Unlocking Telemetry Potential: Self-Supervised Learning for Continuous Clinical Electrocardiogram Monitoring
arxiv(2024)
Abstract
Machine learning (ML) applied to routine patient monitoring within intensive care units (ICUs) has the potential to improve care by providing clinicians with novel insights into each patient's health and expected response to interventions. This paper applies deep learning to a large volume of unlabeled electrocardiogram (ECG) telemetry signals, which are commonly used for continuous patient monitoring in hospitals but have important differences from the standard, single time-point 12-lead ECG used in many prior machine learning studies. We applied self-supervised learning to pretrain a spectrum of deep networks on approximately 147,000 hours of ECG telemetry data. Our approach leverages this dataset to train models that significantly improve performance on four distinct downstream tasks compared with direct supervised learning using labeled data. These pretrained models enable medically useful predictions and estimates in smaller patient cohorts that are typically limited by the scarcity of labels. Notably, we demonstrate that our pretrained networks can continuously annotate ECG telemetry signals, thereby providing monitoring capabilities that are often unavailable due to the requirement for specialized expertise and time-consuming professional annotations.
MoreTranslated text
AI Read Science
Must-Reading Tree
Example
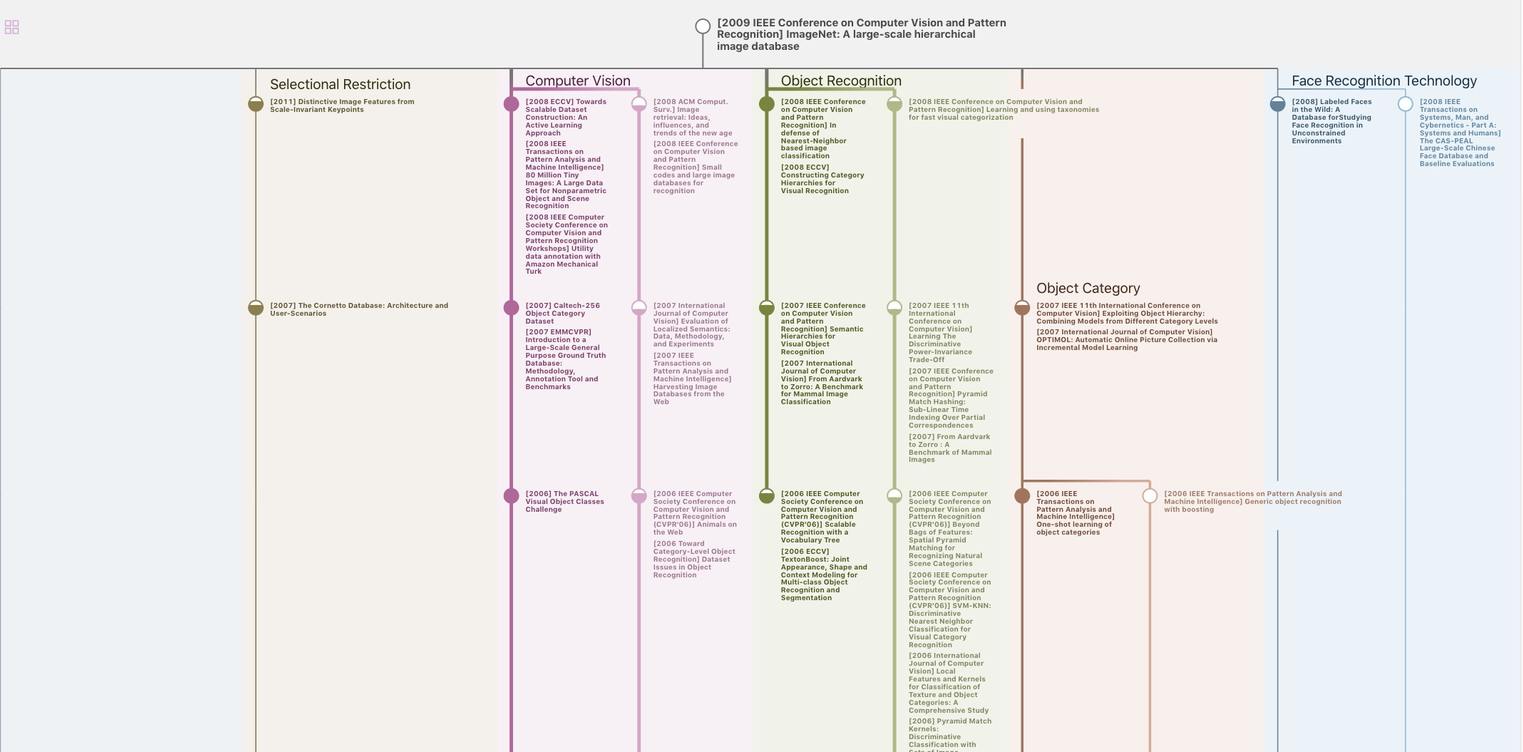
Generate MRT to find the research sequence of this paper
Chat Paper
Summary is being generated by the instructions you defined