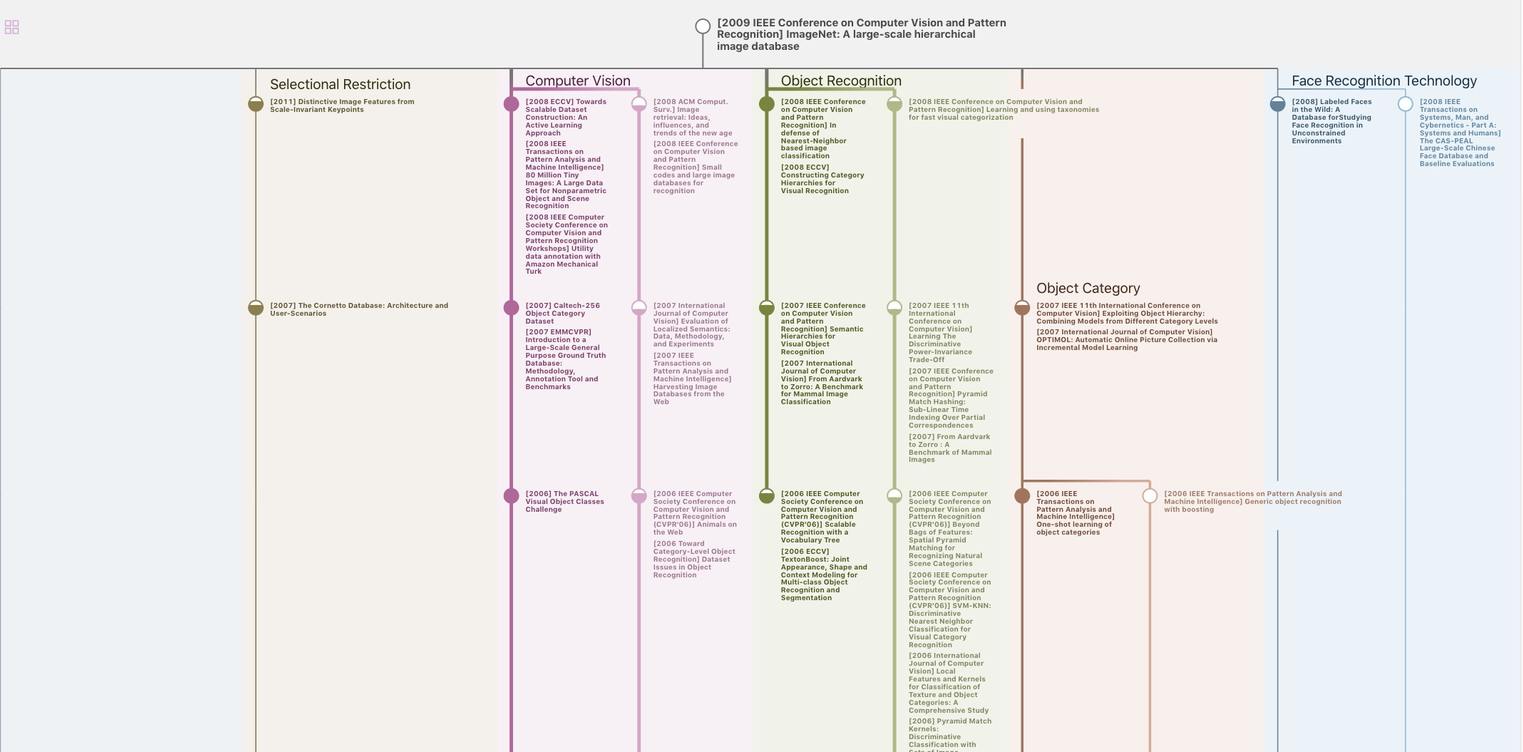
Generate MRT to find the research sequence of this paper
Chat Paper
Summary is being generated by the instructions you defined