Input-Adaptation Approach for Human Activity Recognition
17TH ACM INTERNATIONAL CONFERENCE ON PERVASIVE TECHNOLOGIES RELATED TO ASSISTIVE ENVIRONMENTS, PETRA 2024(2024)
摘要
A methodology for Human Activity Recognition using Deep Neural Networks (DNNs) is presented in this paper. The proposed approach introduces a model-driven input adaptation technique that involves the conversion of binary sensor data collected from ambient sensing devices into an image map grid with a sensor activation sequence. The image map is then transformed into a video stream to capture both spatial and temporal features of the data. The generated streams are subsequently utilised for activity recognition. The experimental results demonstrate the effectiveness of the proposed methodology in achieving remarkable performance and the potential of utilising the proposed methodology for data adaptation in sensor fusion. The overall accuracy achieved by the HAR classifier is 89%, with an average F1-Score of 85% on the CASAS dataset. These results are on par with the performance achieved by existing state-of-the-art performance on the same dataset using only the binary sensor data. The findings of this study contribute to advancing the field of HAR and provide valuable insights for developing more efficient and accurate algorithms with heterogeneous sensors.
更多查看译文
关键词
Activities of Daily Living,Human Activity Recognition,Deep Neural Network,Input-Adaptation
AI 理解论文
溯源树
样例
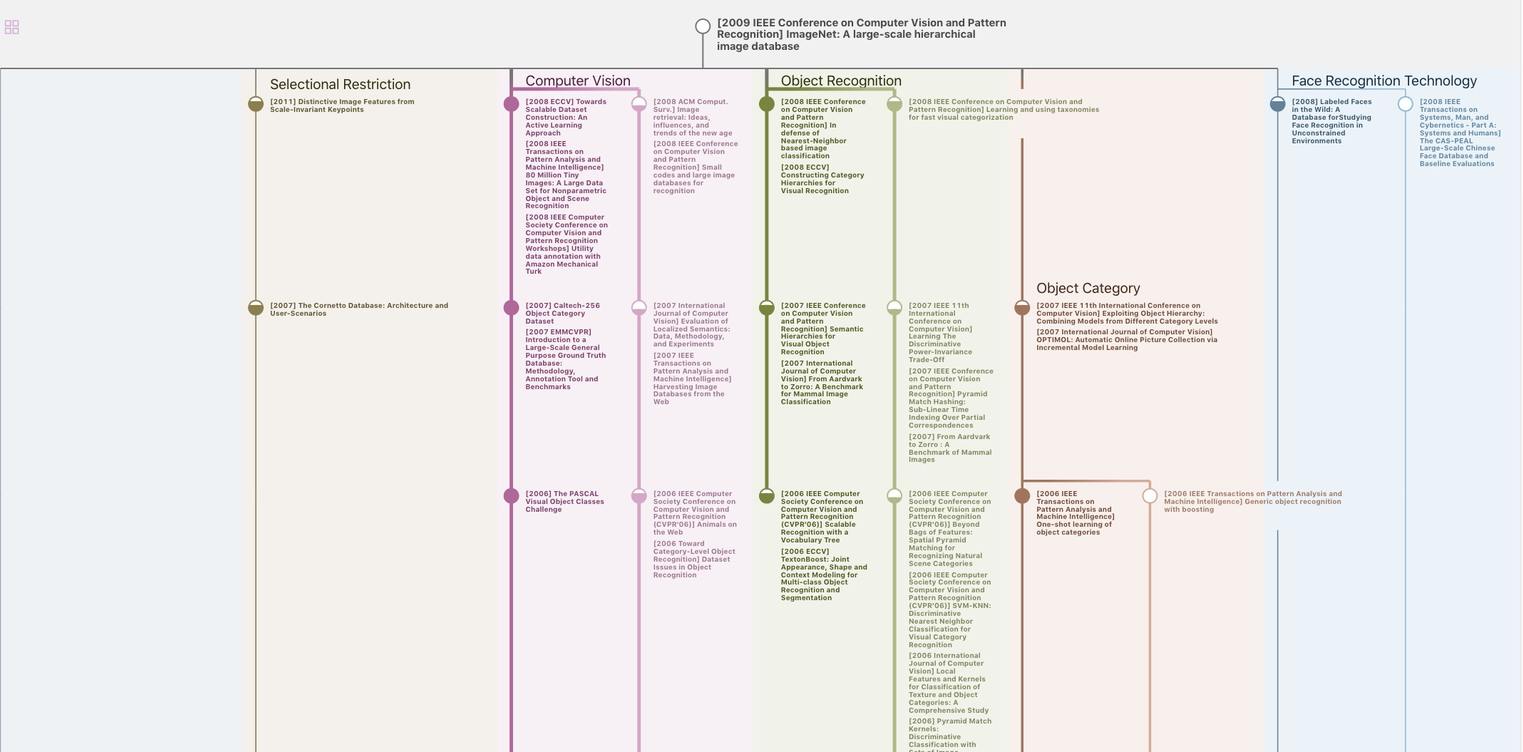
生成溯源树,研究论文发展脉络
Chat Paper
正在生成论文摘要