DPG-DT: Differentially Private Generative Digital Twin for Imbalanced Learning in Industrial IoT
2023 19TH INTERNATIONAL CONFERENCE ON MOBILITY, SENSING AND NETWORKING, MSN 2023(2023)
关键词
Generative Model,Industrial Internet of Things,Differential Privacy,Digital Twin,Energy-based Model
AI 理解论文
溯源树
样例
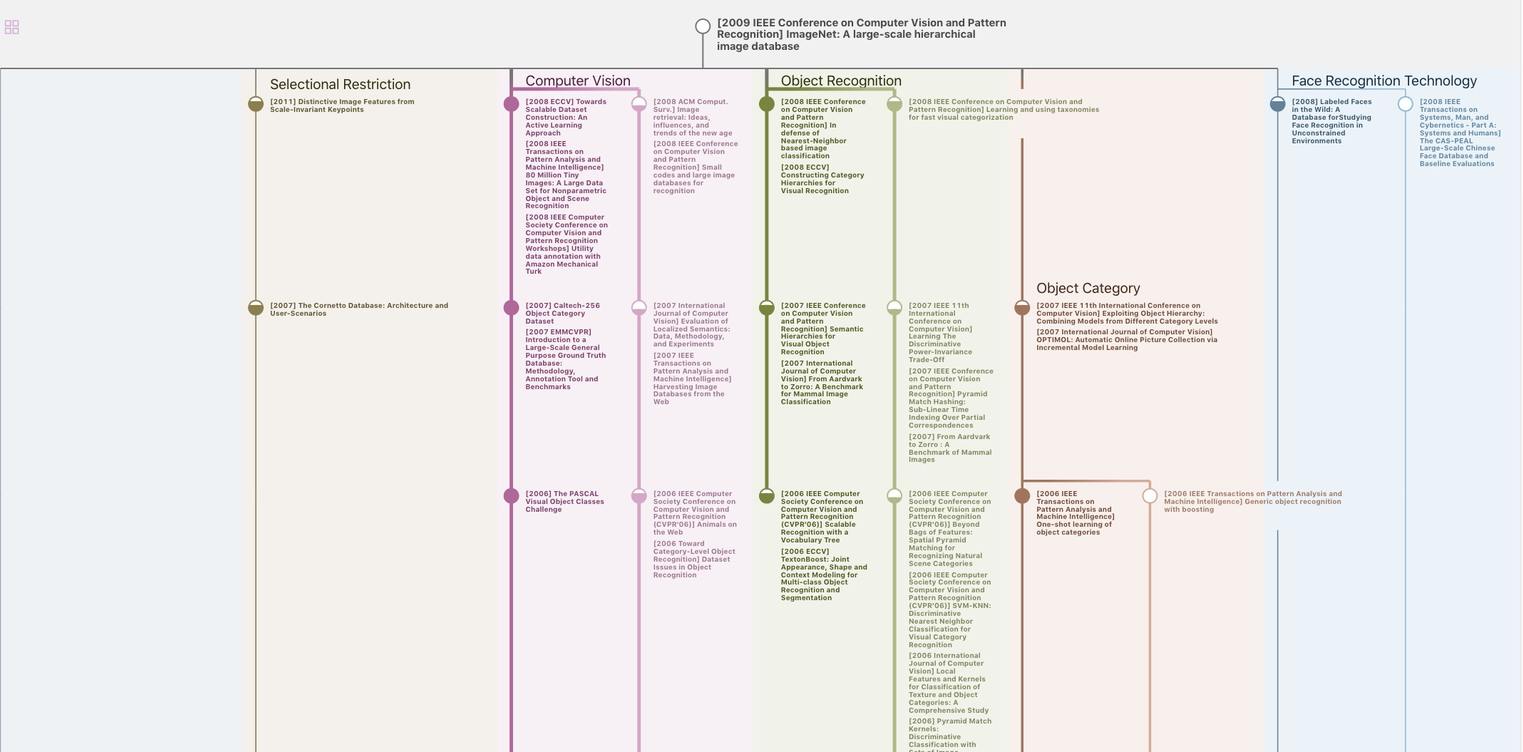
生成溯源树,研究论文发展脉络
Chat Paper
正在生成论文摘要