Automatic Extraction and Cluster Analysis of Natural Disaster Metadata Based on the Unified Metadata Framework
ISPRS international journal of geo-information(2024)
摘要
The development of information technology has led to massive, multidimensional, and heterogeneously sourced disaster data. However, there’s currently no universal metadata standard for managing natural disasters. Common pre-training models for information extraction requiring extensive training data show somewhat limited effectiveness, with limited annotated resources. This study establishes a unified natural disaster metadata standard, utilizes self-trained universal information extraction (UIE) models and Python libraries to extract metadata stored in both structured and unstructured forms, and analyzes the results using the Word2vec-Kmeans cluster algorithm. The results show that (1) the self-trained UIE model, with a learning rate of 3 × 10−4 and a batch_size of 32, significantly improves extraction results for various natural disasters by over 50%. Our optimized UIE model outperforms many other extraction methods in terms of precision, recall, and F1 scores. (2) The quality assessments of consistency, completeness, and accuracy for ten tables all exceed 0.80, with variances between the three dimensions being 0.04, 0.03, and 0.05. The overall evaluation of data items of tables also exceeds 0.80, consistent with the results at the table level. The metadata model framework constructed in this study demonstrates high-quality stability. (3) Taking the flood dataset as an example, clustering reveals five main themes with high similarity within clusters, and the differences between clusters are deemed significant relative to the differences within clusters at a significance level of 0.01. Overall, this experiment supports effective sharing of disaster data resources and enhances natural disaster emergency response efficiency.
更多查看译文
关键词
metadata extraction,UIE,natural disaster,Word2vec-Kmeans clustering
AI 理解论文
溯源树
样例
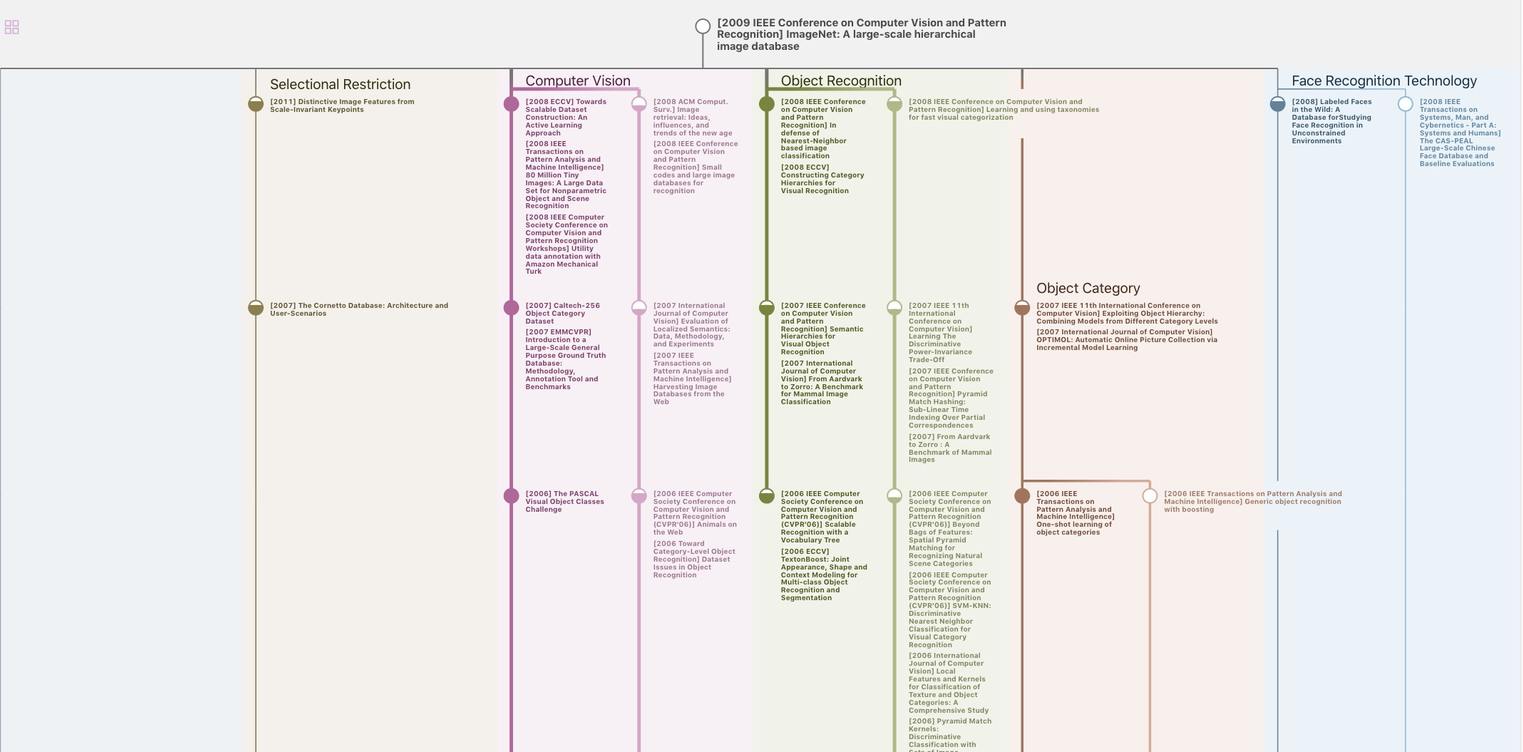
生成溯源树,研究论文发展脉络
Chat Paper
正在生成论文摘要